书目名称 | Effective Statistical Learning Methods for Actuaries I | 副标题 | GLMs and Extensions | 编辑 | Michel Denuit,Donatien Hainaut,Julien Trufin | 视频video | | 概述 | Features numerous examples and case studies in P&C, Life and Health insurance.Provides a broad and self-contained presentation of insurance data analytics techniques, from classical GLMs to neural net | 丛书名称 | Springer Actuarial | 图书封面 | 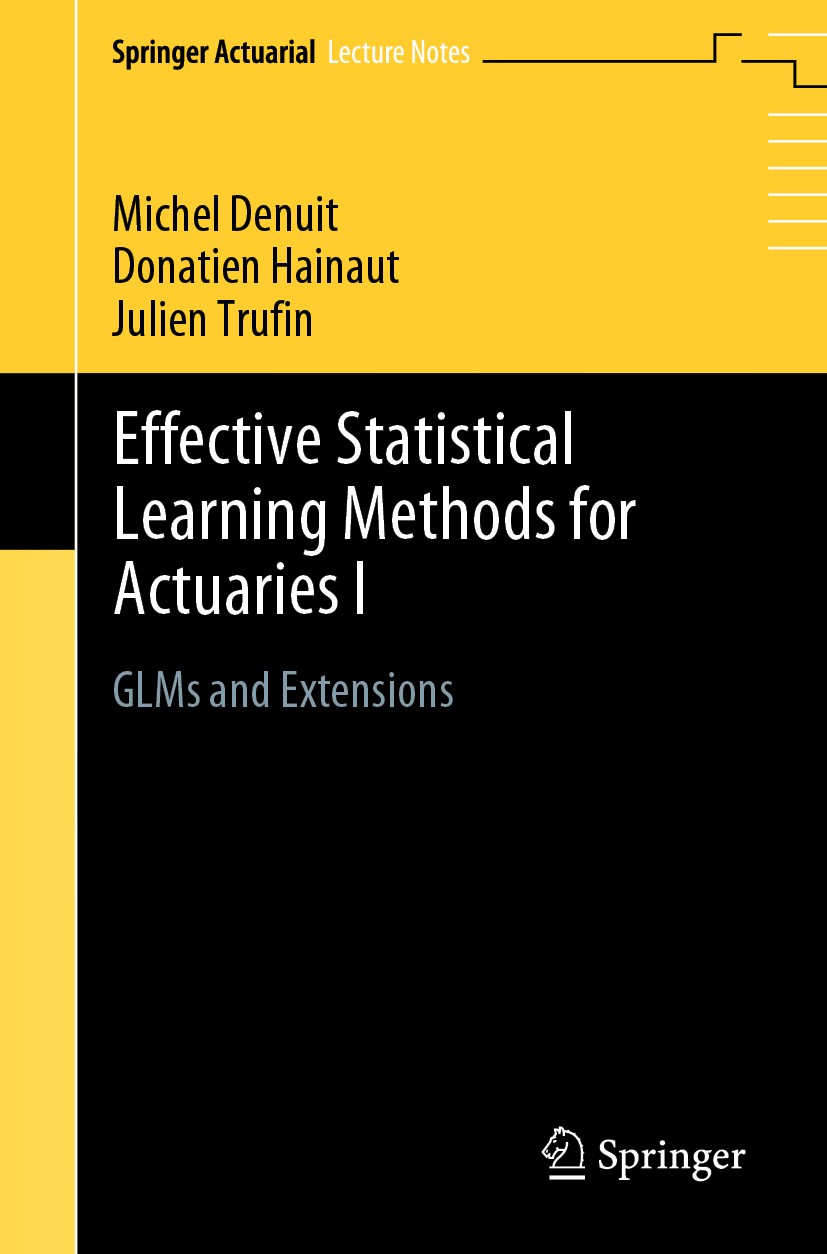 | 描述 | .This book summarizes the state of the art in generalized linear models (GLMs) and their various extensions: GAMs, mixed models and credibility, and some nonlinear variants (GNMs). In order to deal with tail events, analytical tools from Extreme Value Theory are presented. Going beyond mean modeling, it considers volatility modeling (double GLMs) and the general modeling of location, scale and shape parameters (GAMLSS). Actuaries need these advanced analytical tools to turn the massive data sets now at their disposal into opportunities.. .The exposition alternates between methodological aspects and case studies, providing numerical illustrations using the R statistical software. The technical prerequisites are kept at a reasonable level in order to reach a broad readership. ..This is the first of three volumes entitled .Effective Statistical Learning Methods for Actuaries.. Written by actuaries for actuaries, this series offers a comprehensive overview of insurance data analytics with applications to P&C, life and health insurance. Although closely related to the other two volumes, this volume can be read independently.. | 出版日期 | Textbook 2019 | 关键词 | Insurance risk classification; Supervised learning; Exponential dispersion model; Regression analysis; G | 版次 | 1 | doi | https://doi.org/10.1007/978-3-030-25820-7 | isbn_softcover | 978-3-030-25819-1 | isbn_ebook | 978-3-030-25820-7Series ISSN 2523-3262 Series E-ISSN 2523-3270 | issn_series | 2523-3262 | copyright | Springer Nature Switzerland AG 2019 |
The information of publication is updating
|
|