书目名称 | Machine Learning and Knowledge Discovery in Databases, Part II | 副标题 | European Conference, | 编辑 | Dimitrios Gunopulos,Thomas Hofmann,Michalis Vazirg | 视频video | | 概述 | Fast-track conference proceedings.State-of-the-art research.Up-to-date results | 丛书名称 | Lecture Notes in Computer Science | 图书封面 | 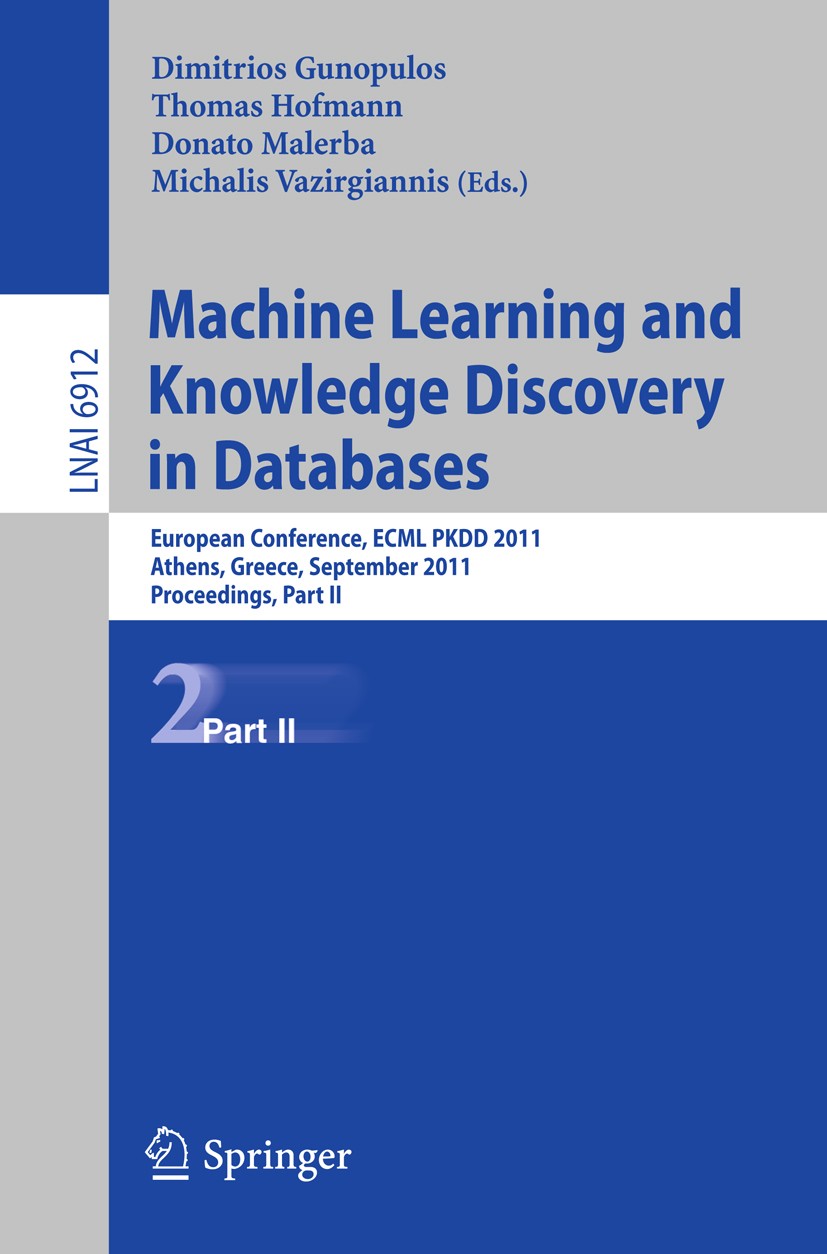 | 描述 | This three-volume set LNAI 6911, LNAI 6912, and LNAI 6913 constitutes the refereed proceedings of the European conference on Machine Learning and Knowledge Discovery in Databases: ECML PKDD 2011, held in Athens, Greece, in September 2011.The 121 revised full papers presented together with 10 invited talks and 11 demos in the three volumes, were carefully reviewed and selected from about 600 paper submissions. The papers address all areas related to machine learning and knowledge discovery in databases as well as other innovative application domains such as supervised