书目名称 | Massively Parallel Evolutionary Computation on GPGPUs | 编辑 | Shigeyoshi Tsutsui,Pierre Collet | 视频video | | 概述 | First book dedicated to exciting new approach.Content characterized by emphasis on solving practical problems.Valuable for researchers, practitioners, and graduate students.Includes supplementary mate | 丛书名称 | Natural Computing Series | 图书封面 | 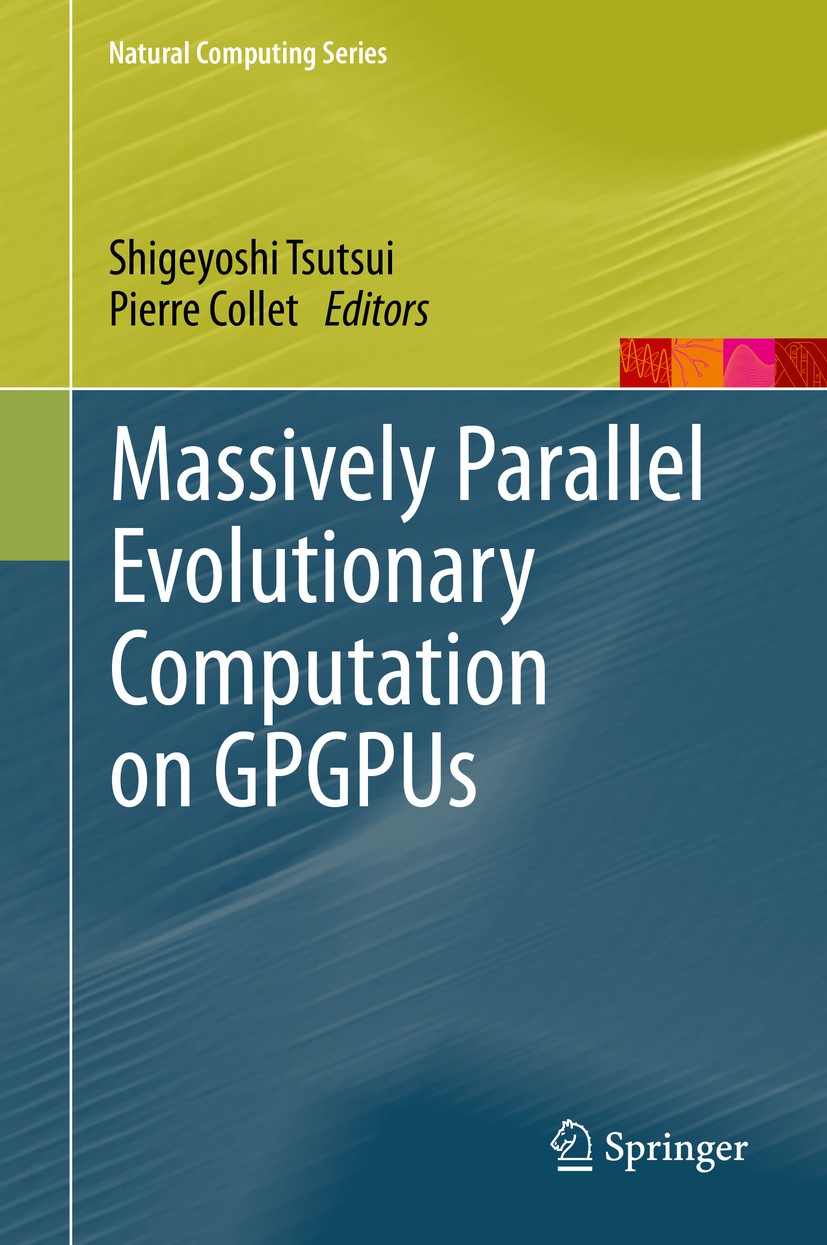 | 描述 | .Evolutionary algorithms (EAs) are metaheuristics that learn from natural collective behavior and are applied to solve optimization problems in domains such as scheduling, engineering, bioinformatics, and finance. Such applications demand acceptable solutions with high-speed execution using finite computational resources. Therefore, there have been many attempts to develop platforms for running parallel EAs using multicore machines, massively parallel cluster machines, or grid computing environments. Recent advances in general-purpose computing on graphics processing units (GPGPU) have opened up this possibility for parallel EAs, and this is the first book dedicated to this exciting development.. .The three chapters of Part I are tutorials, representing a comprehensive introduction to the approach, explaining the characteristics of the hardware used, and presenting a representative project to develop a platform for automatic parallelization of evolutionary computing (EC) on GPGPUs. The 10 chapters in Part II focus on how to consider key EC approaches in the light of this advanced computational technique, in particular addressing generic local search, tabu search, genetic algorithms | 出版日期 | Book 2013 | 关键词 | Artificial chemistries; CGP; Cartesian genetic programming; Clusters; Differential evolution; Evolutionar | 版次 | 1 | doi | https://doi.org/10.1007/978-3-642-37959-8 | isbn_softcover | 978-3-662-51345-3 | isbn_ebook | 978-3-642-37959-8Series ISSN 1619-7127 Series E-ISSN 2627-6461 | issn_series | 1619-7127 | copyright | Springer-Verlag Berlin Heidelberg 2013 |
The information of publication is updating
|
|