书目名称 | Neural Information Processing |
副标题 | 22nd International C |
编辑 | Sabri Arik,Tingwen Huang,Qingshan Liu |
视频video | |
概述 | Includes supplementary material: |
丛书名称 | Lecture Notes in Computer Science |
图书封面 | 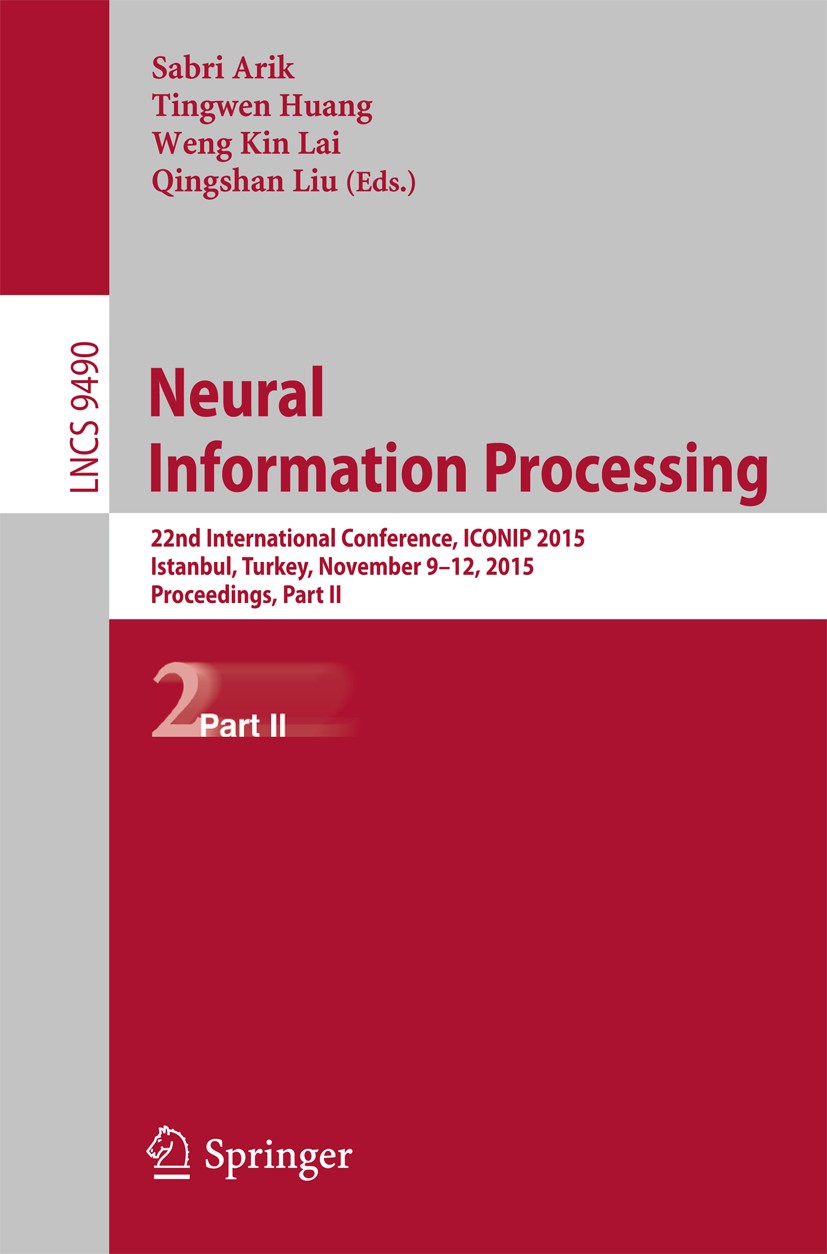 |
描述 | .The four volume set LNCS 9489, LNCS 9490, LNCS 9491, andLNCS 9492 constitutes the proceedings of the 22nd International Conference onNeural Information Processing, ICONIP 2015, held in Istanbul, Turkey, inNovember 2015...The 231 full papers presented were carefully reviewed andselected from 375 submissions. The 4 volumes represent topical sectionscontaining articles on Learning Algorithms and Classification Systems;Artificial Intelligence and Neural Networks: Theory, Design, and Applications;Image and Signal Processing; and Intelligent Social Networks.. |
出版日期 | Conference proceedings 2015 |
关键词 | Biometrics; data mining; genetic algorithm; pattern recognition; semantic Web; artificial neural networks |
版次 | 1 |
doi | https://doi.org/10.1007/978-3-319-26535-3 |
isbn_softcover | 978-3-319-26534-6 |
isbn_ebook | 978-3-319-26535-3Series ISSN 0302-9743 Series E-ISSN 1611-3349 |
issn_series | 0302-9743 |
copyright | Springer International Publishing Switzerland 2015 |