and unsupervised learning with some innovative contributions in fundamental issues; dimensionality reduction, distance and similarity learning, model learning and matrix/tensor analysis; graph mining, graphical models, hidden markov models, kernel methods, active and ensemble learning, semi-supervised and transductive learning, mining sparse representations, model learning, inductive logic programming, and statistical learning. a significant part of the papers covers novel and timely applications of data mining and machine learning in industrial domains. | 出版日期 | Conference proceedings 2011 | 关键词 | decision theory; high-dimensional clustering; natural language processing; recommender systems; self-org | 版次 | 1 | doi | https://doi.org/10.1007/978-3-642-23783-6 | isbn_softcover | 978-3-642-23782-9 | isbn_ebook | 978-3-642-23783-6Series ISSN 0302-9743 Series E-ISSN 1611-3349 | issn_series | 0302-9743 | copyright | Springer-Verlag GmbH Berlin Heidelberg 2011 |
The information of publication is updating
书目名称Machine Learning and Knowledge Discovery in Databases, Part II影响因子(影响力) 
书目名称Machine Learning and Knowledge Discovery in Databases, Part II影响因子(影响力)学科排名 
书目名称Machine Learning and Knowledge Discovery in Databases, Part II网络公开度 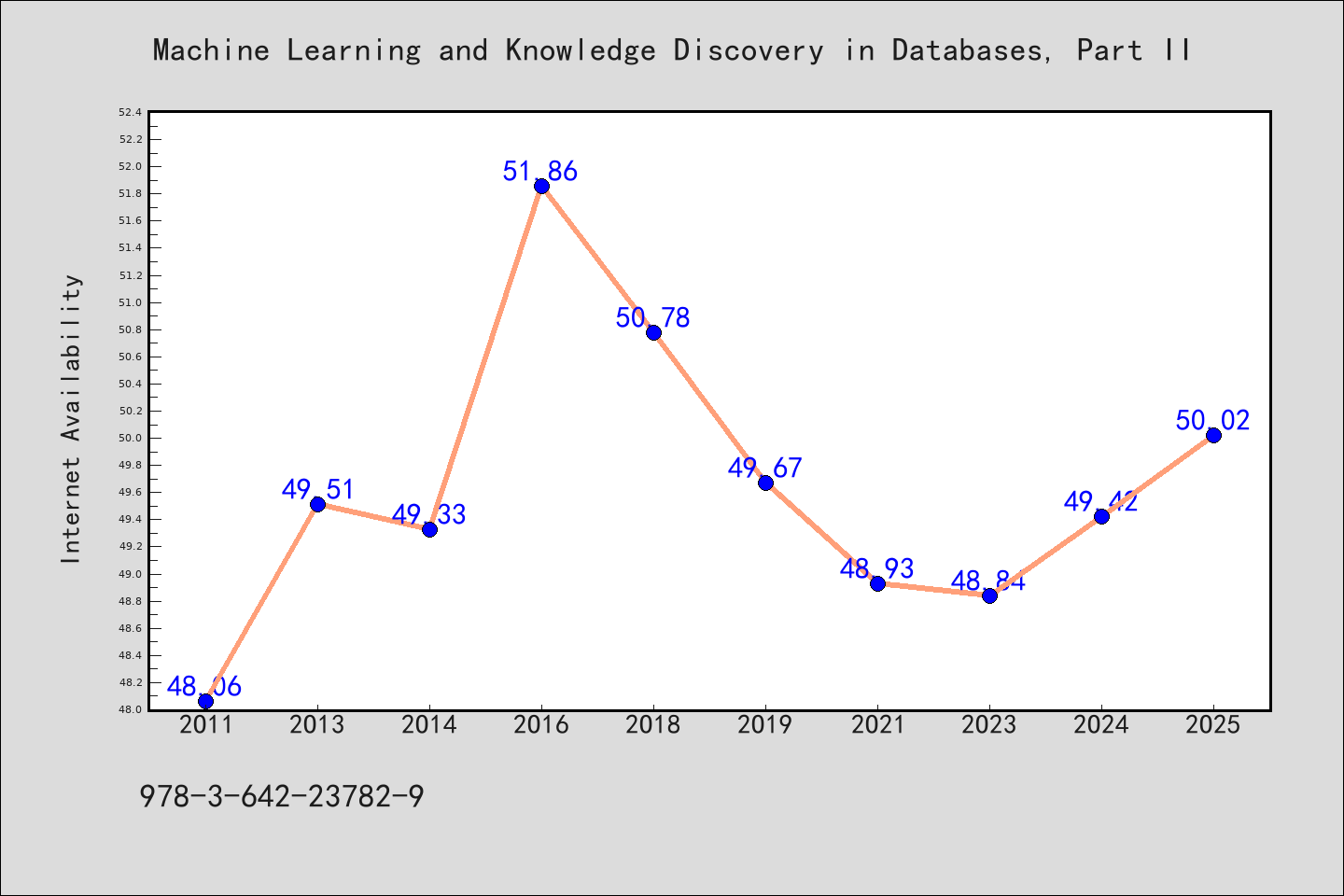
书目名称Machine Learning and Knowledge Discovery in Databases, Part II网络公开度学科排名 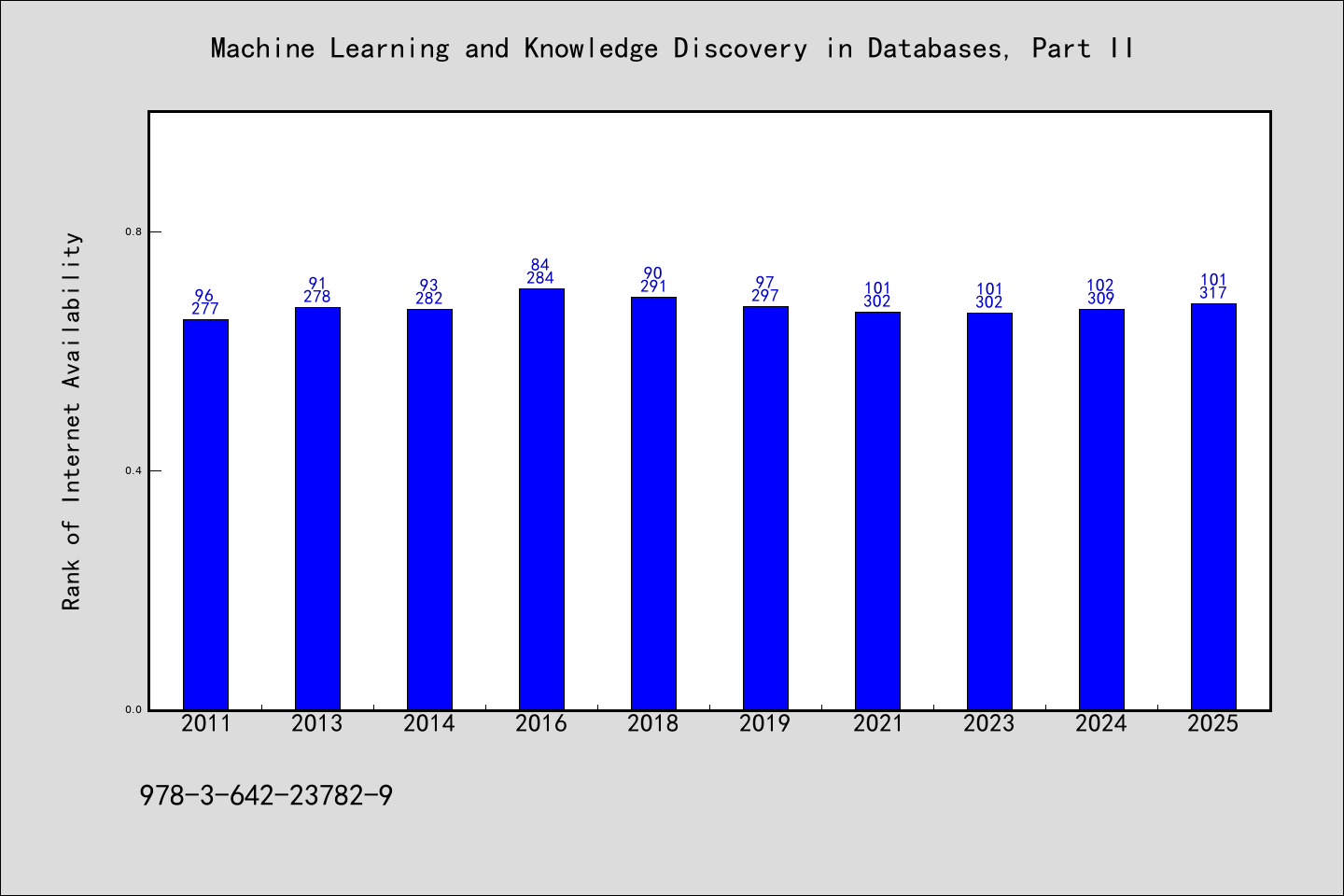
书目名称Machine Learning and Knowledge Discovery in Databases, Part II被引频次 
书目名称Machine Learning and Knowledge Discovery in Databases, Part II被引频次学科排名 
书目名称Machine Learning and Knowledge Discovery in Databases, Part II年度引用 
书目名称Machine Learning and Knowledge Discovery in Databases, Part II年度引用学科排名 
书目名称Machine Learning and Knowledge Discovery in Databases, Part II读者反馈 
书目名称Machine Learning and Knowledge Discovery in Databases, Part II读者反馈学科排名 
|
|
|