书目名称 | Driving Scientific and Engineering Discoveries Through the Convergence of HPC, Big Data and AI | 副标题 | 17th Smoky Mountains | 编辑 | Jeffrey Nichols,Becky Verastegui,Theresa Ahearn | 视频video | | 丛书名称 | Communications in Computer and Information Science | 图书封面 | 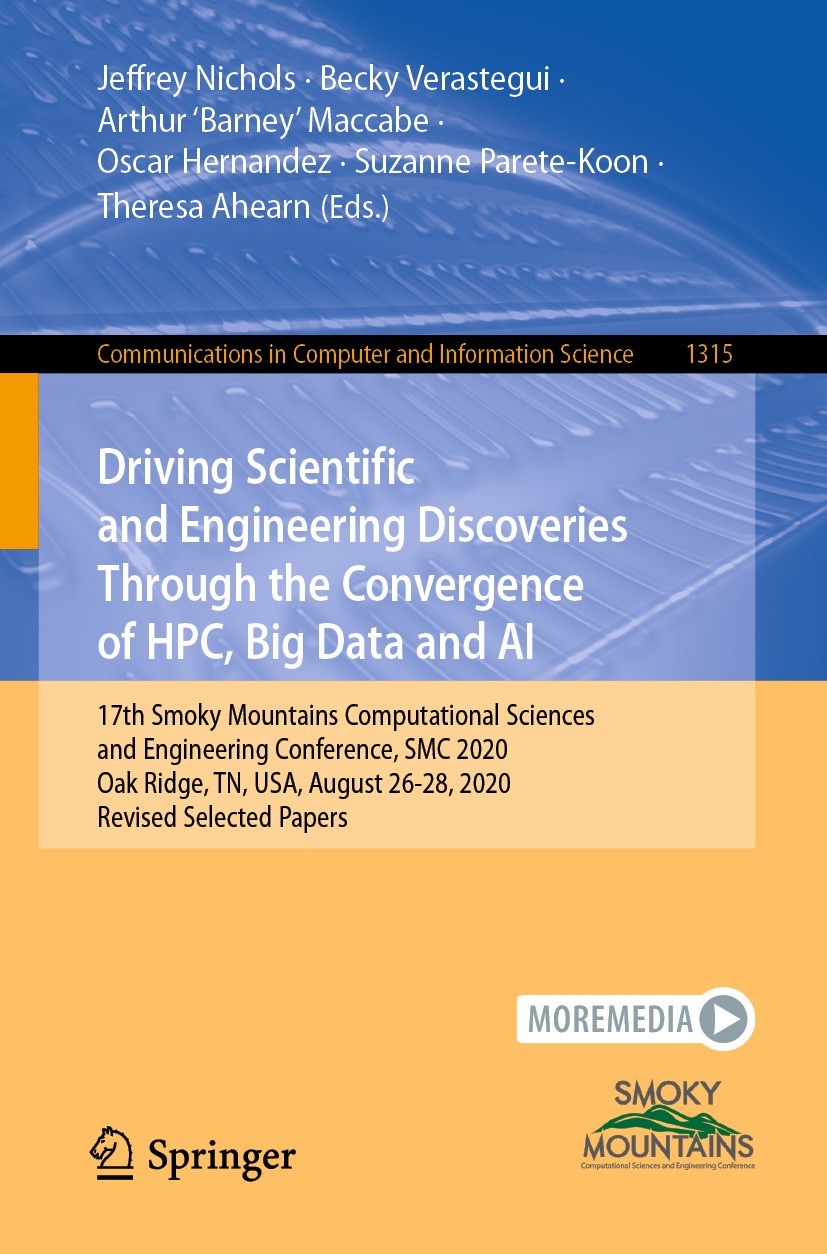 | 描述 | This book constitutes the revised selected papers of the 17th Smoky Mountains Computational Sciences and Engineering Conference, SMC 2020, held in Oak Ridge, TN, USA*, in August 2020..The 36 full papers and 1 short paper presented were carefully reviewed and selected from a total of 94 submissions. The papers are organized in topical sections of computational applications: converged HPC and artificial intelligence; system software: data infrastructure and life cycle; experimental/observational applications: use cases that drive requirements for AI and HPC convergence; deploying computation: on the road to a converged ecosystem; scientific data challenges..