书目名称 | Medical Image Computing and Computer Assisted Intervention – MICCAI 2020 | 副标题 | 23rd International C | 编辑 | Anne L. Martel,Purang Abolmaesumi,Leo Joskowicz | 视频video | | 丛书名称 | Lecture Notes in Computer Science | 图书封面 | 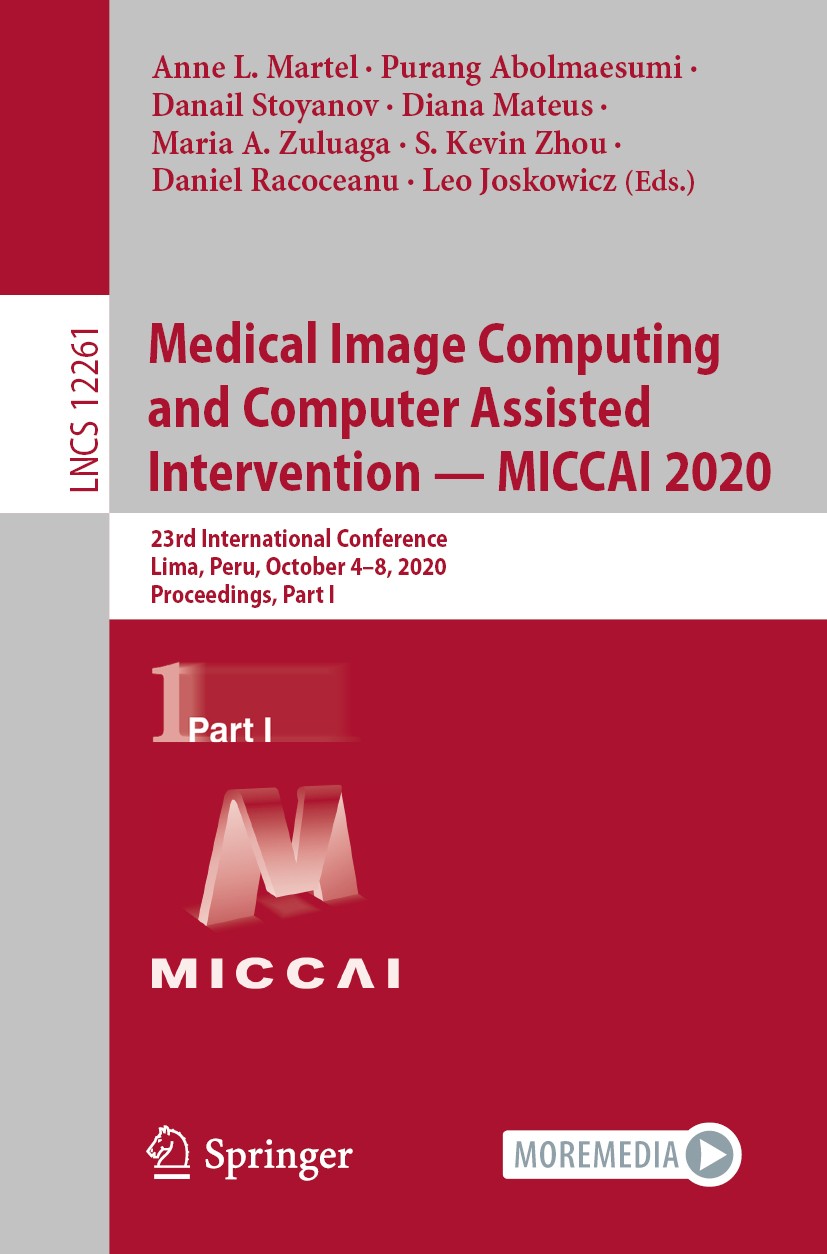 | 描述 | The seven-volume set LNCS 12261, 12262, 12263, 12264, 12265, 12266, and 12267 constitutes the refereed proceedings of the 23rd International Conference on Medical Image Computing and Computer-Assisted Intervention, MICCAI 2020, held in Lima, Peru, in October 2020. The conference was held virtually due to the COVID-19 pandemic..The 542 revised full papers presented were carefully reviewed and selected from 1809 submissions in a double-blind review process. The papers are organized in the following topical sections:..Part I: machine learning methodologies..Part II: image reconstruction; prediction and diagnosis; cross-domain methods and reconstruction; domain adaptation; machine learning applications; generative adversarial networks..Part III: CAI applications; image registration; instrumentation and surgical phase detection; navigation and visualization; ultrasound imaging; video image analysis..Part IV: segmentation; shape models and landmark detection..Part V: biological, optical, microscopic imaging; cell segmentation and stain normalization; histopathology image analysis; opthalmology..Part VI: angiography and vessel analysis; breast imaging; colonoscopy; dermatology; fetal imag | 出版日期 | Conference proceedings 2020 | 关键词 | artificial intelligence; bioinformatics; computer vision; deep learning; image analysis; image processing | 版次 | 1 | doi | https://doi.org/10.1007/978-3-030-59710-8 | isbn_softcover | 978-3-030-59709-2 | isbn_ebook | 978-3-030-59710-8Series ISSN 0302-9743 Series E-ISSN 1611-3349 | issn_series | 0302-9743 | copyright | Springer Nature Switzerland AG 2020 |
The information of publication is updating
|
|