书目名称 | Statistical Learning from a Regression Perspective | 编辑 | Richard A. Berk | 视频video | | 概述 | Provides accompanying, fully updated R code.Evaluates the ethical and political implications of the application of algorithmic methods.Features a new chapter on deep learning | 丛书名称 | Springer Texts in Statistics | 图书封面 | 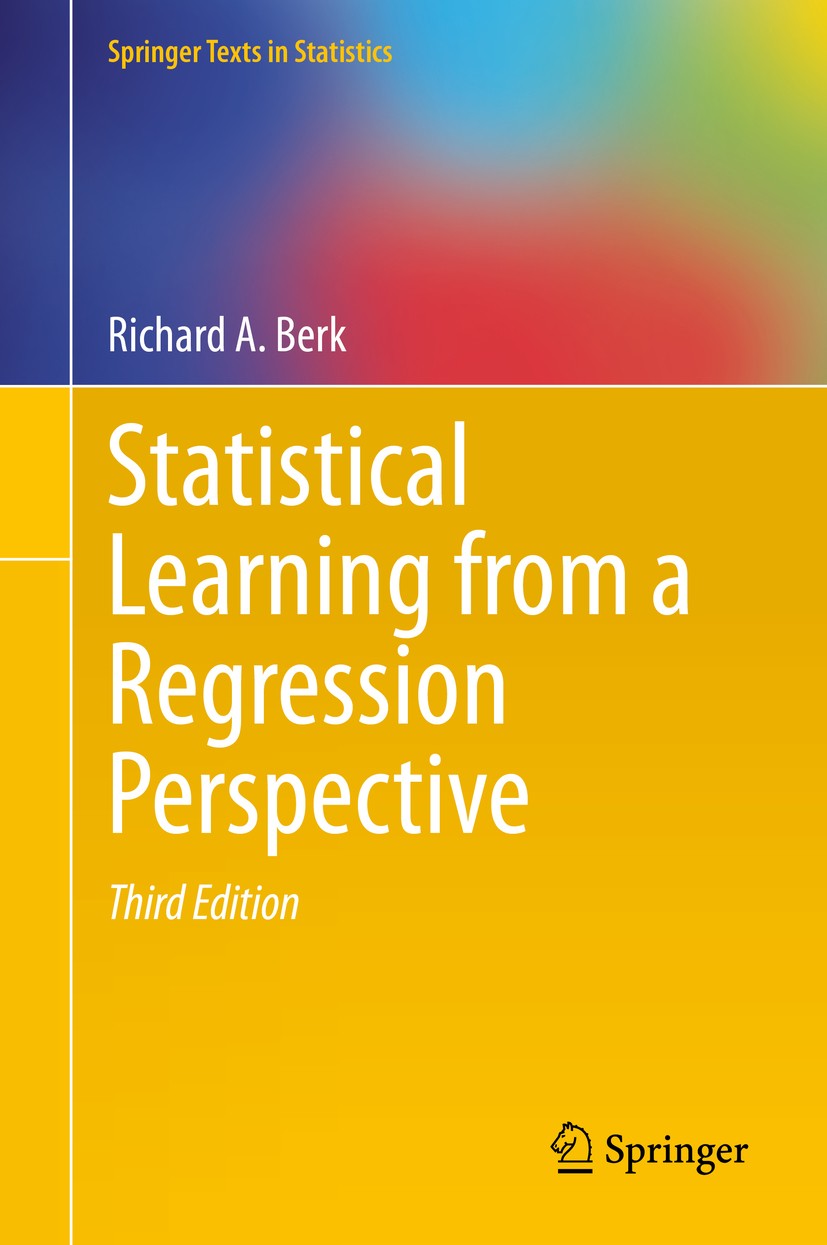 | 描述 | .This textbook considers statistical learning applications when interest centers on the conditional distribution of a response variable, given a set of predictors, and in the absence of a credible model that can be specified before the data analysis begins. Consistent with modern data analytics, it emphasizes that a proper statistical learning data analysis depends in an integrated fashion on sound data collection, intelligent data management, appropriate statistical procedures, and an accessible interpretation of results. The unifying theme is that supervised learning properly can be seen as a form of regression analysis. Key concepts and procedures are illustrated with a large number of real applications and their associated code in R, with an eye toward practical implications. The growing integration of computer science and statistics is well represented including the occasional, but salient, tensions that result. Throughout, there are links to the big picture...The third edition considers significant advances in recent years, among which are:..the development of overarching, conceptual frameworks for statistical learning;.the impact of “big data” on statistical learning;.the n | 出版日期 | Textbook 2020Latest edition | 关键词 | classification; random forests; support vector machines; machine learning; boosting; bagging; decision tre | 版次 | 3 | doi | https://doi.org/10.1007/978-3-030-40189-4 | isbn_softcover | 978-3-030-42923-2 | isbn_ebook | 978-3-030-40189-4Series ISSN 1431-875X Series E-ISSN 2197-4136 | issn_series | 1431-875X | copyright | Springer Nature Switzerland AG 2020 |
The information of publication is updating
|
|