书目名称 | Nonlinear Principal Component Analysis and Its Applications | 编辑 | Yuichi Mori,Masahiro Kuroda,Naomichi Makino | 视频video | | 概述 | Shows that PCA, nonlinear PCA, and MCA can be integrated as a single formulation, which can easily be extended to several applications.Provides an acceleration algorithm that speeds up the convergent | 丛书名称 | SpringerBriefs in Statistics | 图书封面 | 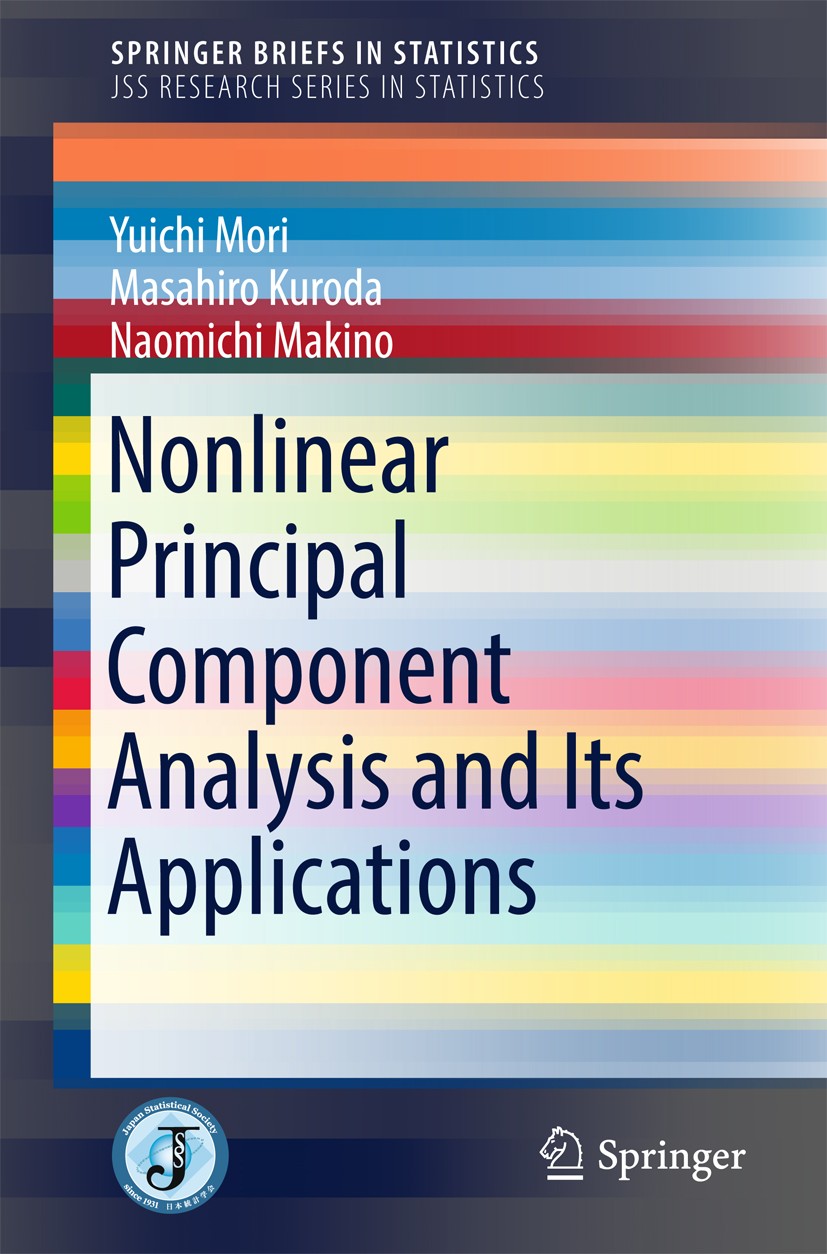 | 描述 | This book expounds the principle and related applications of nonlinear principal component analysis (PCA), which is useful method to analyze mixed measurement levels data. .In the part dealing with the principle, after a brief introduction of ordinary PCA, a PCA for categorical data (nominal and ordinal) is introduced as nonlinear PCA, in which an optimal scaling technique is used to quantify the categorical variables. The alternating least squares (ALS) is the main algorithm in the method. Multiple correspondence analysis (MCA), a special case of nonlinear PCA, is also introduced. All formulations in these methods are integrated in the same manner as matrix operations. Because any measurement levels data can be treated consistently as numerical data and ALS is a very powerful tool for estimations, the methods can be utilized in a variety of fields such as biometrics, econometrics, psychometrics, and sociology. .In the applications part of the book, four applications are introduced: variable selection for mixed measurement levels data, sparse MCA, joint dimension reduction and clustering methods for categorical data, and acceleration of ALS computation. The variable selection metho | 出版日期 | Book 2016 | 关键词 | Alternating Least Squares; Mixed Measurement Level Data; Multiple Correspondence Analysis; Nonlinear PC | 版次 | 1 | doi | https://doi.org/10.1007/978-981-10-0159-8 | isbn_softcover | 978-981-10-0157-4 | isbn_ebook | 978-981-10-0159-8Series ISSN 2191-544X Series E-ISSN 2191-5458 | issn_series | 2191-544X | copyright | The Author(s) 2016 |
The information of publication is updating
|
|