书目名称 | Normalization Techniques in Deep Learning | 编辑 | Lei Huang | 视频video | | 概述 | Presents valuable guidelines for selecting normalization techniques for use in training deep neural networks.Discusses the research landscape of normalization techniques and covers the needed methods, | 丛书名称 | Synthesis Lectures on Computer Vision | 图书封面 | 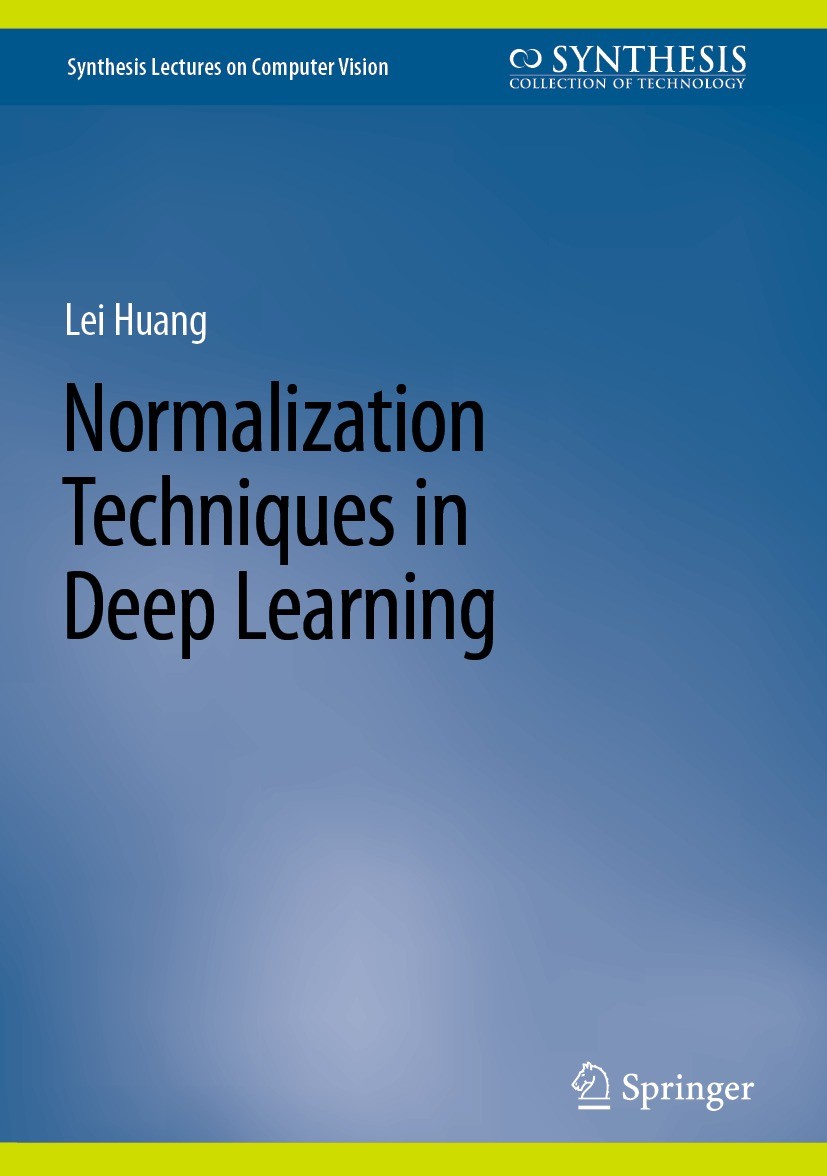 | 描述 | This book presents and surveys normalization techniques with a deep analysis in training deep neural networks. In addition, the author provides technical details in designing new normalization methods and network architectures tailored to specific tasks. Normalization methods can improve the training stability, optimization efficiency, and generalization ability of deep neural networks (DNNs) and have become basic components in most state-of-the-art DNN architectures. The author provides guidelines for elaborating, understanding, and applying normalization methods. This book is ideal for readers working on the development of novel deep learning algorithms and/or their applications to solve practical problems in computer vision and machine learning tasks. The book also serves as a resource researchers, engineers, and students who are new to the field and need to understand and train DNNs.. | 出版日期 | Book 2022 | 关键词 | Computer Vision; Deep Neural Networks (DNNs); Normalization Techniques; Machine Learning; Artificial Int | 版次 | 1 | doi | https://doi.org/10.1007/978-3-031-14595-7 | isbn_softcover | 978-3-031-14597-1 | isbn_ebook | 978-3-031-14595-7Series ISSN 2153-1056 Series E-ISSN 2153-1064 | issn_series | 2153-1056 | copyright | The Editor(s) (if applicable) and The Author(s), under exclusive license to Springer Nature Switzerl |
The information of publication is updating
|
|