书目名称 | Machine Learning and Knowledge Discovery in Databases |
副标题 | European Conference, |
编辑 | Massih-Reza Amini,Stéphane Canu,Grigorios Tsoumaka |
视频video | |
丛书名称 | Lecture Notes in Computer Science |
图书封面 | 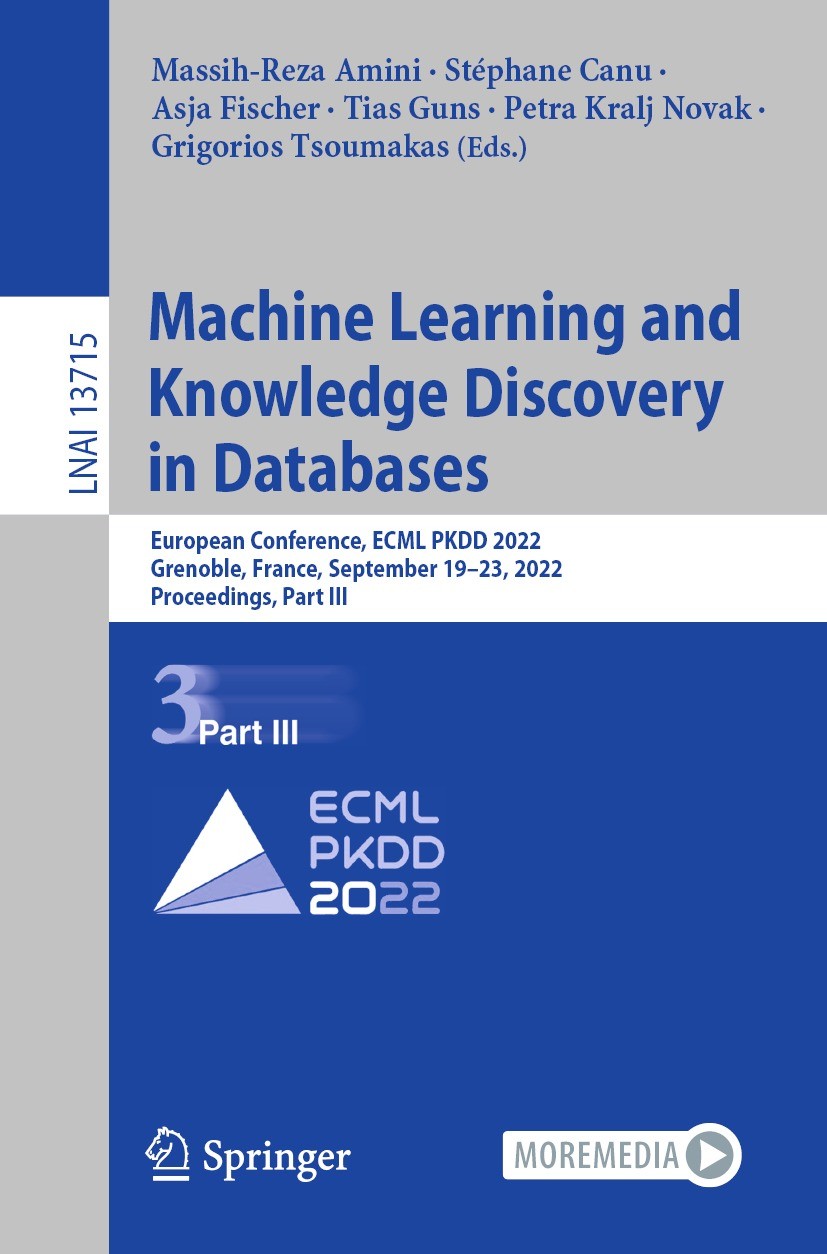 |
描述 | The multi-volume set LNAI 13713 until 13718 constitutes the refereed proceedings of the European Conference on Machine Learning and Knowledge Discovery in Databases, ECML PKDD 2022, which took place in Grenoble, France, in September 2022..The 236 full papers presented in these proceedings were carefully reviewed and selected from a total of 1060 submissions. In addition, the proceedings include 17 Demo Track contributions...The volumes are organized in topical sections as follows:..Part I:. Clustering and dimensionality reduction; anomaly detection; interpretability and explainability; ranking and recommender systems; transfer and multitask learning; ..Part II: .Networks and graphs; knowledge graphs; social network analysis; graph neural networks; natural language processing and text mining; conversational systems; ..Part III: .Deep learning; robust and adversarial machine learning; generative models; computer vision; meta-learning, neural architecture search; ..Part IV:. Reinforcement learning; multi-agent reinforcement learning; bandits and online learning; active and semi-supervised learning; private and federated learning; ...Part V:. Supervised learning; probabilistic inferenc |
出版日期 | Conference proceedings 2023 |
关键词 | artificial intelligence; computer networks; computer vision; deep learning; education; engineering; image |
版次 | 1 |
doi | https://doi.org/10.1007/978-3-031-26409-2 |
isbn_softcover | 978-3-031-26408-5 |
isbn_ebook | 978-3-031-26409-2Series ISSN 0302-9743 Series E-ISSN 1611-3349 |
issn_series | 0302-9743 |
copyright | The Editor(s) (if applicable) and The Author(s), under exclusive license to Springer Nature Switzerl |