书目名称 | Machine Learning: ECML 2007 |
副标题 | 18th European Confer |
编辑 | Joost N. Kok,Jacek Koronacki,Andrzej Skowron |
视频video | |
丛书名称 | Lecture Notes in Computer Science |
图书封面 | 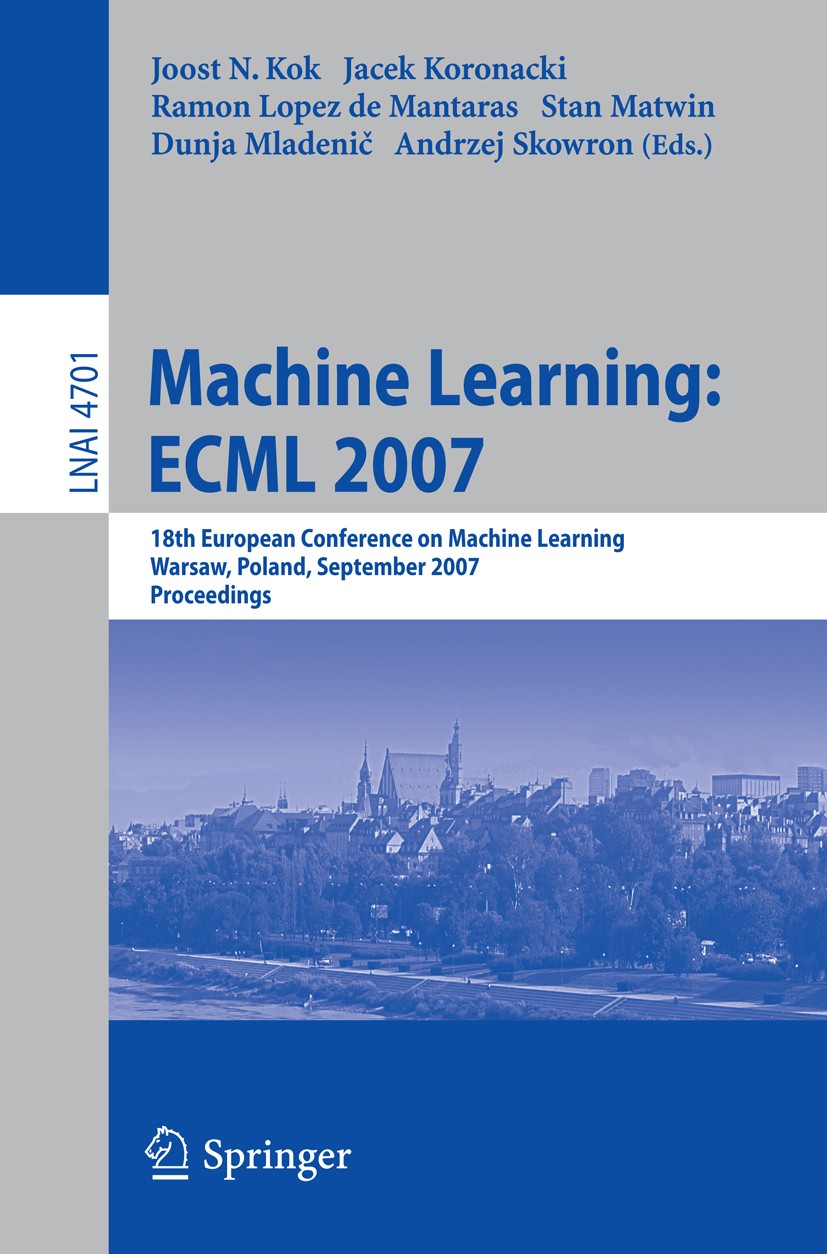 |
描述 | The two premier annual European conferences in the areas of machine learning and data mining have been collocated ever since the ?rst joint conference in Freiburg, 2001. The European Conference on Machine Learning (ECML) traces its origins to 1986, when the ?rst European Working Session on Learning was held in Orsay, France. The European Conference on Principles and Practice of KnowledgeDiscoveryinDatabases(PKDD) was?rstheldin1997inTrondheim, Norway. Over the years, the ECML/PKDD series has evolved into one of the largest and most selective international conferences in machine learning and data mining. In 2007, the seventh collocated ECML/PKDD took place during September 17–21 on the centralcampus of WarsawUniversityand in the nearby Staszic Palace of the Polish Academy of Sciences. The conference for the third time used a hierarchical reviewing process. We nominated 30 Area Chairs, each of them responsible for one sub-?eld or several closely related research topics. Suitable areas were selected on the basis of the submission statistics for ECML/PKDD 2006 and for last year’s International Conference on Machine Learning (ICML 2006) to ensure a proper load balance amongtheAreaChairs. |
出版日期 | Conference proceedings 2007 |
关键词 | active learning; algorithmic learning; algorithms; classifier systems; cognition; genetic programming; ind |
版次 | 1 |
doi | https://doi.org/10.1007/978-3-540-74958-5 |
isbn_softcover | 978-3-540-74957-8 |
isbn_ebook | 978-3-540-74958-5Series ISSN 0302-9743 Series E-ISSN 1611-3349 |
issn_series | 0302-9743 |
copyright | Springer-Verlag Berlin Heidelberg 2007 |