书目名称 | Graphs in Biomedical Image Analysis, and Overlapped Cell on Tissue Dataset for Histopathology | 编辑 | Seyed-Ahmad Ahmadi,Sérgio Pereira | 视频video | | 丛书名称 | Lecture Notes in Computer Science | 图书封面 | 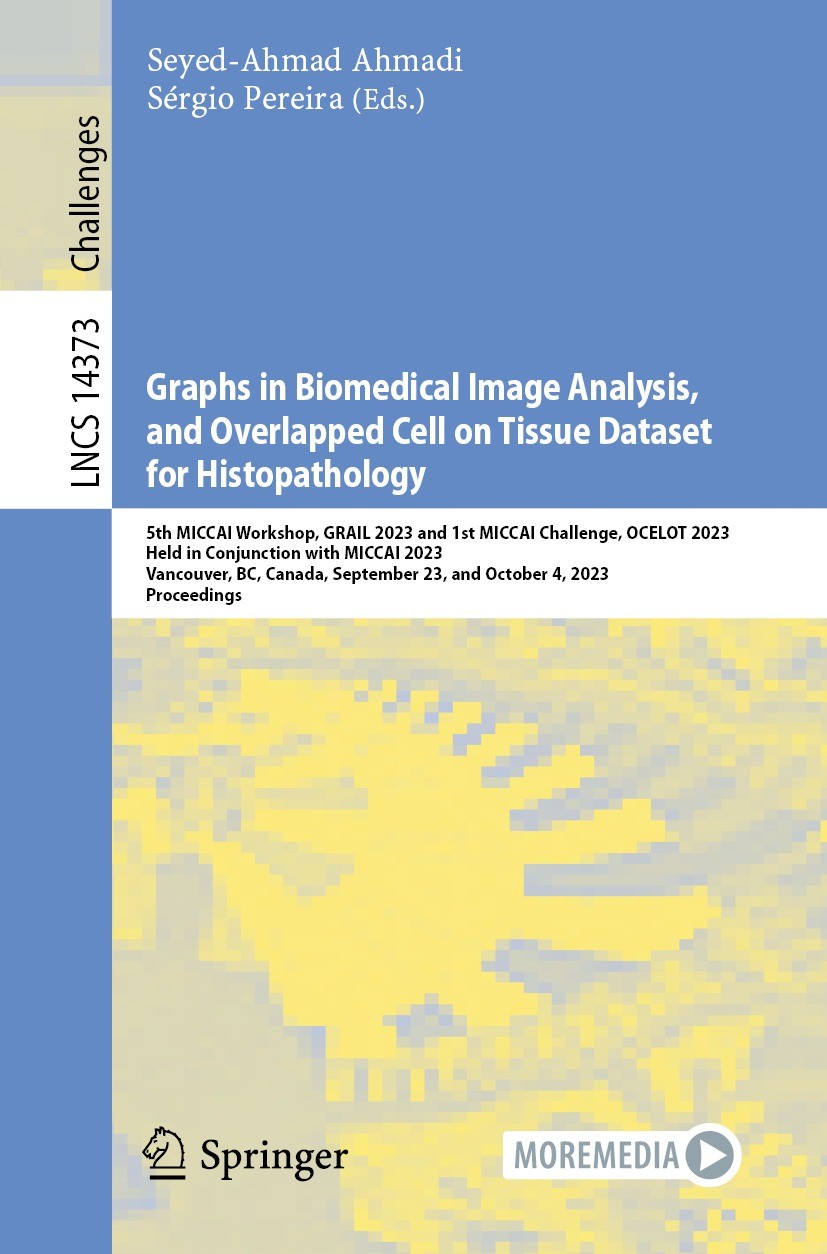 | 出版日期 | Conference proceedings 2024 | 版次 | 1 | doi | https://doi.org/10.1007/978-3-031-55088-1 | isbn_softcover | 978-3-031-55087-4 | isbn_ebook | 978-3-031-55088-1Series ISSN 0302-9743 Series E-ISSN 1611-3349 | issn_series | 0302-9743 |
The information of publication is updating
