书目名称 | Metric Learning | 编辑 | Aurélien Bellet,Amaury Habrard,Marc Sebban | 视频video | | 丛书名称 | Synthesis Lectures on Artificial Intelligence and Machine Learning | 图书封面 | 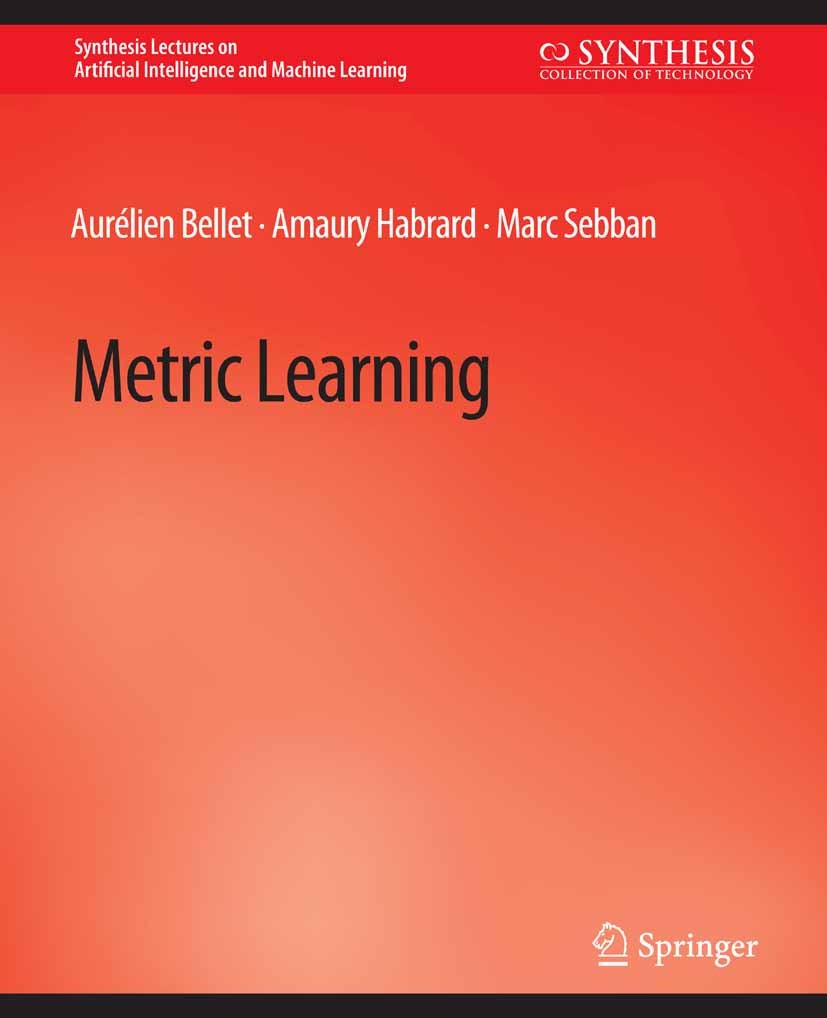 | 描述 | Similarity between objects plays an important role in both human cognitive processes and artificial systems for recognition and categorization. How to appropriately measure such similarities for a given task is crucial to the performance of many machine learning, pattern recognition and data mining methods. This book is devoted to metric learning, a set of techniques to automatically learn similarity and distance functions from data that has attracted a lot of interest in machine learning and related fields in the past ten years. In this book, we provide a thorough review of the metric learning literature that covers algorithms, theory and applications for both numerical and structured data. We first introduce relevant definitions and classic metric functions, as well as examples of their use in machine learning and data mining. We then review a wide range of metric learning algorithms, starting with the simple setting of linear distance and similarity learning. We show how one may scale-up these methods to very large amounts of training data. To go beyond the linear case, we discuss methods that learn nonlinear metrics or multiple linear metrics throughout the feature space, and r | 出版日期 | Book 2015 | 版次 | 1 | doi | https://doi.org/10.1007/978-3-031-01572-4 | isbn_softcover | 978-3-031-00444-5 | isbn_ebook | 978-3-031-01572-4Series ISSN 1939-4608 Series E-ISSN 1939-4616 | issn_series | 1939-4608 | copyright | Springer Nature Switzerland AG 2015 |
The information of publication is updating
|
|