书目名称 | Machine Learning and Knowledge Discovery in Databases | 副标题 | European Conference, | 编辑 | Frank Hutter,Kristian Kersting,Isabel Valera | 视频video | | 丛书名称 | Lecture Notes in Computer Science | 图书封面 | 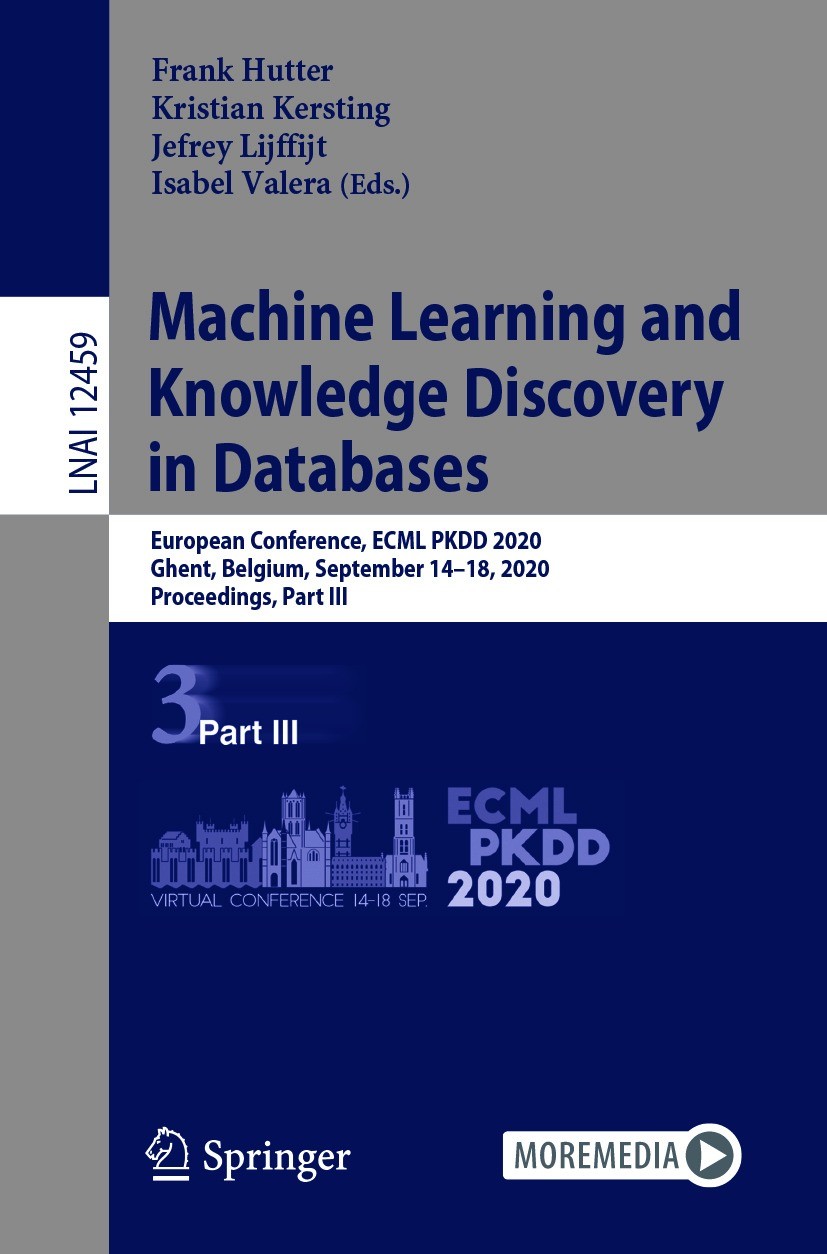 | 描述 | The 5-volume proceedings, LNAI 12457 until 12461 constitutes the refereed proceedings of the European Conference on Machine Learning and Knowledge Discovery in Databases, ECML PKDD 2020, which was held during September 14-18, 2020. The conference was planned to take place in Ghent, Belgium, but had to change to an online format due to the COVID-19 pandemic..The 232 full papers and 10 demo papers presented in this volume were carefully reviewed and selected for inclusion in the proceedings. ..The volumes are organized in topical sections as follows:..Part I:. Pattern Mining; clustering; privacy and fairness; (social) network analysis and computational social science; dimensionality reduction and autoencoders; domain adaptation; sketching, sampling, and binary projections; graphical models and causality; (spatio-) temporal data and recurrent neural networks; collaborative filtering and matrix completion...Part II:. deep learning optimization and theory;active learning; adversarial learning; federated learning; Kernel methods and online learning; partial label learning; reinforcement learning; transfer and multi-task learning; Bayesian optimization and few-shot learning...Part III: .C | 出版日期 | Conference proceedings 2021 | 关键词 | artificial intelligence; classification methods; computer vision; data mining; graph theory; Human-Comput | 版次 | 1 | doi | https://doi.org/10.1007/978-3-030-67664-3 | isbn_softcover | 978-3-030-67663-6 | isbn_ebook | 978-3-030-67664-3Series ISSN 0302-9743 Series E-ISSN 1611-3349 | issn_series | 0302-9743 | copyright | Springer Nature Switzerland AG 2021 |
The information of publication is updating
|
|