*The conference was held virtually due to the COVID-19 pandemic.. | 出版日期 | Conference proceedings 2020 | 关键词 | artificial intelligence; cloud computing; computer hardware; computer networks; computer systems; compute | 版次 | 1 | doi | https://doi.org/10.1007/978-3-030-63393-6 | isbn_softcover | 978-3-030-63392-9 | isbn_ebook | 978-3-030-63393-6Series ISSN 1865-0929 Series E-ISSN 1865-0937 | issn_series | 1865-0929 | copyright | Springer Nature Switzerland AG 2020 |
The information of publication is updating
书目名称Driving Scientific and Engineering Discoveries Through the Convergence of HPC, Big Data and AI影响因子(影响力) 
书目名称Driving Scientific and Engineering Discoveries Through the Convergence of HPC, Big Data and AI影响因子(影响力)学科排名 
书目名称Driving Scientific and Engineering Discoveries Through the Convergence of HPC, Big Data and AI网络公开度 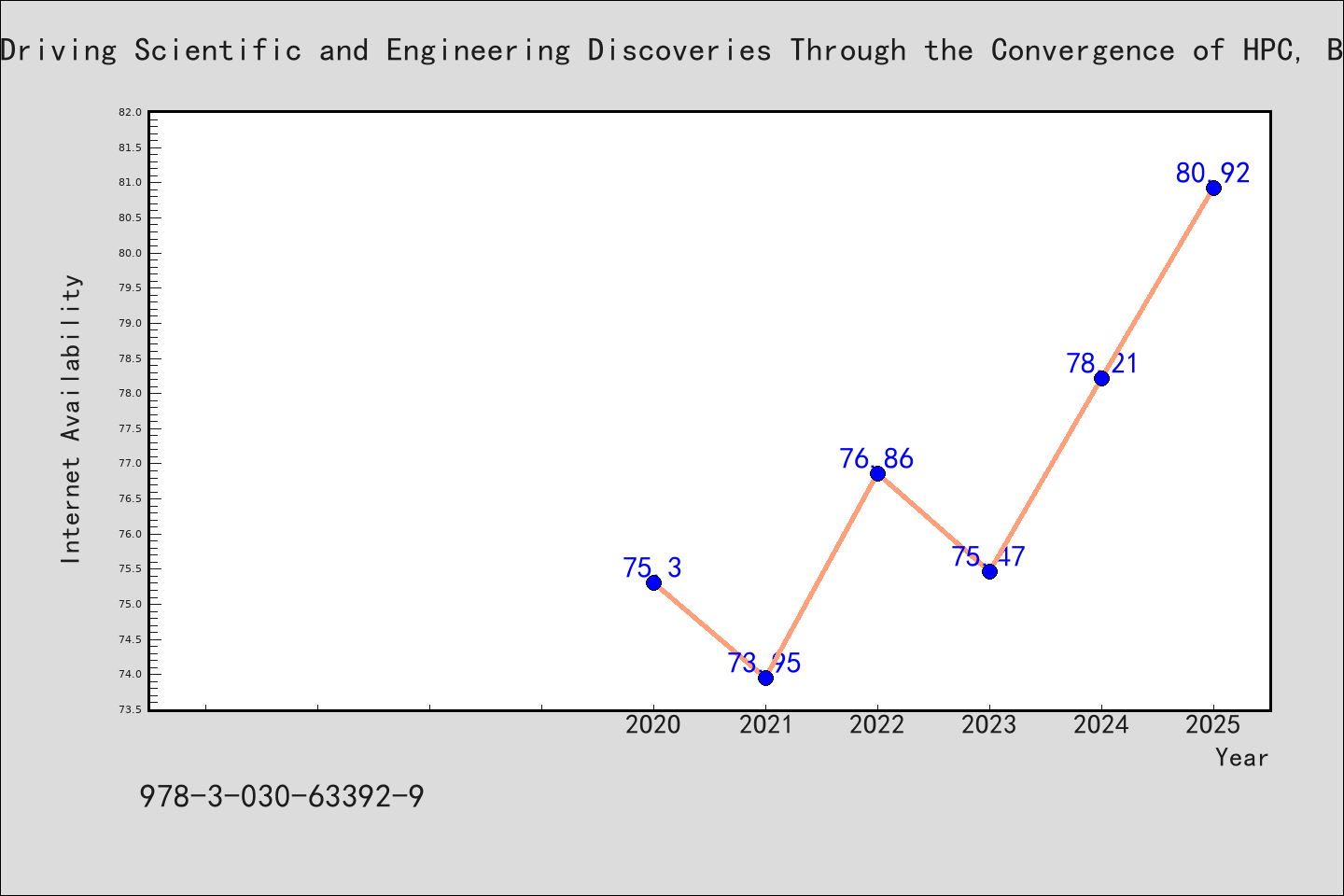
书目名称Driving Scientific and Engineering Discoveries Through the Convergence of HPC, Big Data and AI网络公开度学科排名 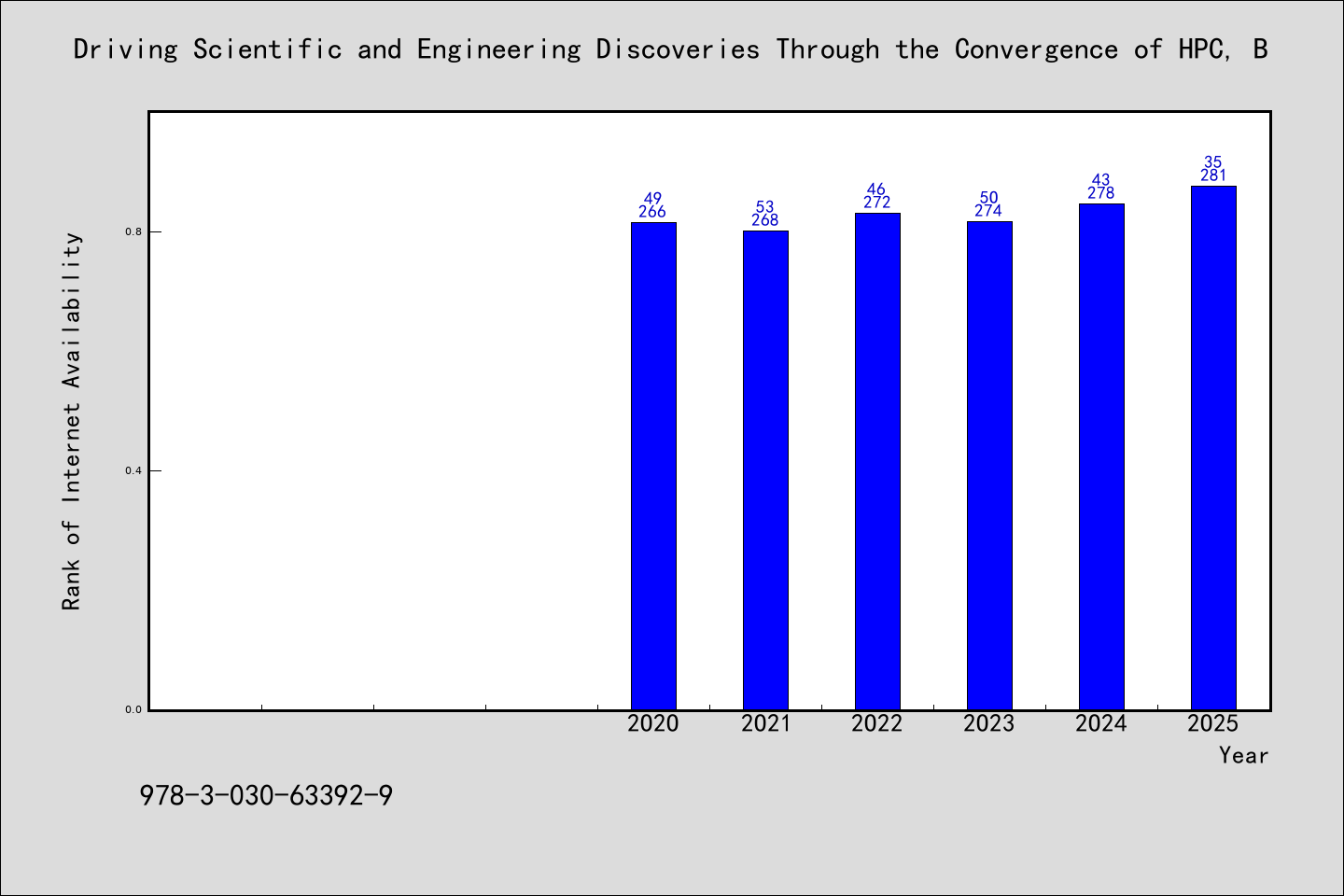
书目名称Driving Scientific and Engineering Discoveries Through the Convergence of HPC, Big Data and AI被引频次 
书目名称Driving Scientific and Engineering Discoveries Through the Convergence of HPC, Big Data and AI被引频次学科排名 
书目名称Driving Scientific and Engineering Discoveries Through the Convergence of HPC, Big Data and AI年度引用 
书目名称Driving Scientific and Engineering Discoveries Through the Convergence of HPC, Big Data and AI年度引用学科排名 
书目名称Driving Scientific and Engineering Discoveries Through the Convergence of HPC, Big Data and AI读者反馈 
书目名称Driving Scientific and Engineering Discoveries Through the Convergence of HPC, Big Data and AI读者反馈学科排名 
|
|
|