书目名称 | Modern Deep Learning for Tabular Data | 副标题 | Novel Approaches to | 编辑 | Andre Ye,Zian Wang | 视频video | | 概述 | Explains deep learning applications to tabular data, documenting novel methods and techniques.Exposes and synthesizes lesser-known deep learning tools and techniques backed by recent research.Apply co | 图书封面 | 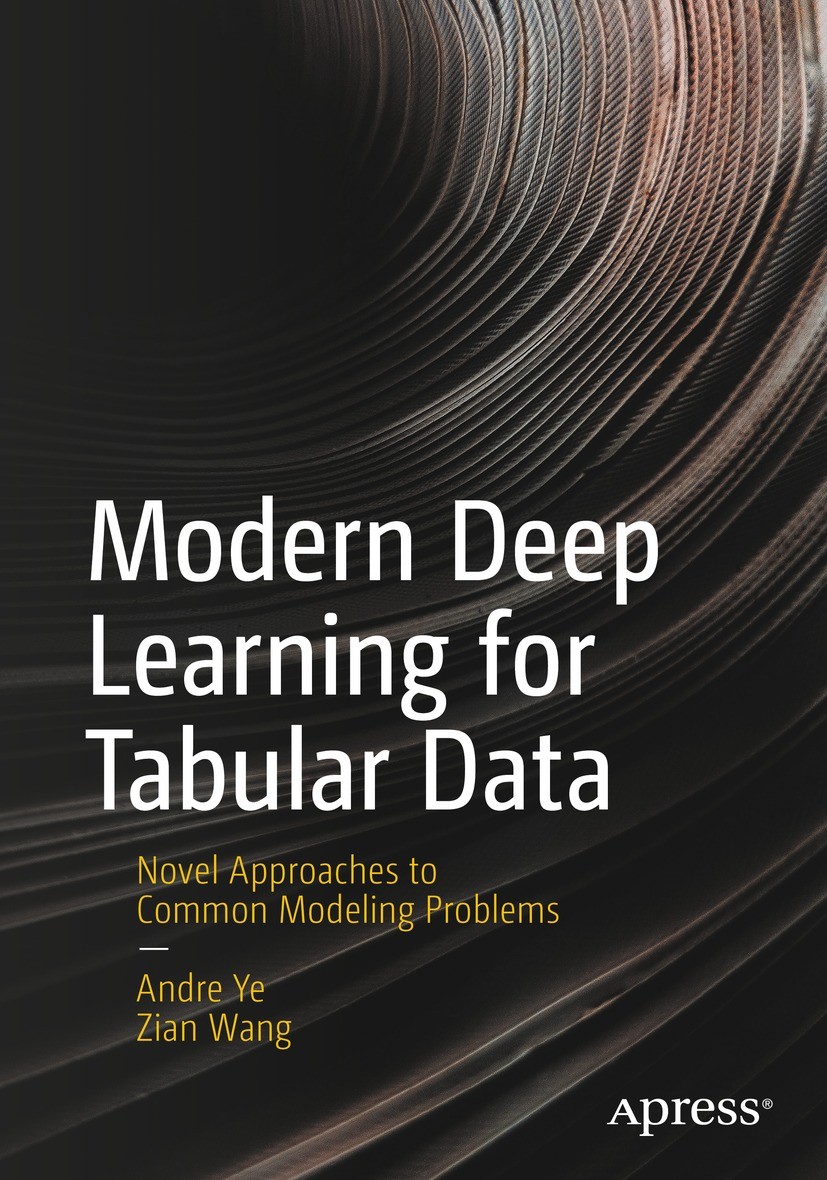 | 描述 | .Deep learning is one of the most powerful tools in the modern artificial intelligence landscape. While having been predominantly applied to highly specialized image, text, and signal datasets, this book synthesizes and presents novel deep learning approaches to a seemingly unlikely domain – tabular data. Whether for finance, business, security, medicine, or countless other domain, deep learning can help mine and model complex patterns in tabular data – an incredibly ubiquitous form of structured data...Part I of the book offers a rigorous overview of machine learning principles, algorithms, and implementation skills relevant to holistically modeling and manipulating tabular data. Part II studies five dominant deep learning model designs – Artificial Neural Networks, Convolutional Neural Networks, Recurrent Neural Networks, Attention and Transformers, and Tree-Rooted Networks – through both their ‘default’ usage and their application to tabular data. Part III compounds the power of the previously covered methods by surveying strategies and techniques to supercharge deep learning systems: autoencoders, deep data generation, meta-optimization, multi-model arrangement, and neural netw | 出版日期 | Book 2023 | 关键词 | Deep Learning; Tabular Data; Machine Learning; Neural Network; Recurrent Neural Networks; Convolutional N | 版次 | 1 | doi | https://doi.org/10.1007/978-1-4842-8692-0 | isbn_softcover | 978-1-4842-8691-3 | isbn_ebook | 978-1-4842-8692-0 | copyright | Andre Ye and Zian Wang 2023 |
The information of publication is updating
|
|