书目名称 | Nonlinear Dimensionality Reduction | 编辑 | John A. Lee,Michel Verleysen | 视频video | | 概述 | Covers a wide range of methods to reduce the dimensionality of data and presents a unifying point of view.Includes supplementary material: | 丛书名称 | Information Science and Statistics | 图书封面 | 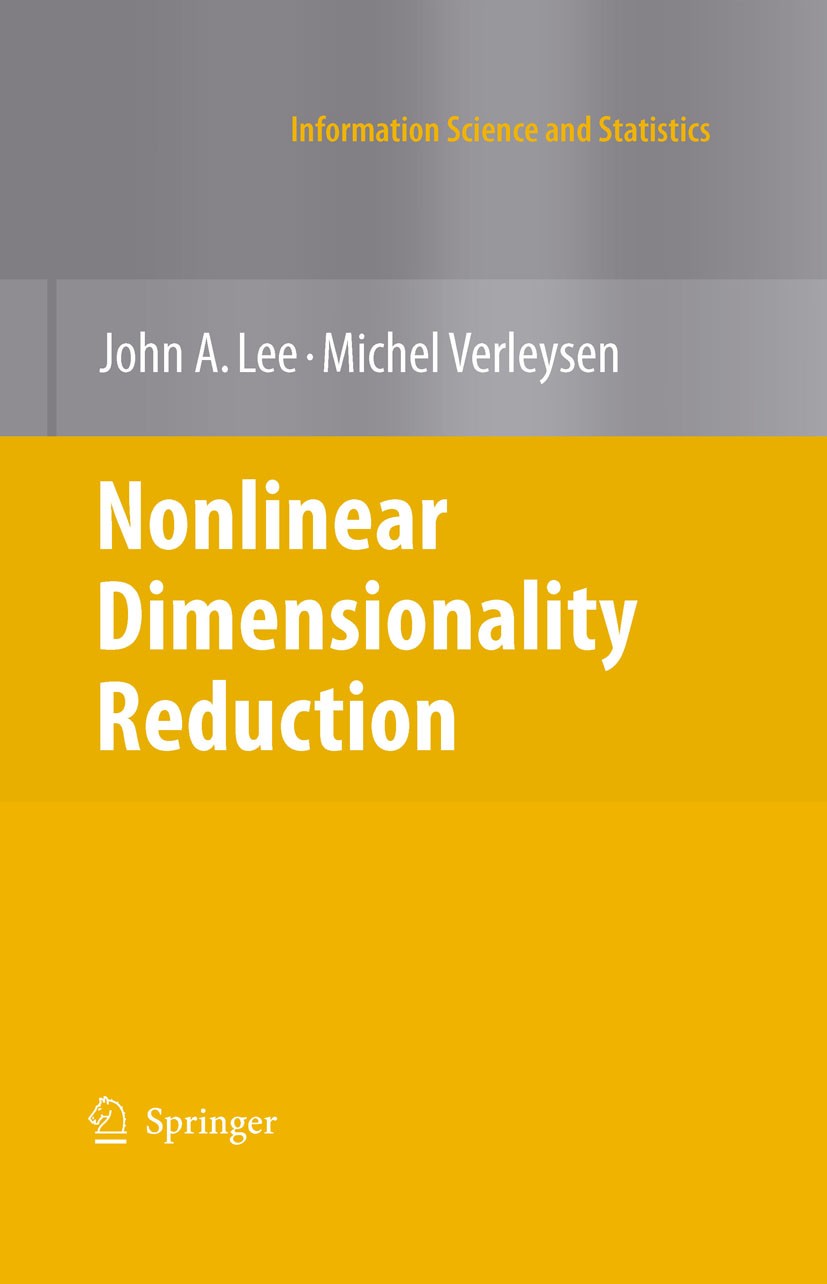 | 描述 | .Methods of dimensionality reduction provide a way to understand and visualize the structure of complex data sets. Traditional methods like principal component analysis and classical metric multidimensional scaling suffer from being based on linear models. Until recently, very few methods were able to reduce the data dimensionality in a nonlinear way. However, since the late nineties, many new methods have been developed and nonlinear dimensionality reduction, also called manifold learning, has become a hot topic. New advances that account for this rapid growth are, e.g. the use of graphs to represent the manifold topology, and the use of new metrics like the geodesic distance. In addition, new optimization schemes, based on kernel techniques and spectral decomposition, have lead to spectral embedding, which encompasses many of the recently developed methods. ..This book describes existing and advanced methods to reduce the dimensionality of numerical databases. For each method, the description starts from intuitive ideas, develops the necessary mathematical details, and ends by outlining the algorithmic implementation. Methods are compared with each other with the help of differen | 出版日期 | Book 2007 | 关键词 | Principal component analysis; algorithms; computer; database; learning; multidimensional scaling; optimiza | 版次 | 1 | doi | https://doi.org/10.1007/978-0-387-39351-3 | isbn_softcover | 978-1-4419-2288-5 | isbn_ebook | 978-0-387-39351-3Series ISSN 1613-9011 Series E-ISSN 2197-4128 | issn_series | 1613-9011 | copyright | Springer-Verlag New York 2007 |
The information of publication is updating
|
|