书目名称 | Machine Learning and Deep Learning in Computational Toxicology | 编辑 | Huixiao Hong | 视频video | | 概述 | Covers comprehensive view of the machine learning and deep learning algorithms, methods, and software tools.Provides many practical applications of machine learning and deep learning techniques in pre | 丛书名称 | Computational Methods in Engineering & the Sciences | 图书封面 | 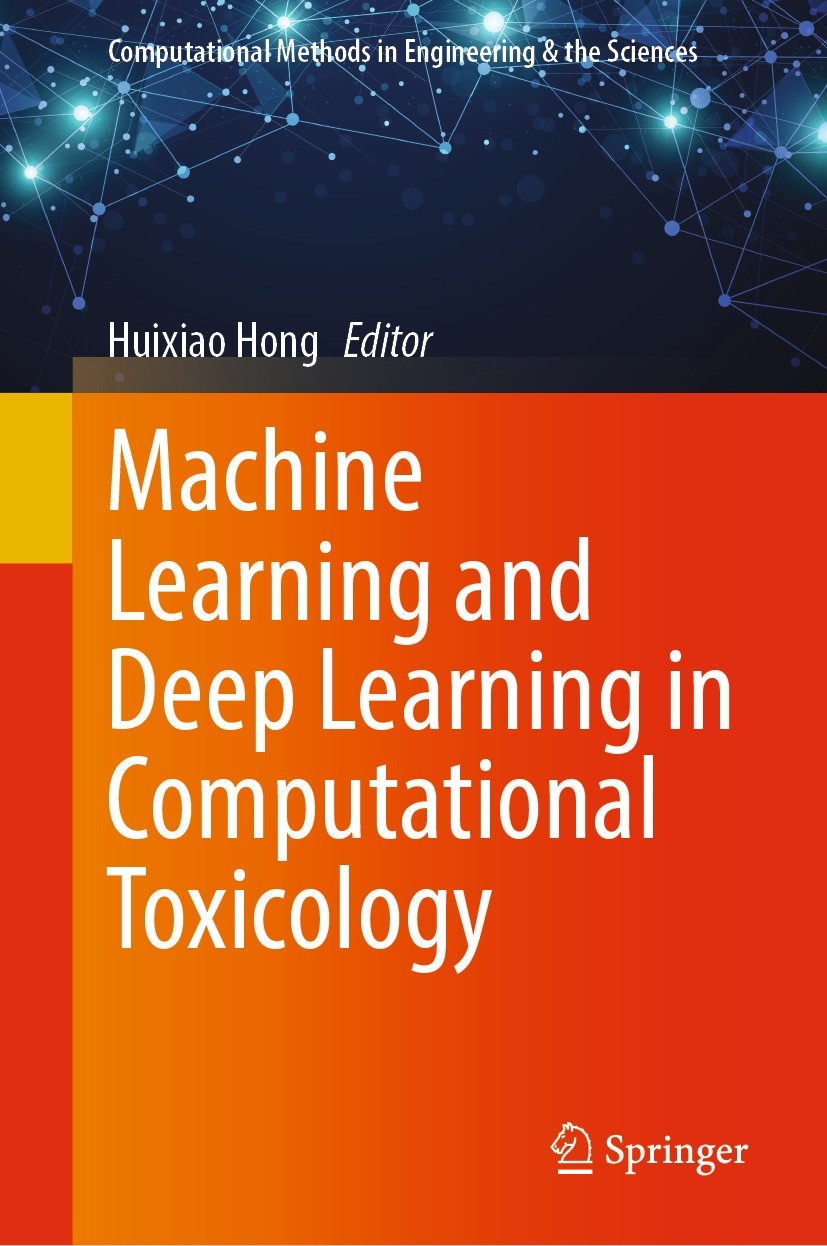 | 描述 | This book is a collection of machine learning and deep learning algorithms, methods, architectures, and software tools that have been developed and widely applied in predictive toxicology. It compiles a set of recent applications using state-of-the-art machine learning and deep learning techniques in analysis of a variety of toxicological endpoint data. The contents illustrate those machine learning and deep learning algorithms, methods, and software tools and summarise the applications of machine learning and deep learning in predictive toxicology with informative text, figures, and tables that are contributed by the first tier of experts. One of the major features is the case studies of applications of machine learning and deep learning in toxicological research that serve as examples for readers to learn how to apply machine learning and deep learning techniques in predictive toxicology. This book is expected to provide a reference for practical applications of machine learning anddeep learning in toxicological research. It is a useful guide for toxicologists, chemists, drug discovery and development researchers, regulatory scientists, government reviewers, and graduate students | 出版日期 | Book 2023 | 关键词 | Machine Learning; Deep Learning; Toxicology; Model; Prediction; Algorithm | 版次 | 1 | doi | https://doi.org/10.1007/978-3-031-20730-3 | isbn_softcover | 978-3-031-20732-7 | isbn_ebook | 978-3-031-20730-3Series ISSN 2662-4869 Series E-ISSN 2662-4877 | issn_series | 2662-4869 | copyright | This is a U.S. government work and not under copyright protection in the U.S.; foreign copyright pro |
The information of publication is updating
|
|