书目名称 | Machine Learning under Malware Attack | 编辑 | Raphael Labaca-Castro | 视频video | | 图书封面 | 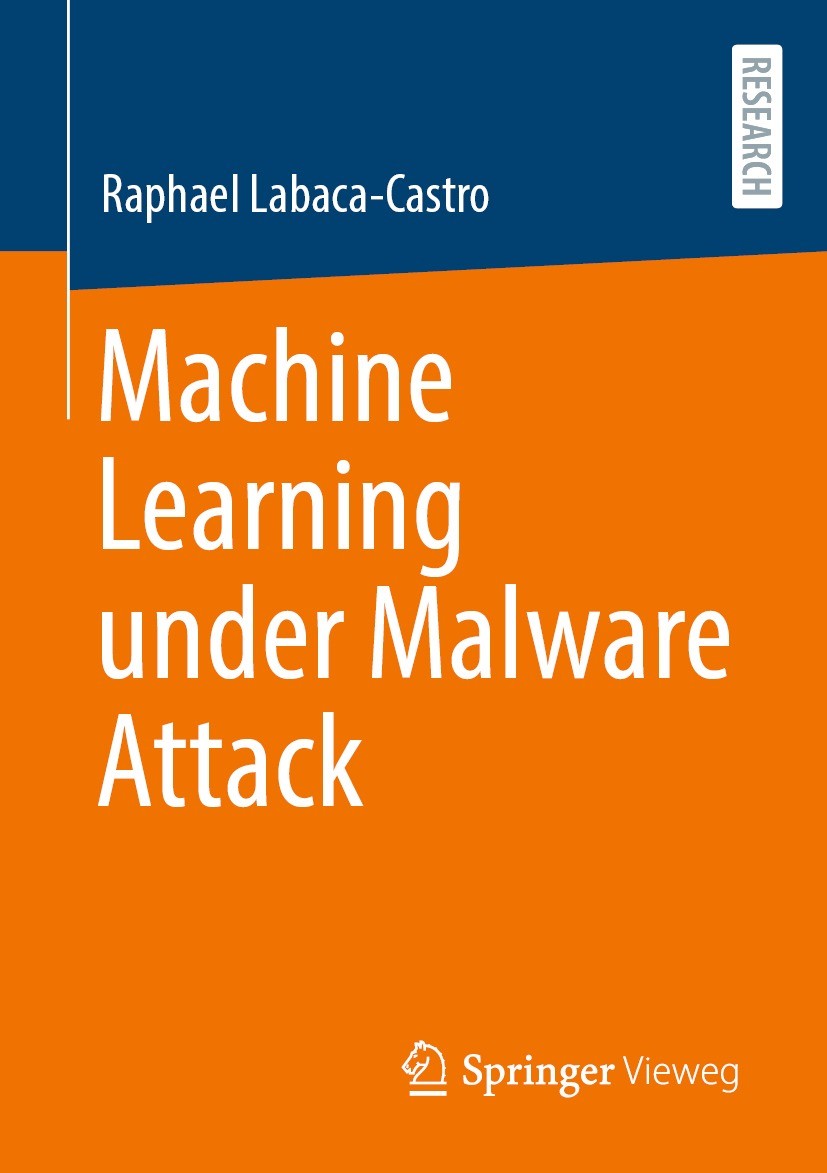 | 描述 | Machine learning has become key in supporting decision-making processes across a wide array of applications, ranging from autonomous vehicles to malware detection. However, while highly accurate, these algorithms have been shown to exhibit vulnerabilities, in which they could be deceived to return preferred predictions. Therefore, carefully crafted adversarial objects may impact the trust of machine learning systems compromising the reliability of their predictions, irrespective of the field in which they are deployed. The goal of this book is to improve the understanding of adversarial attacks, particularly in the malware context, and leverage the knowledge to explore defenses against adaptive adversaries. Furthermore, to study systemic weaknesses that can improve the resilience of machine learning models. | 出版日期 | Book 2023 | 关键词 | Computer Security; Machine Learning; Adversarial ML; Trustworthy AI; Malware; FAME | 版次 | 1 | doi | https://doi.org/10.1007/978-3-658-40442-0 | isbn_softcover | 978-3-658-40441-3 | isbn_ebook | 978-3-658-40442-0 | copyright | The Editor(s) (if applicable) and The Author(s), under exclusive license to Springer Fachmedien Wies |
The information of publication is updating
|
|