书目名称 | Neural Approximations for Optimal Control and Decision |
编辑 | Riccardo Zoppoli,Marcello Sanguineti,Thomas Parisi |
视频video | |
概述 | Material is an up-to-date treatment of optimal control problems which have thus far been difficult to solve.Applications selected have major current interest: routing in communications networks, freew |
丛书名称 | Communications and Control Engineering |
图书封面 | 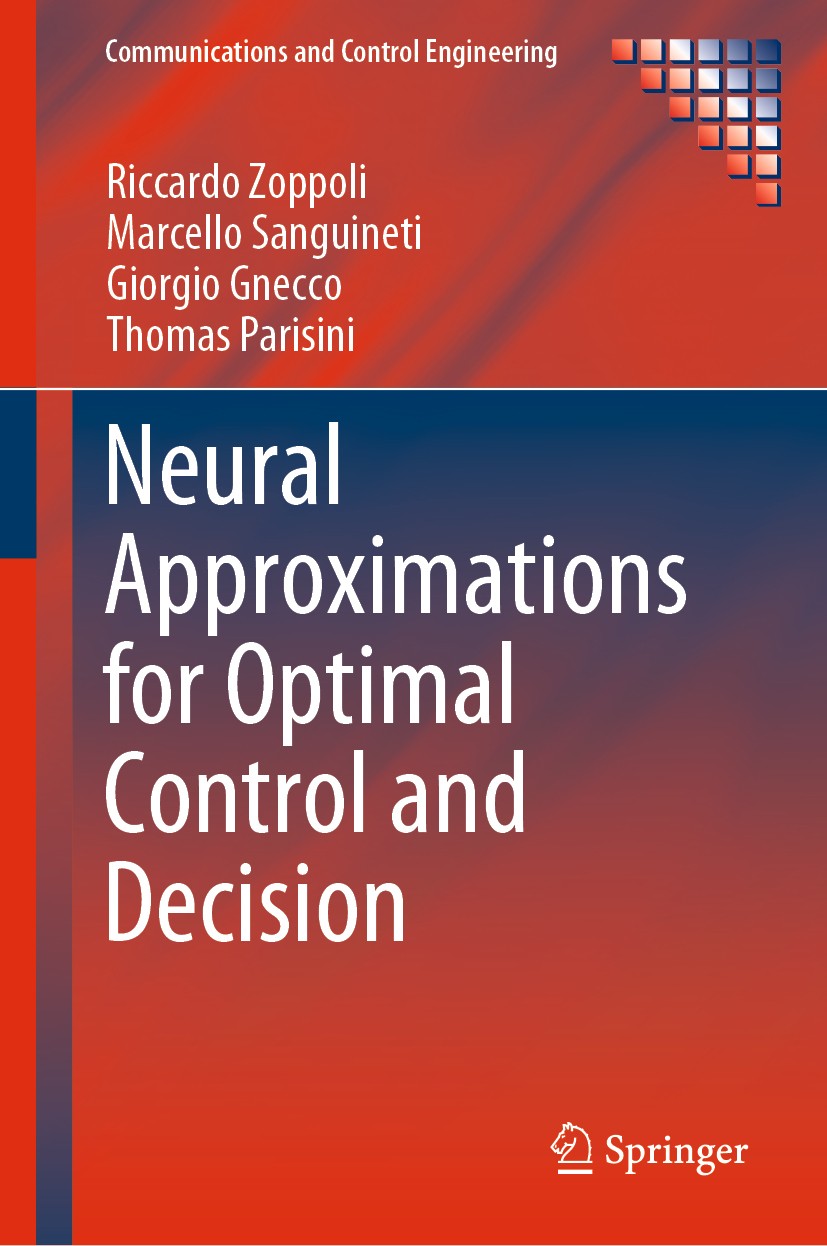 |
描述 | .Neural Approximations for Optimal Control and Decision. provides a comprehensive methodology for the approximate solution of functional optimization problems using neural networks and other nonlinear approximators where the use of traditional optimal control tools is prohibited by complicating factors like non-Gaussian noise, strong nonlinearities, large dimension of state and control vectors, etc...Features of the text include:..• a general functional optimization framework;..• thorough illustration of recent theoretical insights into the approximate solutions of complex functional optimization problems;..• comparison of classical and neural-network based methods of approximate solution;..• bounds to the errors of approximate solutions;..• solution algorithms for optimal control and decision in deterministic or stochastic environments with perfect or imperfect state measurements over a finite or infinite time horizon and with onedecision maker or several;..• applications of current interest: routing in communications networks, traffic control, water resource management, etc.; and..• numerous, numerically detailed examples...The authors’ diverse backgrounds in systems and control |
出版日期 | Book 2020 |
关键词 | Bellman‘s Curse of Dimensionality; Control; Control Engineering; Control Theory; Decision Engineering; Ne |
版次 | 1 |
doi | https://doi.org/10.1007/978-3-030-29693-3 |
isbn_ebook | 978-3-030-29693-3Series ISSN 0178-5354 Series E-ISSN 2197-7119 |
issn_series | 0178-5354 |
copyright | Springer Nature Switzerland AG 2020 |