书目名称 | Multi-Level Bayesian Models for Environment Perception | 编辑 | Csaba Benedek | 视频video | | 概述 | Provides novel Bayesian models for complex environment perception problems.Describes spatial and temporal extensions of widely used probabilistic inference methods.Provides real-world application exam | 图书封面 | 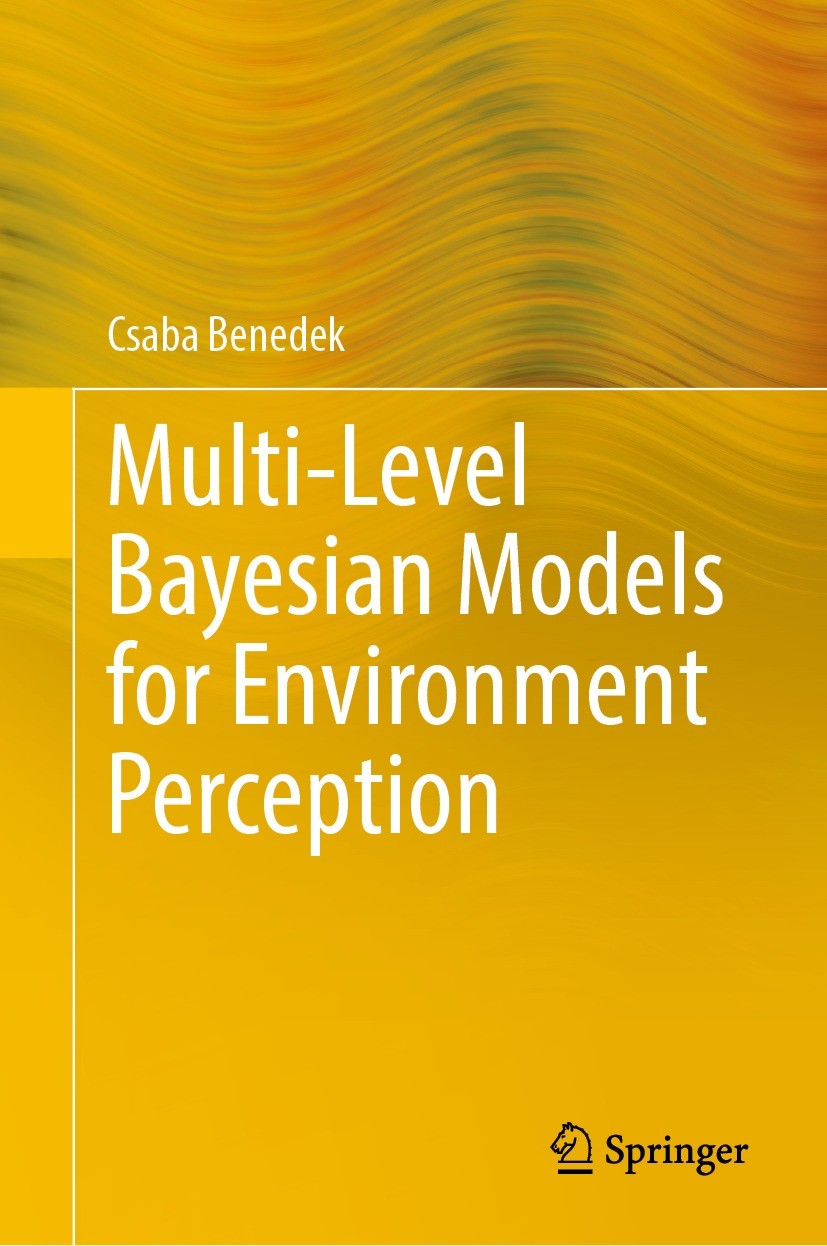 | 描述 | .This book deals with selected problems of machine perception, using various 2D and 3D imaging sensors. It proposes several new original methods, and also provides a detailed state-of-the-art overview of existing techniques for automated, multi-level interpretation of the observed static or dynamic environment. To ensure a sound theoretical basis of the new models, the surveys and algorithmic developments are performed in well-established Bayesian frameworks. Low level scene understanding functions are formulated as various image segmentation problems, where the advantages of probabilistic inference techniques such as Markov Random Fields (MRF) or Mixed Markov Models are considered. For the object level scene analysis, the book mainly relies on the literature of Marked Point Process (MPP) approaches, which consider strong geometric and prior interaction constraints in object population modeling. In particular, key developments are introduced in the spatial hierarchical decomposition of the observed scenarios, and in the temporal extension of complex MRF and MPP models. Apart from utilizing conventional optical sensors, case studies are provided on passive radar (ISAR) and Lidar-b | 出版日期 | Book 2022 | 关键词 | Bayesian modeling; Stochastic optimization; Markov Random Fields; Marked Point Processes; Computer visio | 版次 | 1 | doi | https://doi.org/10.1007/978-3-030-83654-2 | isbn_softcover | 978-3-030-83656-6 | isbn_ebook | 978-3-030-83654-2 | copyright | The Editor(s) (if applicable) and The Author(s), under exclusive license to Springer Nature Switzerl |
The information of publication is updating
|
|