书目名称 | Machine Learning for Engineers | 副标题 | Using data to solve | 编辑 | Ryan G. McClarren | 视频video | | 概述 | Illustrates concepts with examples and case studies drawn from engineering science.Presents detailed coverage of deep neural networks for practical applications in engineering science.Provides source | 图书封面 | 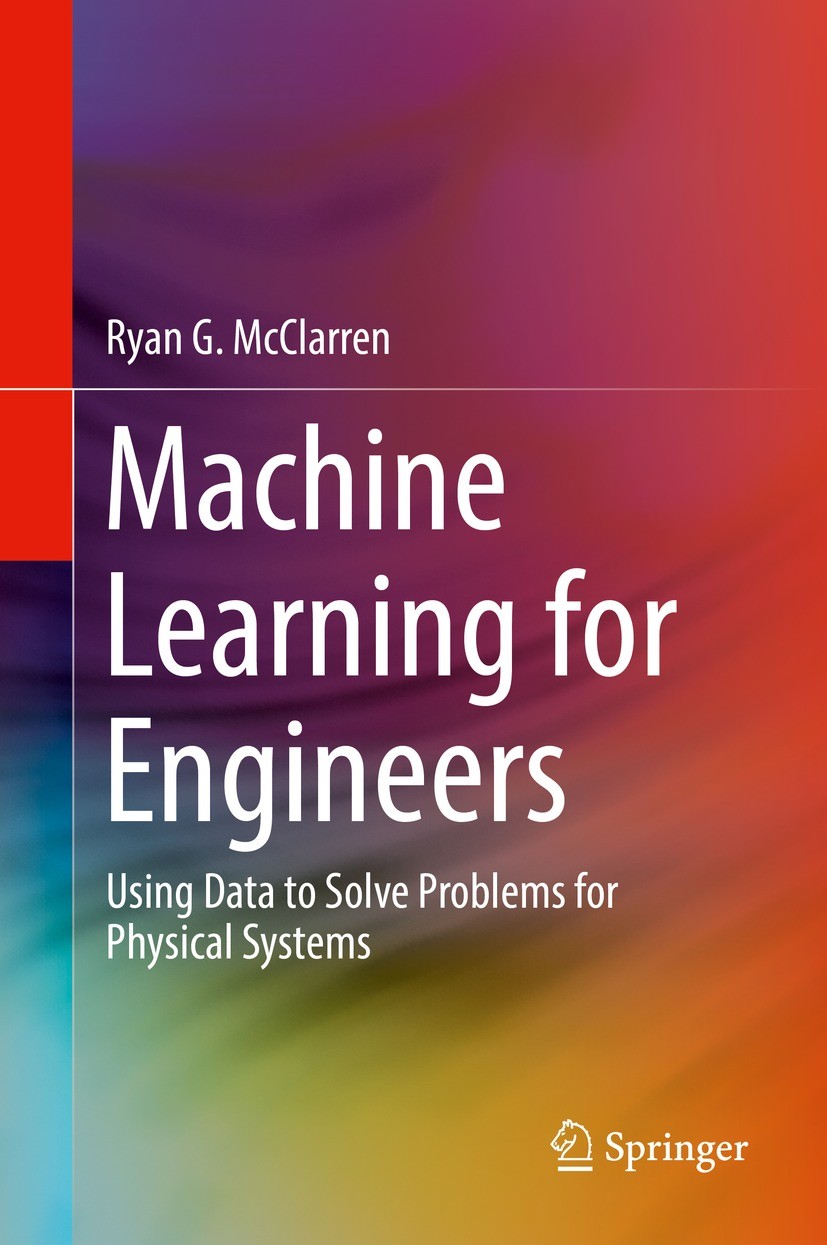 | 描述 | .All engineers and applied scientists will need to harness the power of machine learning to solve the highly complex and data intensive problems now emerging. This text teaches state-of-the-art machine learning technologies to students and practicing engineers from the traditionally “analog” disciplines—mechanical, aerospace, chemical, nuclear, and civil. Dr. McClarren examines these technologies from an engineering perspective and illustrates their specific value to engineers by presenting concrete examples based on physical systems. The book proceeds from basic learning models to deep neural networks, gradually increasing readers’ ability to apply modern machine learning techniques to their current work and to prepare them for future, as yet unknown, problems. Rather than taking a black box approach, the author teaches a broad range of techniques while conveying the kinds of problems best addressed by each. Examples and case studies in controls, dynamics, heat transfer, and other engineering applications are implemented in Python and the libraries scikit-learn and tensorflow, demonstrating how readers can apply the most up-to-date methods to their own problems. The book equally | 出版日期 | Textbook 2021 | 关键词 | supervised learning; unsupervised learning; Bayesian statistics; linear models; tree-based models; deep n | 版次 | 1 | doi | https://doi.org/10.1007/978-3-030-70388-2 | isbn_softcover | 978-3-030-70390-5 | isbn_ebook | 978-3-030-70388-2 | copyright | Springer Nature Switzerland AG 2021 |
The information of publication is updating
|
|