书目名称 | Model-Free Prediction and Regression | 副标题 | A Transformation-Bas | 编辑 | Dimitris N. Politis | 视频video | | 概述 | Develops the model-free prediction principle.Goes beyond fitting models toward direct prediction.Treats applications in regression and autoregression in detail | 丛书名称 | Frontiers in Probability and the Statistical Sciences | 图书封面 | 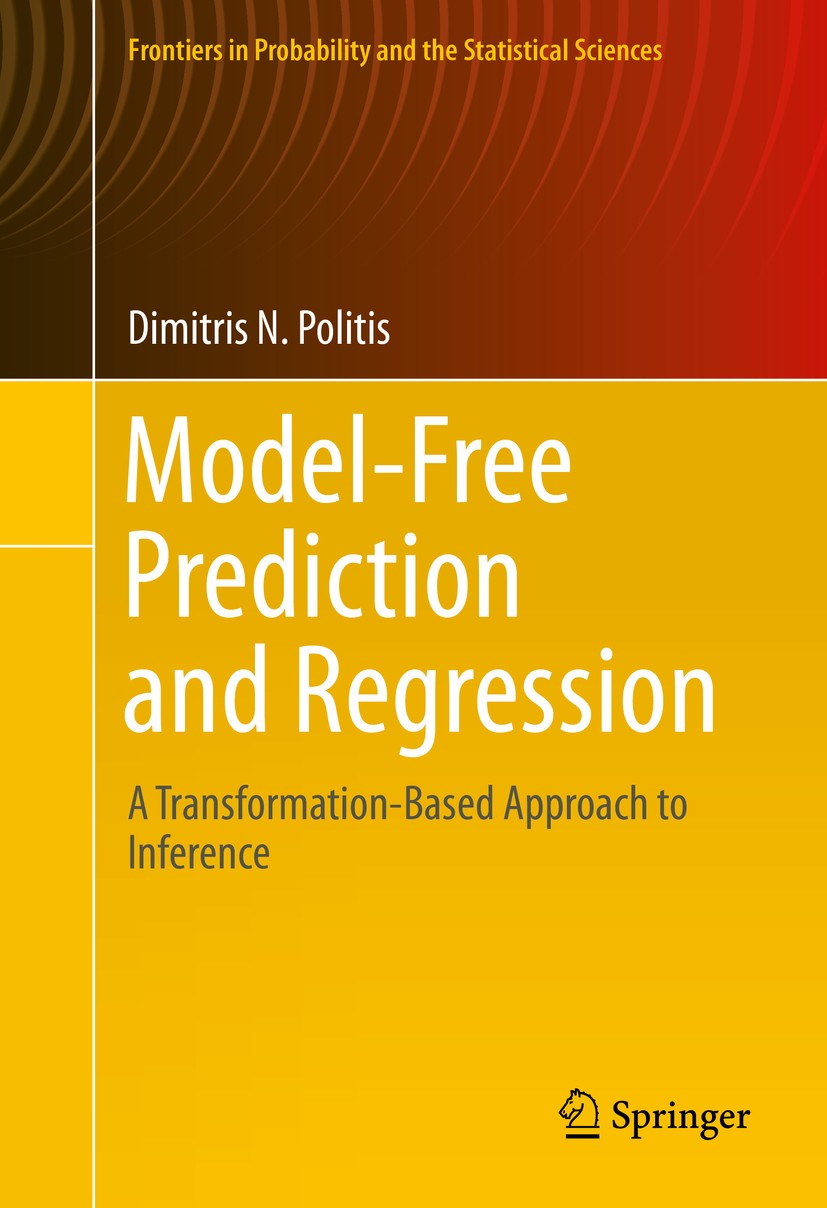 | 描述 | .The Model-Free Prediction Principle expounded upon in this monograph is based on the simple notion of transforming a complex dataset to one that is easier to work with, e.g., i.i.d. or Gaussian. As such, it restores the emphasis on observable quantities, i.e., current and future data, as opposed to unobservable model parameters and estimates thereof, and yields optimal predictors in diverse settings such as regression and time series. Furthermore, the Model-Free Bootstrap takes us beyond point prediction in order to construct frequentist prediction intervals without resort to unrealistic assumptions such as normality..Prediction has been traditionally approached via a model-based paradigm, i.e., (a) fit a model to the data at hand, and (b) use the fitted model to extrapolate/predict future data. Due to both mathematical and computational constraints, 20th century statistical practice focused mostly on parametric models. Fortunately, with the advent of widely accessible powerful computing in the late 1970s, computer-intensive methods such as the bootstrap and cross-validation freed practitioners from the limitations of parametric models, and paved the way towards the `big data‘ era | 出版日期 | Book 2015 | 关键词 | Independent and identically distributed; Markov processes; Modeling; Prediction; Regression; Statistical | 版次 | 1 | doi | https://doi.org/10.1007/978-3-319-21347-7 | isbn_softcover | 978-3-319-35249-7 | isbn_ebook | 978-3-319-21347-7Series ISSN 2624-9987 Series E-ISSN 2624-9995 | issn_series | 2624-9987 | copyright | The Author 2015 |
The information of publication is updating
|
|