书目名称 | New Generation Artificial Intelligence-Driven Diagnosis and Maintenance Techniques | 副标题 | Advanced Machine Lea | 编辑 | Guangrui Wen,Zihao Lei,Xin Huang | 视频video | | 概述 | Offers in-depth discussion on the improvement of the accuracy and efficiency of intelligent diagnosis and maintenance.Shares many novel tips and insights into intelligent diagnosis and maintenance.Inc | 丛书名称 | Smart Sensors, Measurement and Instrumentation | 图书封面 | 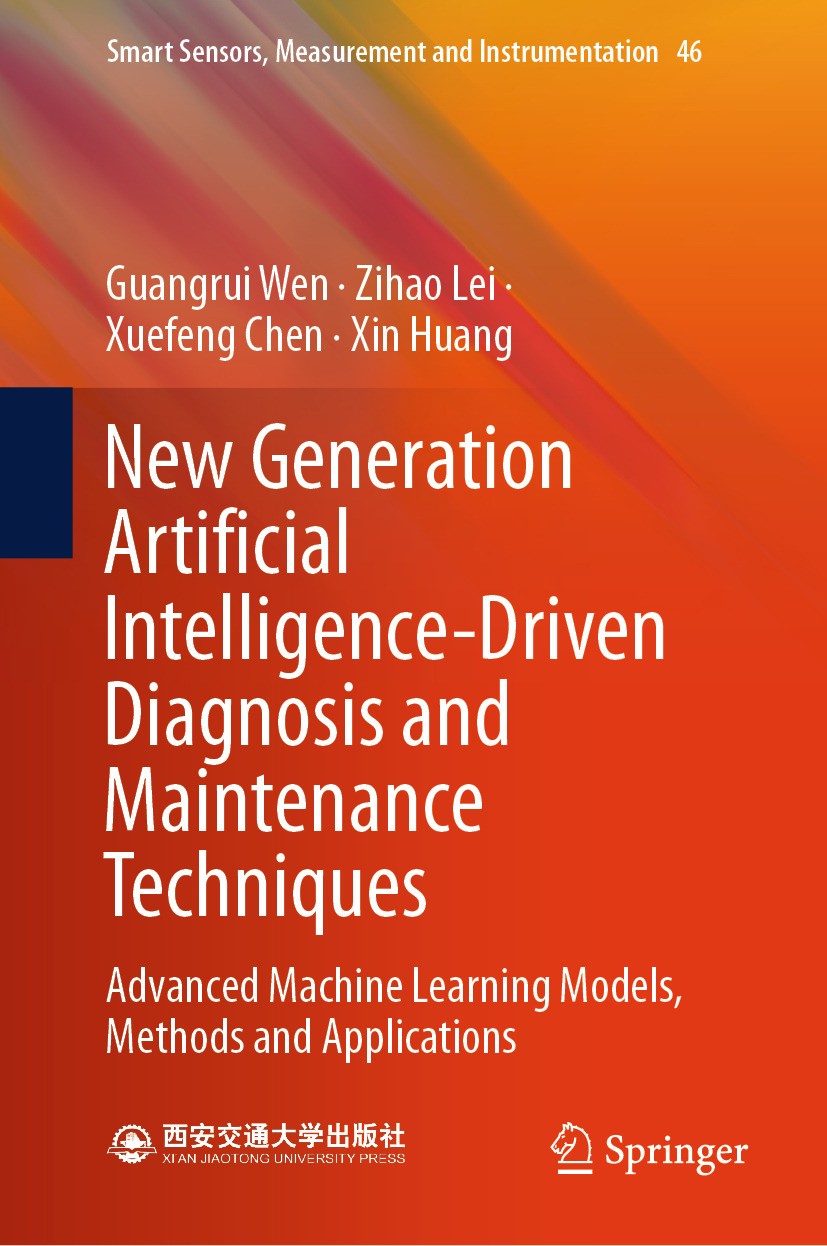 | 描述 | .The intelligent diagnosis and maintenance of the machine mainly includes condition monitoring, fault diagnosis, performance degradation assessment and remaining useful life prediction, which plays an important role in protecting people‘s lives and property. In actual engineering scenarios, machine users always hope to use an automatic method to shorten the maintenance cycle and improve the accuracy of fault diagnosis and prognosis. In the past decade, Artificial Intelligence applications have flourished in many different fields, which also provide powerful tools for intelligent diagnosis and maintenance...This book highlights the latest advances and trends in new generation artificial intelligence-driven techniques, including knowledge-driven deep learning, transfer learning, adversarial learning, complex network, graph neural network and multi-source information fusion, for diagnosis and maintenance of rotating machinery. Its primary focus is on the utilization of advanced artificial intelligence techniques to monitor, diagnose, and perform predictive maintenance of critical structures and machines, such as aero-engine, gas turbines, wind turbines, and machine tools...The main ma | 出版日期 | Book 2024 | 关键词 | Prognostics Health Management (PHM); Abnormal Detection; Condition Monitoring; Fault Diagnosis and Prog | 版次 | 1 | doi | https://doi.org/10.1007/978-981-97-1176-5 | isbn_softcover | 978-981-97-1178-9 | isbn_ebook | 978-981-97-1176-5Series ISSN 2194-8402 Series E-ISSN 2194-8410 | issn_series | 2194-8402 | copyright | Xi‘an Jiaotong University Press 2024 |
The information of publication is updating
|
|