书目名称 | Explainable and Interpretable Models in Computer Vision and Machine Learning |
编辑 | Hugo Jair Escalante,Sergio Escalera,Marcel‘van Ger |
视频video | |
概述 | Presents a snapshot of explainable and interpretable models in the context of computer vision and machine learning.Covers fundamental topics to serve as a reference for newcomers to the field.Offers s |
丛书名称 | The Springer Series on Challenges in Machine Learning |
图书封面 | 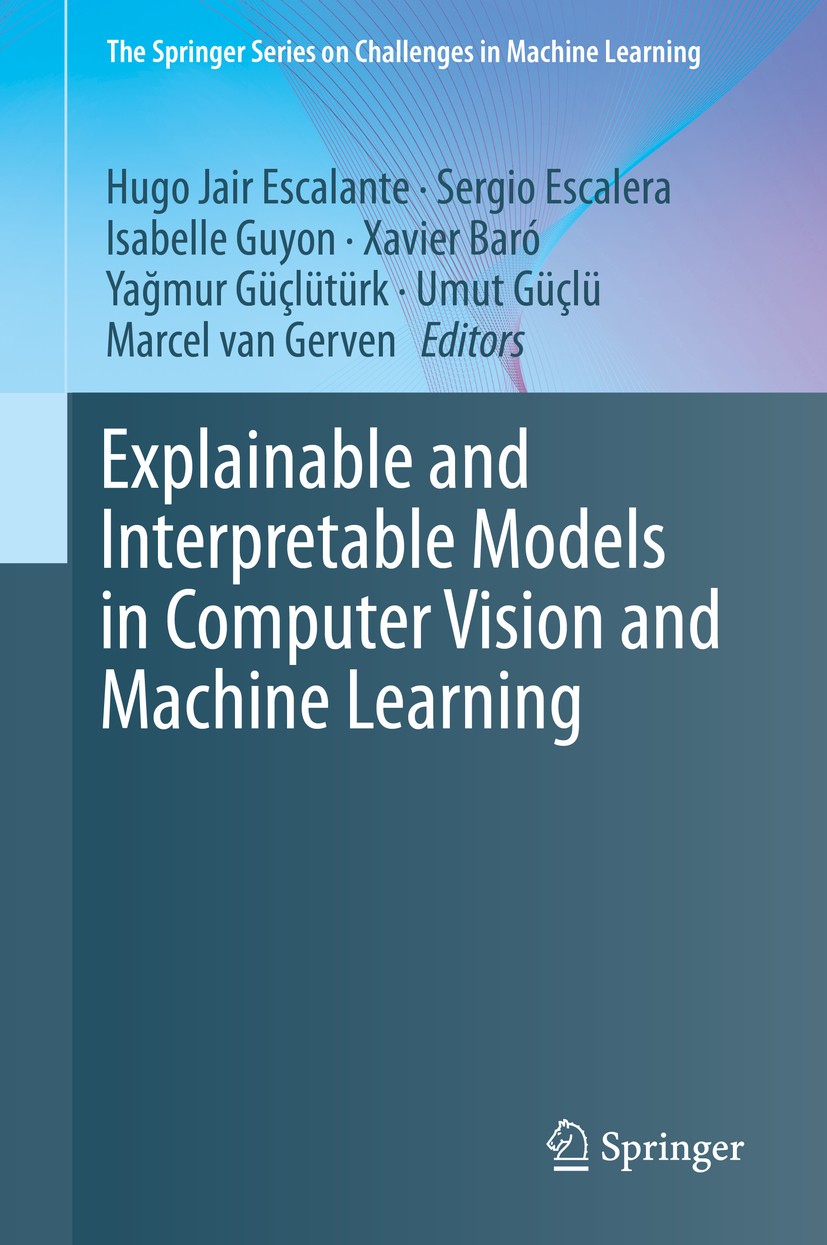 |
描述 | .This book compiles leading research on the development of explainable and interpretable machine learning methods in the context of computer vision and machine learning...Research progress in computer vision and pattern recognition has led to a variety of modeling techniques with almost human-like performance. Although these models have obtained astounding results, they are limited in their explainability and interpretability: what is the rationale behind the decision made? what in the model structure explains its functioning? Hence, while good performance is a critical required characteristic for learning machines, explainability and interpretability capabilities are needed to take learning machines to the next step to include them in decision support systems involving human supervision. .. This book, written by leading international researchers, addresses key topics of explainability and interpretability, including the following:.. ..· Evaluation and Generalization in Interpretable Machine Learning..· Explanation Methods in Deep Learning..· Learning Functional Causal Models with Generative Neural Networks..· Learning Interpreatable Rules for Mult |
出版日期 | Book 2018 |
关键词 | Explainable models in computer vision; Explainable learning machines; Interpretable models; Explaining |
版次 | 1 |
doi | https://doi.org/10.1007/978-3-319-98131-4 |
isbn_ebook | 978-3-319-98131-4Series ISSN 2520-131X Series E-ISSN 2520-1328 |
issn_series | 2520-131X |
copyright | Springer Nature Switzerland AG 2018 |