书目名称 | Machine Learning in Modeling and Simulation |
副标题 | Methods and Applicat |
编辑 | Timon Rabczuk,Klaus-Jürgen Bathe |
视频video | |
概述 | Comprehensive state-of-the-art book on scientific machine learning approaches in modelling & simulation.Covers the wide range of PDEs, uncertainty, optimization, inverse analysis, constitutive modelli |
丛书名称 | Computational Methods in Engineering & the Sciences |
图书封面 | 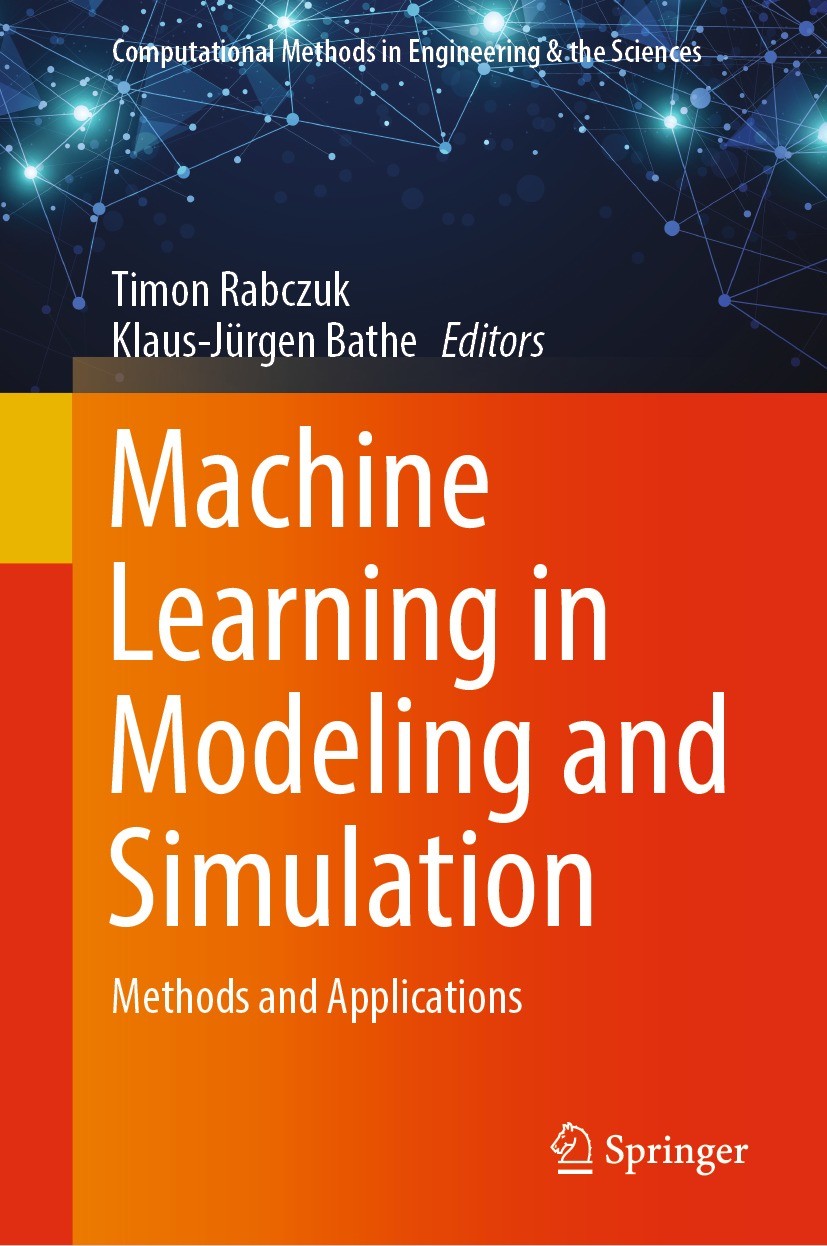 |
描述 | Machine learning (ML) approaches have been extensively and successfully employed in various areas, like in economics, medical predictions, face recognition, credit card fraud detection, and spam filtering. There is clearly also the potential that ML techniques developed in Engineering and the Sciences will drastically increase the possibilities of analysis and accelerate the design to analysis time. With the use of ML techniques, coupled to conventional methods like finite element and digital twin technologies, new avenues of modeling and simulation can be opened but the potential of these ML techniques needs to still be fully harvested, with the methods developed and enhanced. The objective of this book is to provide an overview of ML in Engineering and the Sciences presenting fundamental theoretical ingredients with a focus on the next generation of computer modeling in Engineering and the Sciences in which the exciting aspects of machine learning are incorporated. The book is of value to any researcher and practitioner interested in research or applications of ML in the areas of scientific modeling and computer aided engineering.. |
出版日期 | Book 2023 |
关键词 | Machine Learning; Modeling and Simulation; Computational Mechanics; Computational Materials Science; Opt |
版次 | 1 |
doi | https://doi.org/10.1007/978-3-031-36644-4 |
isbn_softcover | 978-3-031-36646-8 |
isbn_ebook | 978-3-031-36644-4Series ISSN 2662-4869 Series E-ISSN 2662-4877 |
issn_series | 2662-4869 |
copyright | The Editor(s) (if applicable) and The Author(s), under exclusive license to Springer Nature Switzerl |