书目名称Graphs in Biomedical Image Analysis, and Overlapped Cell on Tissue Dataset for Histopathology影响因子(影响力) 
书目名称Graphs in Biomedical Image Analysis, and Overlapped Cell on Tissue Dataset for Histopathology影响因子(影响力)学科排名 
书目名称Graphs in Biomedical Image Analysis, and Overlapped Cell on Tissue Dataset for Histopathology网络公开度 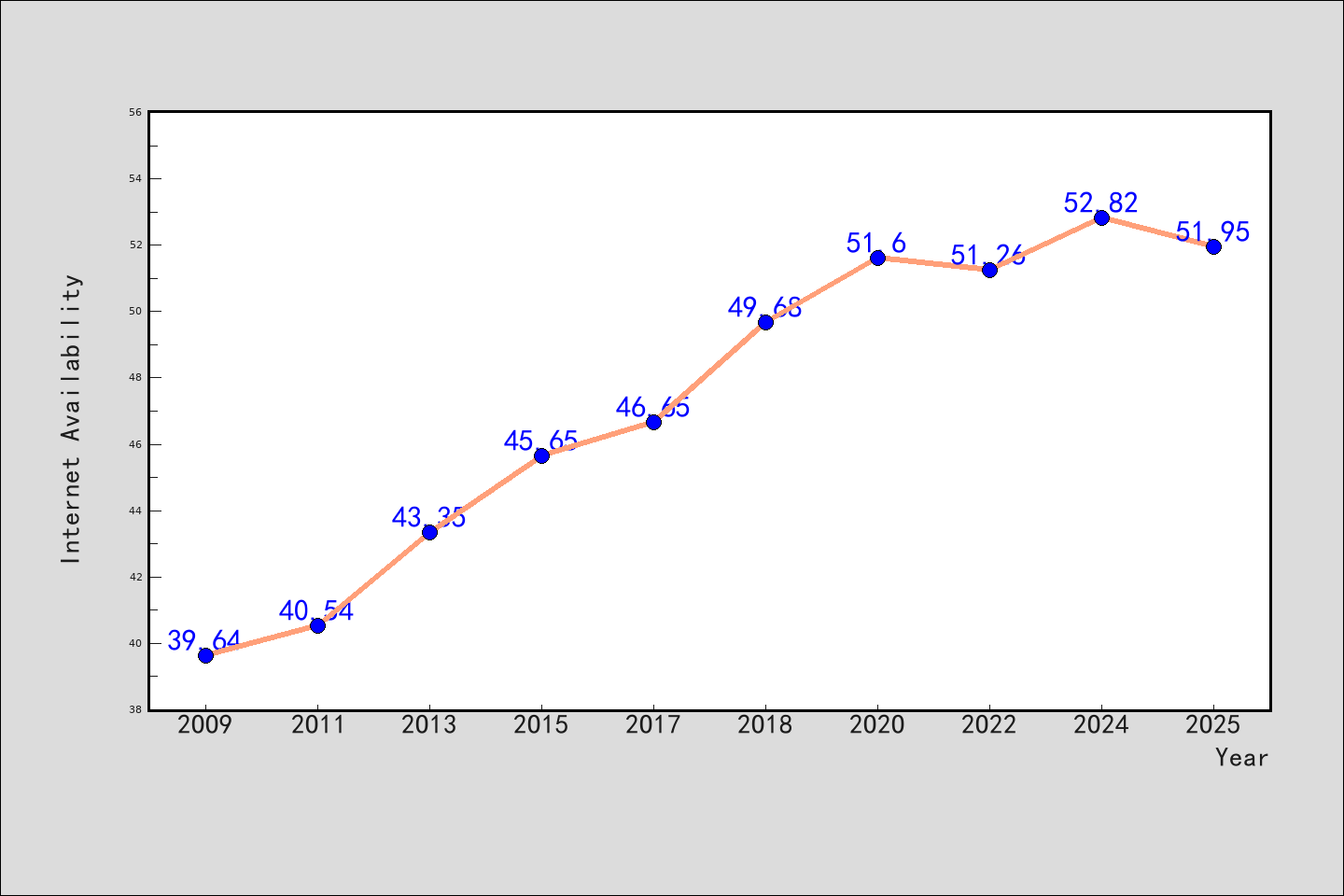
书目名称Graphs in Biomedical Image Analysis, and Overlapped Cell on Tissue Dataset for Histopathology网络公开度学科排名 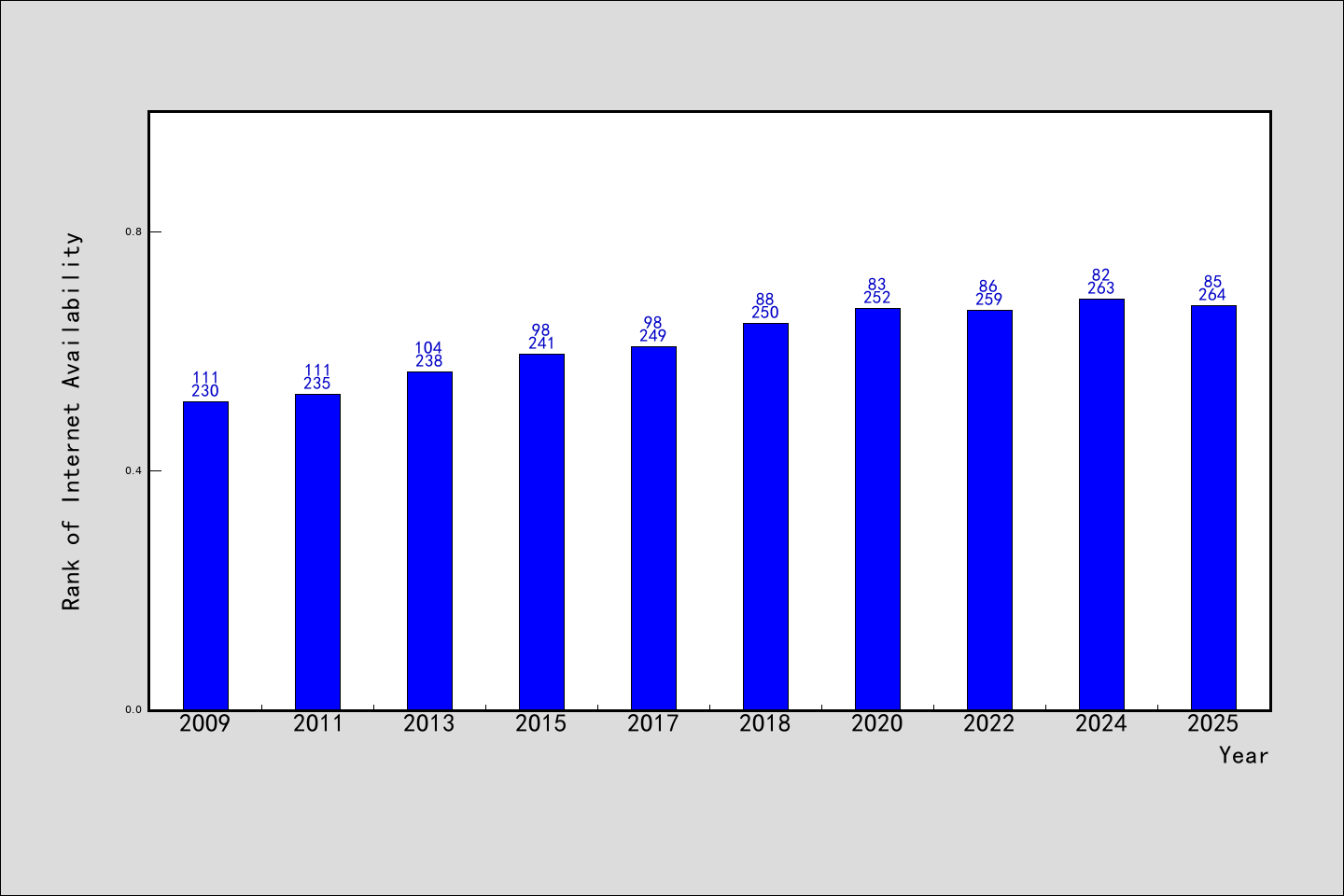
书目名称Graphs in Biomedical Image Analysis, and Overlapped Cell on Tissue Dataset for Histopathology被引频次 
书目名称Graphs in Biomedical Image Analysis, and Overlapped Cell on Tissue Dataset for Histopathology被引频次学科排名 
书目名称Graphs in Biomedical Image Analysis, and Overlapped Cell on Tissue Dataset for Histopathology年度引用 
书目名称Graphs in Biomedical Image Analysis, and Overlapped Cell on Tissue Dataset for Histopathology年度引用学科排名 
书目名称Graphs in Biomedical Image Analysis, and Overlapped Cell on Tissue Dataset for Histopathology读者反馈 
书目名称Graphs in Biomedical Image Analysis, and Overlapped Cell on Tissue Dataset for Histopathology读者反馈学科排名 
|
|
|