书目名称 | Unsupervised Domain Adaptation |
副标题 | Recent Advances and |
编辑 | Jingjing Li,Lei Zhu,Zhekai Du |
视频video | |
概述 | Covers not only conventional domain adaptation, but also source-free domain adaptation and active domain adaptation.Presents unique methods to approach domain adaptation from novel perspectives, which |
丛书名称 | Machine Learning: Foundations, Methodologies, and Applications |
图书封面 | 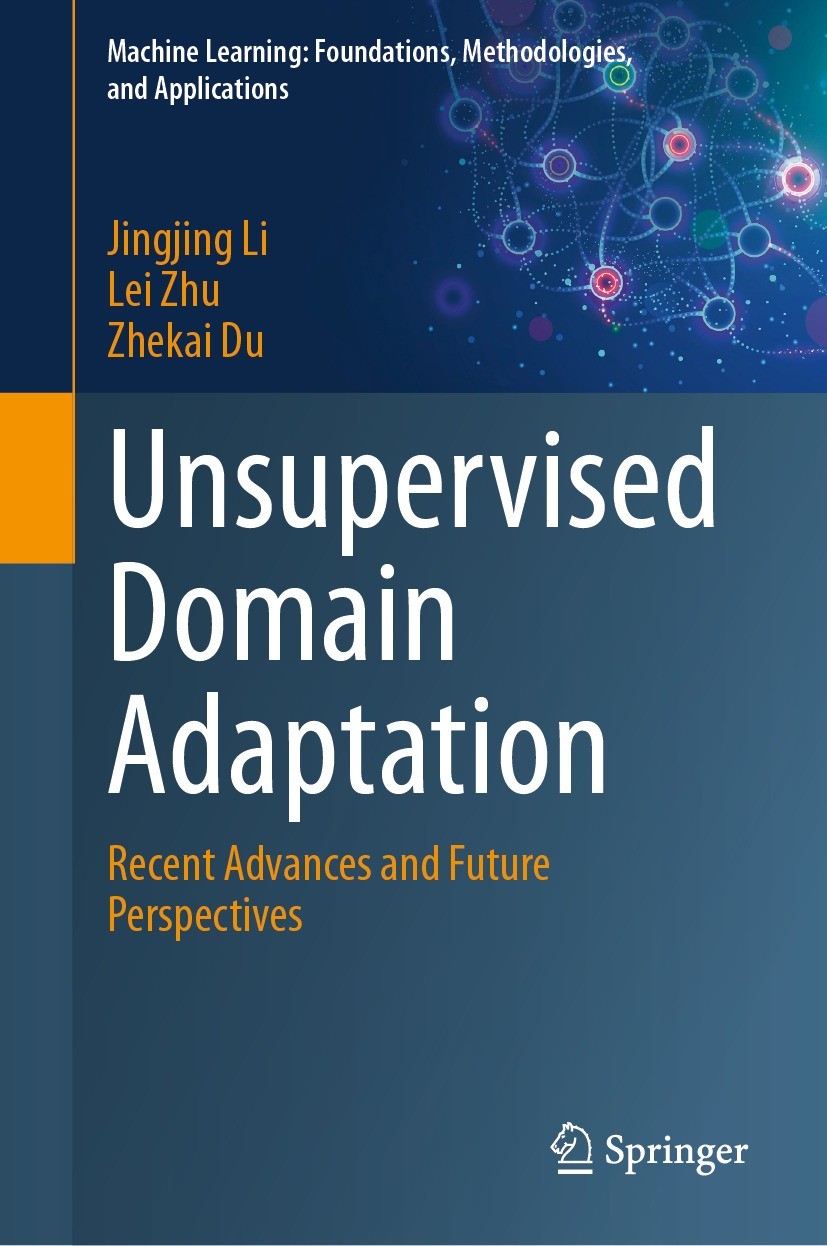 |
描述 | .Unsupervised domain adaptation (UDA) is a challenging problem in machine learning where the model is trained on a source domain with labeled data and tested on a target domain with unlabeled data. In recent years, UDA has received significant attention from the research community due to its applicability in various real-world scenarios. This book provides a comprehensive review of state-of-the-art UDA methods and explores new variants of UDA that have the potential to advance the field...The book begins with a clear introduction to the UDA problem and is mainly organized into four technical sections, each focused on a specific piece of UDA research. The first section covers criterion optimization-based UDA, which aims to learn domain-invariant representations by minimizing the discrepancy between source and target domains. The second section discusses bi-classifier adversarial learning-based UDA, which creatively leverages adversarial learning by conducting a minimax game between the feature extractor and two task classifiers. The third section introduces source-free UDA, a novel UDA setting that does not require any raw data from the source domain. The fourth section presents act |
出版日期 | Book 2024 |
关键词 | Transfer Learning; Adversarial Learning; Source-Free Domain adaptation; Active Domain Adaptation; Unsupe |
版次 | 1 |
doi | https://doi.org/10.1007/978-981-97-1025-6 |
isbn_softcover | 978-981-97-1027-0 |
isbn_ebook | 978-981-97-1025-6Series ISSN 2730-9908 Series E-ISSN 2730-9916 |
issn_series | 2730-9908 |
copyright | The Editor(s) (if applicable) and The Author(s), under exclusive license to Springer Nature Singapor |