书目名称 | Monte Carlo Methods in Bayesian Computation | 编辑 | Ming-Hui Chen,Qi-Man Shao,Joseph G. Ibrahim | 视频video | | 概述 | Includes supplementary material: | 丛书名称 | Springer Series in Statistics | 图书封面 | 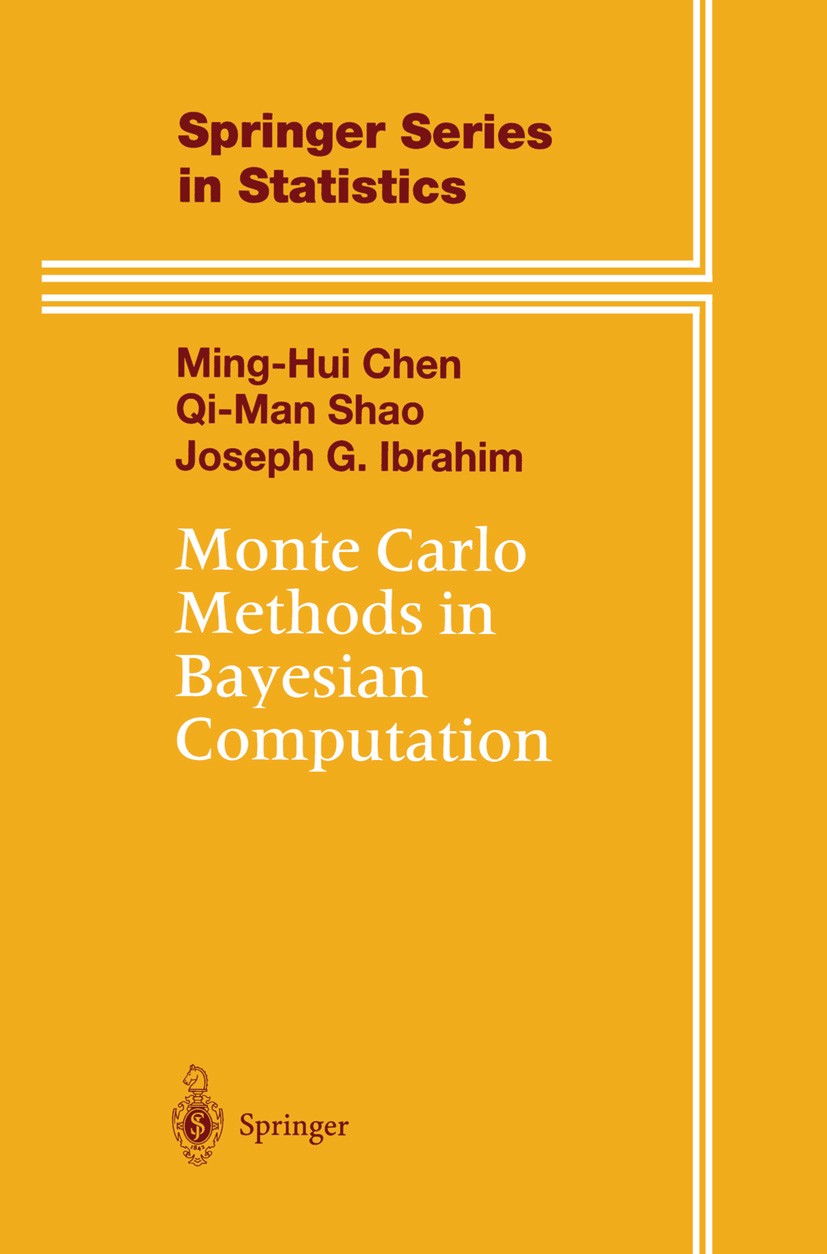 | 描述 | Sampling from the posterior distribution and computing posterior quanti ties of interest using Markov chain Monte Carlo (MCMC) samples are two major challenges involved in advanced Bayesian computation. This book examines each of these issues in detail and focuses heavily on comput ing various posterior quantities of interest from a given MCMC sample. Several topics are addressed, including techniques for MCMC sampling, Monte Carlo (MC) methods for estimation of posterior summaries, improv ing simulation accuracy, marginal posterior density estimation, estimation of normalizing constants, constrained parameter problems, Highest Poste rior Density (HPD) interval calculations, computation of posterior modes, and posterior computations for proportional hazards models and Dirichlet process models. Also extensive discussion is given for computations in volving model comparisons, including both nested and nonnested models. Marginal likelihood methods, ratios of normalizing constants, Bayes fac tors, the Savage-Dickey density ratio, Stochastic Search Variable Selection (SSVS), Bayesian Model Averaging (BMA), the reverse jump algorithm, and model adequacy using predictive and latent | 出版日期 | Book 2000 | 关键词 | Bayesian Computation; Estimator; Likelihood; Logistic Regression; Markov Chain; Monte Carlo Methods; Time | 版次 | 1 | doi | https://doi.org/10.1007/978-1-4612-1276-8 | isbn_softcover | 978-1-4612-7074-4 | isbn_ebook | 978-1-4612-1276-8Series ISSN 0172-7397 Series E-ISSN 2197-568X | issn_series | 0172-7397 | copyright | Springer Science+Business Media New York 2000 |
The information of publication is updating
|
|