书目名称 | Multiple Fuzzy Classification Systems |
编辑 | Rafał Scherer |
视频video | |
概述 | Novel approach for exploratory data analysis with ensembles of various neuro-fuzzy systems.Derivation of various ensemble architectures that are able to.work with missing data.Written by an expert in |
丛书名称 | Studies in Fuzziness and Soft Computing |
图书封面 | 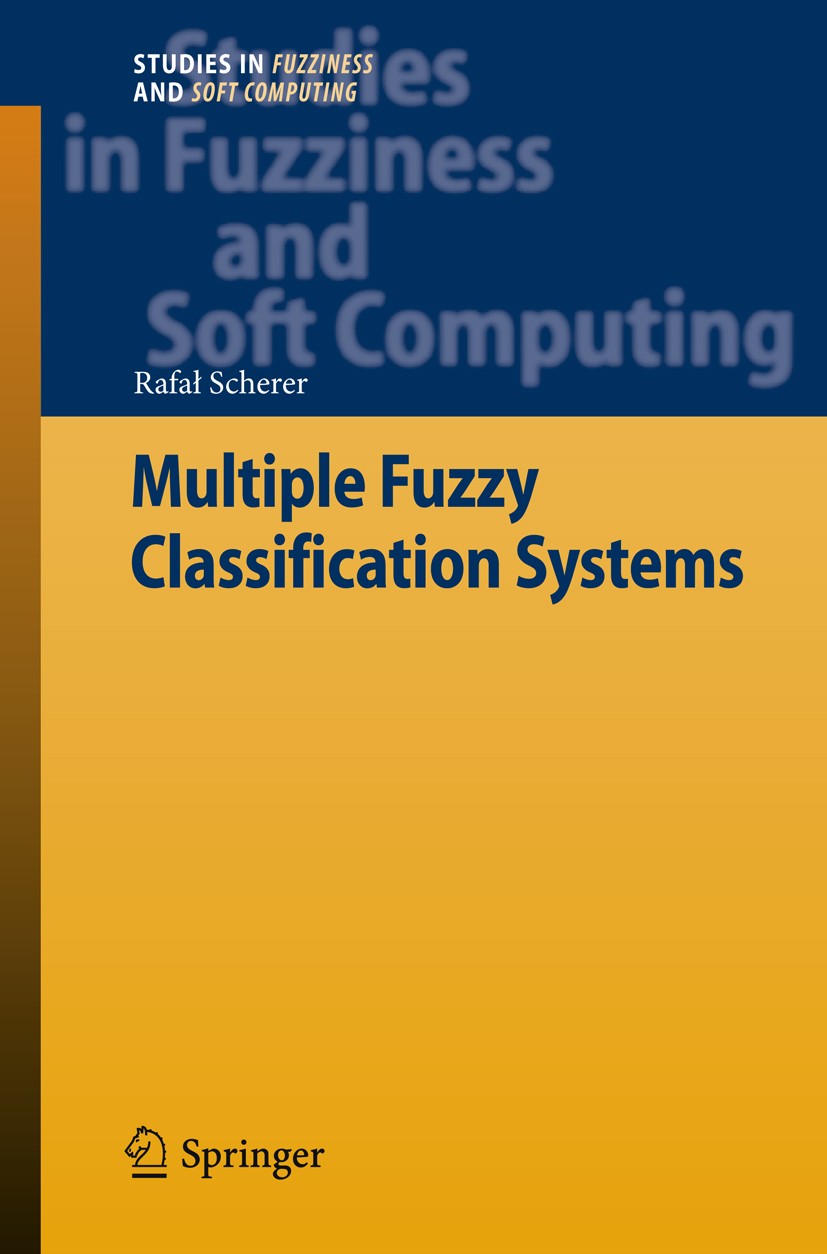 |
描述 | .Fuzzy classifiers are important tools in exploratory data analysis, which is a vital set of methods used in various engineering, scientific and business applications. Fuzzy classifiers use fuzzy rules and do not require assumptions common to statistical classification. Rough set theory is useful when data sets are incomplete. It defines a formal approximation of crisp sets by providing the lower and the upper approximation of the original set. Systems based on rough sets have natural ability to work on such data and incomplete vectors do not have to be preprocessed before classification. To achieve better performance than existing machine learning systems, fuzzy classifiers and rough sets can be combined in ensembles. Such ensembles consist of a finite set of learning models, usually weak learners. .The present book discusses the three aforementioned fields – fuzzy systems, rough sets and ensemble techniques. As the trained ensemble should represent a single hypothesis, a lot of attention is placed on the possibility to combine fuzzy rules from fuzzy systems being members of classification ensemble. Furthermore, an emphasis is placed on ensembles that can work on incomplete data, thanks to |
出版日期 | Book 2012 |
关键词 | Boosting; Classifiers; Decision Making; Ensemble Techniques; Fuzzy Systems; Mamdani Fuzzy Systems; Negativ |
版次 | 1 |
doi | https://doi.org/10.1007/978-3-642-30604-4 |
isbn_softcover | 978-3-642-43657-4 |
isbn_ebook | 978-3-642-30604-4Series ISSN 1434-9922 Series E-ISSN 1860-0808 |
issn_series | 1434-9922 |
copyright | Springer-Verlag Berlin Heidelberg 2012 |