书目名称 | Reinforcement Learning | 副标题 | State-of-the-Art | 编辑 | Marco Wiering,Martijn Otterlo | 视频video | | 概述 | Covers all important recent developments in reinforcement learning.Very good introduction and explanation of the different emerging areas in Reinforcement Learning.Includes a survey of previous papers | 丛书名称 | Adaptation, Learning, and Optimization | 图书封面 | 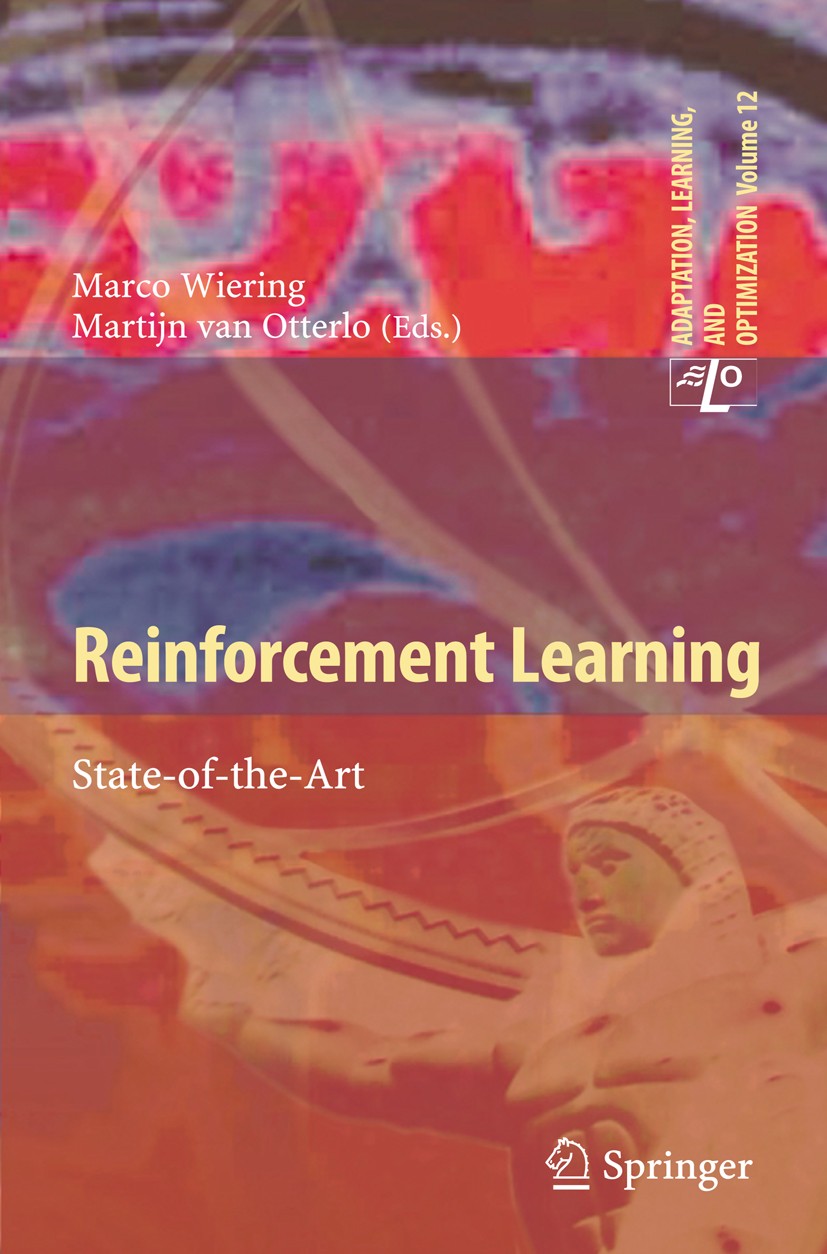 | 描述 | .Reinforcement learning encompasses both a science of adaptive behavior of rational beings in uncertain environments and a computational methodology for finding optimal behaviors for challenging problems in control, optimization and adaptive behavior of intelligent agents. As a field, reinforcement learning has progressed tremendously in the past decade..The main goal of this book is to present an up-to-date series of survey articles on the main contemporary sub-fields of reinforcement learning. This includes surveys on partially observable environments, hierarchical task decompositions, relational knowledge representation and predictive state representations. Furthermore, topics such as transfer, evolutionary methods and continuous spaces in reinforcement learning are surveyed. In addition, several chapters review reinforcement learning methods in robotics, in games, and in computational neuroscience. In total seventeen different subfields are presented by mostly young experts in those areas, and together they truly represent a state-of-the-art of current reinforcement learning research..Marco Wiering works at the artificial intelligence department of the University of Groningen i | 出版日期 | Book 2012 | 关键词 | Artificial Intelligence; Computational Intelligence; Decision-Theoretic Planning; Dynamic Programming; M | 版次 | 1 | doi | https://doi.org/10.1007/978-3-642-27645-3 | isbn_softcover | 978-3-642-44685-6 | isbn_ebook | 978-3-642-27645-3Series ISSN 1867-4534 Series E-ISSN 1867-4542 | issn_series | 1867-4534 | copyright | Springer-Verlag Berlin Heidelberg 2012 |
The information of publication is updating
|
|