书目名称 | Machine Learning and Knowledge Discovery in Databases: Research Track |
副标题 | European Conference, |
编辑 | Danai Koutra,Claudia Plant,Francesco Bonchi |
视频video | |
丛书名称 | Lecture Notes in Computer Science |
图书封面 | 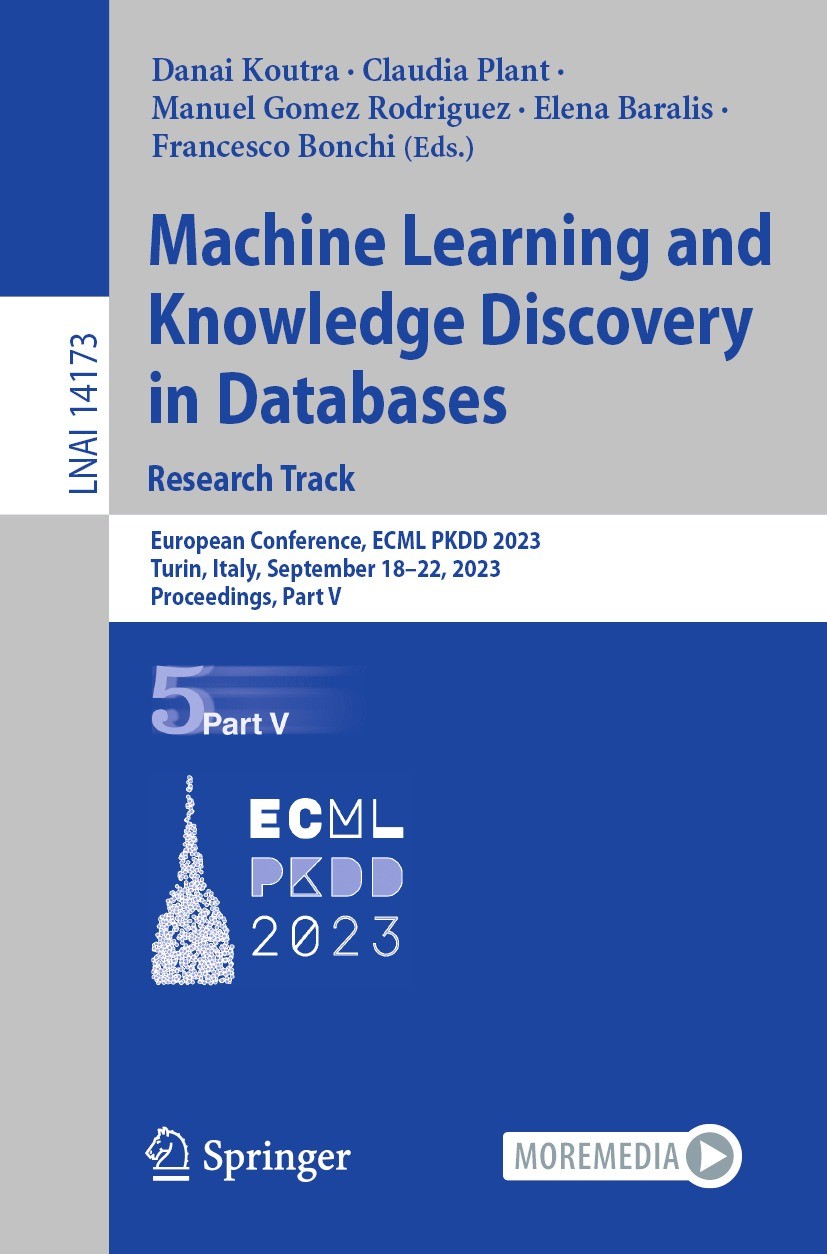 |
描述 | The multi-volume set LNAI 14169 until 14175 constitutes the refereed proceedings of the European Conference on Machine Learning and Knowledge Discovery in Databases, ECML PKDD 2023, which took place in Turin, Italy, in September 2023..The 196 papers were selected from the 829 submissions for the Research Track, and 58 papers were selected from the 239 submissions for the Applied Data Science Track. .The volumes are organized in topical sections as follows:.Part I:. Active Learning; Adversarial Machine Learning; Anomaly Detection; Applications; Bayesian Methods; Causality; Clustering..Part II: .Computer Vision; Deep Learning; Fairness; Federated Learning; Few-shot learning; Generative Models; Graph Contrastive Learning..Part III: .Graph Neural Networks; Graphs; Interpretability; Knowledge Graphs; Large-scale Learning..Part IV:. Natural Language Processing; Neuro/Symbolic Learning; Optimization; Recommender Systems; Reinforcement Learning; Representation Learning..Part V:. Robustness; Time Series; Transfer and Multitask Learning..Part VI:. Applied Machine Learning; Computational Social Sciences; Finance; Hardware and Systems; Healthcare & Bioinformatics; Human-Computer Intera |
出版日期 | Conference proceedings 2023 |
关键词 | artificial intelligence; computer hardware; computer networks; computer security; computer systems; compu |
版次 | 1 |
doi | https://doi.org/10.1007/978-3-031-43424-2 |
isbn_softcover | 978-3-031-43423-5 |
isbn_ebook | 978-3-031-43424-2Series ISSN 0302-9743 Series E-ISSN 1611-3349 |
issn_series | 0302-9743 |
copyright | The Editor(s) (if applicable) and The Author(s), under exclusive license to Springer Nature Switzerl |