书目名称 | Machine Learning for Ecology and Sustainable Natural Resource Management | 编辑 | Grant Humphries,Dawn R. Magness,Falk Huettmann | 视频video | | 概述 | Shows ecologists cutting-edge methods that can help in understanding complex systems with multiple interacting variablesto and to form predictive hypotheses from large datasets.Provides practical exam | 图书封面 | 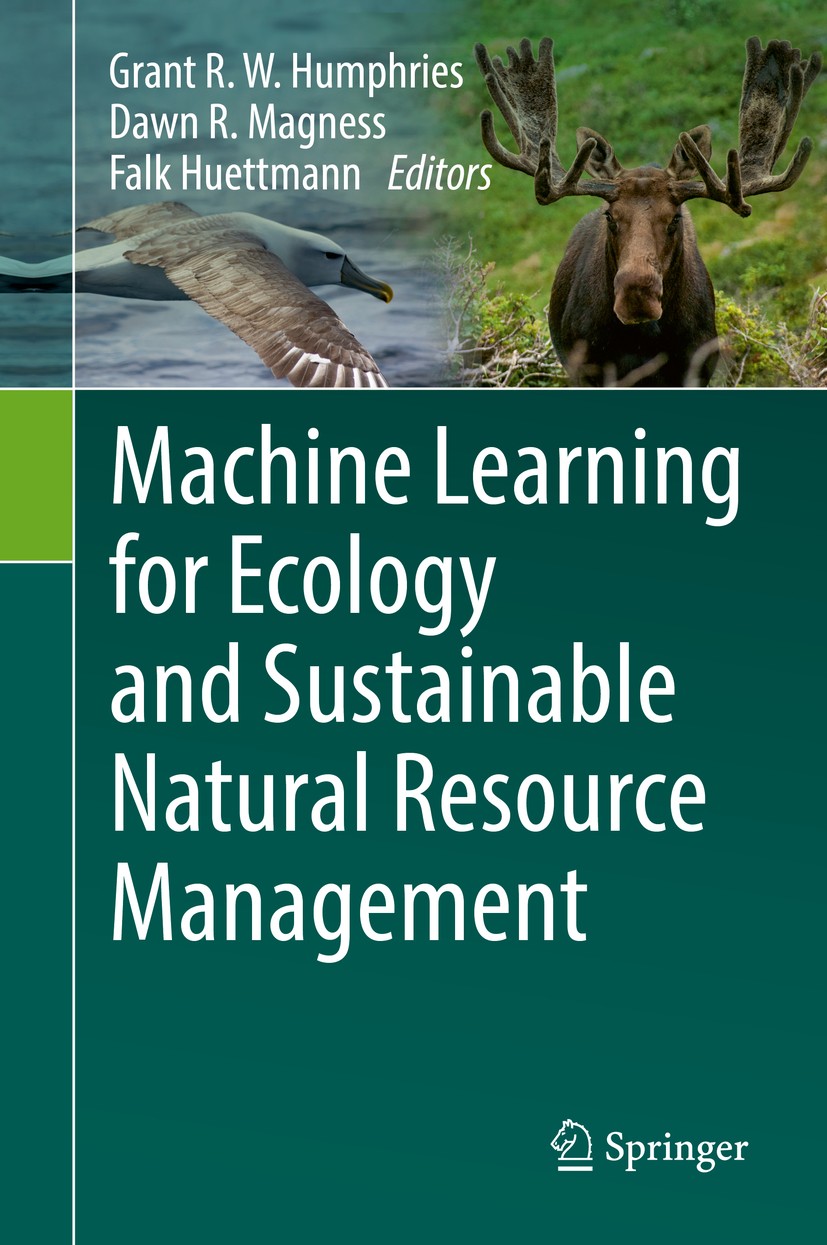 | 描述 | .Ecologists and natural resource managers are charged with making complex management decisions in the face of a rapidly changing environment resulting from climate change, energy development, urban sprawl, invasive species and globalization. Advances in Geographic Information System (GIS) technology, digitization, online data availability, historic legacy datasets, remote sensors and the ability to collect data on animal movements via satellite and GPS have given rise to large, highly complex datasets. These datasets could be utilized for making critical management decisions, but are often “messy” and difficult to interpret. Basic artificial intelligence algorithms (i.e., machine learning) are powerful tools that are shaping the world and must be taken advantage of in the life sciences. In ecology, machine learning algorithms are critical to helping resource managers synthesize information to better understand complex ecological systems. Machine Learning has a wide variety of powerful applications, with three general uses that are of particular interest to ecologists: (1) data exploration to gain system knowledge and generate new hypotheses, (2) predicting ecological patterns in sp | 出版日期 | Book 2018 | 关键词 | Quantitative ecology; artificial intelligence; Statistics; data mining; machine learning; Wildlife biolog | 版次 | 1 | doi | https://doi.org/10.1007/978-3-319-96978-7 | isbn_ebook | 978-3-319-96978-7 | copyright | Springer Nature Switzerland AG 2018 |
The information of publication is updating
|
|