书目名称 | Neural Information Processing | 副标题 | 21st International C | 编辑 | Chu Kiong Loo,Keem Siah Yap,Kaizhu Huang | 视频video | | 丛书名称 | Lecture Notes in Computer Science | 图书封面 | 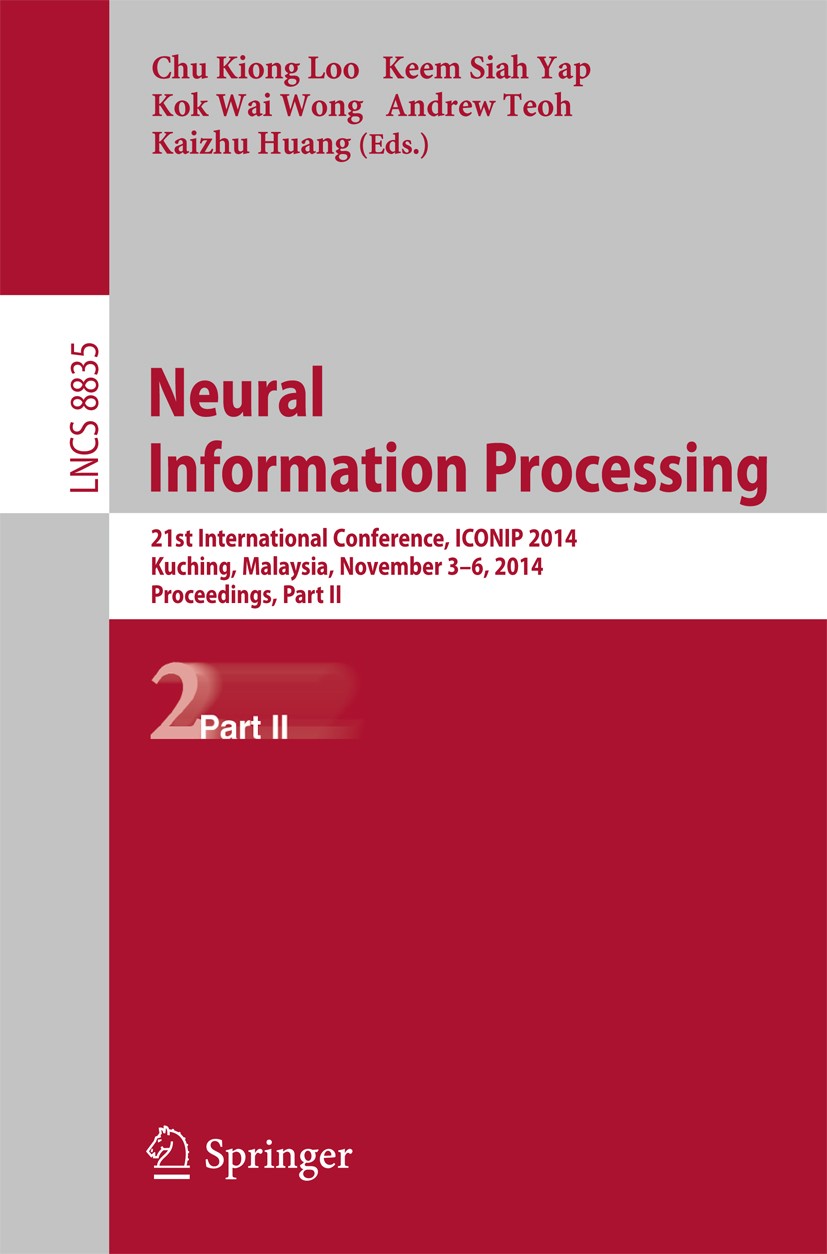 | 描述 | The three volume set LNCS 8834, LNCS 8835, and LNCS 8836 constitutes the proceedings of the 20th International Conference on Neural Information Processing, ICONIP 2014, held in Kuching, Malaysia, in November 2014. The 231 full papers presented were carefully reviewed and selected from 375 submissions. The selected papers cover major topics of theoretical research, empirical study, and applications of neural information processing research. The 3 volumes represent topical sections containing articles on cognitive science, neural networks and learning systems, theory and design, applications, kernel and statistical methods, evolutionary computation and hybrid intelligent systems, signal and image processing, and special sessions intelligent systems for supporting decision, making processes,theories and applications, cognitive robotics, and learning systems for social network and web mining. | 出版日期 | Conference proceedings 2014 | 关键词 | activity recognition; artificial intelligence; big data; bio-inspired computing; brain-computer interfac | 版次 | 1 | doi | https://doi.org/10.1007/978-3-319-12640-1 | isbn_softcover | 978-3-319-12639-5 | isbn_ebook | 978-3-319-12640-1Series ISSN 0302-9743 Series E-ISSN 1611-3349 | issn_series | 0302-9743 | copyright | Springer International Publishing Switzerland 2014 |
The information of publication is updating
|
|