书目名称 | Open Problems in Spectral Dimensionality Reduction |
编辑 | Harry Strange,Reyer Zwiggelaar |
视频video | |
概述 | Provides a clear and concise overview of spectral dimensionality reduction.Offers uniquely practical knowledge without requiring a background in the area.Suggests interesting starting points for futur |
丛书名称 | SpringerBriefs in Computer Science |
图书封面 | 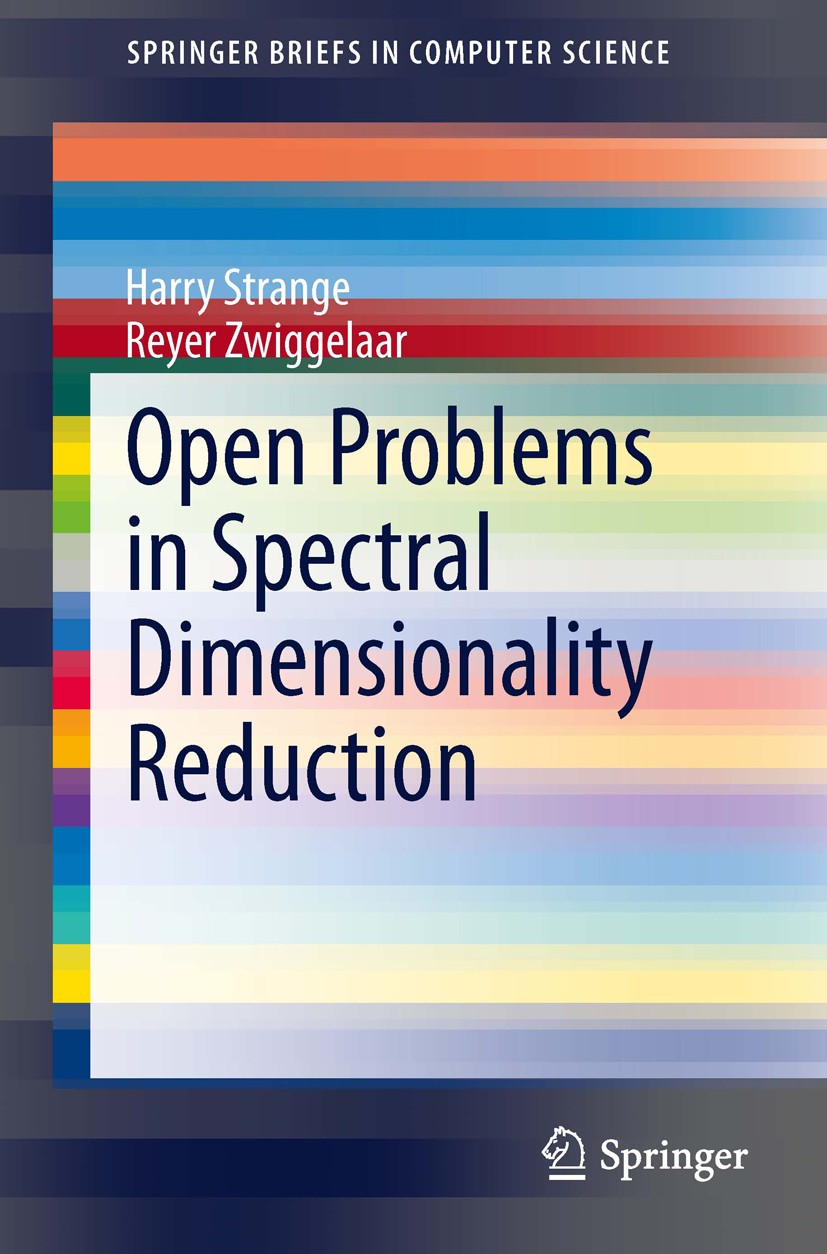 |
描述 | The last few years have seen a great increase in the amount of data available to scientists, yet many of the techniques used to analyse this data cannot cope with such large datasets. Therefore, strategies need to be employed as a pre-processing step to reduce the number of objects or measurements whilst retaining important information. Spectral dimensionality reduction is one such tool for the data processing pipeline. Numerous algorithms and improvements have been proposed for the purpose of performing spectral dimensionality reduction, yet there is still no gold standard technique. This book provides a survey and reference aimed at advanced undergraduate and postgraduate students as well as researchers, scientists, and engineers in a wide range of disciplines. Dimensionality reduction has proven useful in a wide range of problem domains and so this book will be applicable to anyone with a solid grounding in statistics and computer science seeking to apply spectral dimensionality to their work. |
出版日期 | Book 2014 |
关键词 | Big Data; Machine Learning; Manifold Learning Algorithms; Nonlinear Dimensionality Reduction (NLDR); Pri |
版次 | 1 |
doi | https://doi.org/10.1007/978-3-319-03943-5 |
isbn_softcover | 978-3-319-03942-8 |
isbn_ebook | 978-3-319-03943-5Series ISSN 2191-5768 Series E-ISSN 2191-5776 |
issn_series | 2191-5768 |
copyright | The Author(s) 2014 |