书目名称 | Domain Adaptation in Computer Vision Applications | 编辑 | Gabriela Csurka | 视频video | | 概述 | The first book focused on domain adaptation for visual applications.Provides a comprehensive experimental study, highlighting the strengths and weaknesses of popular methods, and introducing new and m | 丛书名称 | Advances in Computer Vision and Pattern Recognition | 图书封面 | 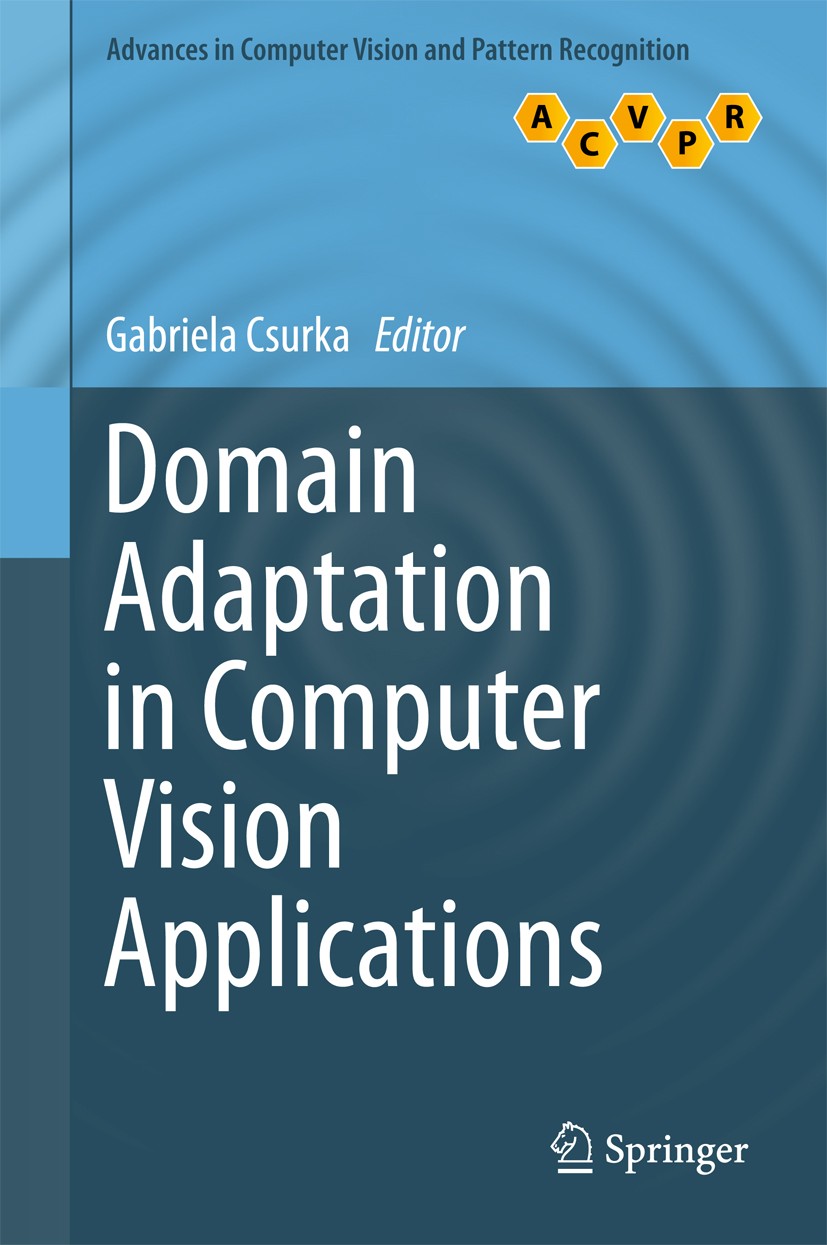 | 描述 | This comprehensive text/reference presents a broad review of diverse domain adaptation (DA) methods for machine learning, with a focus on solutions for visual applications. The book collects together solutions and perspectives proposed by an international selection of pre-eminent experts in the field, addressing not only classical image categorization, but also other computer vision tasks such as detection, segmentation and visual attributes..Topics and features: surveys the complete field of visual DA, including shallow methods designed for homogeneous and heterogeneous data as well as deep architectures; presents a positioning of the dataset bias in the CNN-based feature arena; proposes detailed analyses of popular shallow methods that addresses landmark data selection, kernel embedding, feature alignment, joint feature transformation and classifier adaptation, or the case of limited access to the source data; discusses more recent deep DA methods, including discrepancy-based adaptation networks and adversarial discriminative DA models; addresses domain adaptation problems beyond image categorization, such as a Fisher encoding adaptation for vehicle re-identification, semantic se | 出版日期 | Book 2017 | 关键词 | Computer Vision; Visual Applications; Image Categorization; Pattern Recognition; Data Analytics; Unsuperv | 版次 | 1 | doi | https://doi.org/10.1007/978-3-319-58347-1 | isbn_softcover | 978-3-319-86383-2 | isbn_ebook | 978-3-319-58347-1Series ISSN 2191-6586 Series E-ISSN 2191-6594 | issn_series | 2191-6586 | copyright | Springer International Publishing AG 2017 |
The information of publication is updating
|
|