书目名称 | Machine Learning and Knowledge Discovery in Databases | 副标题 | European Conference, | 编辑 | Walter Daelemans,Bart Goethals,Katharina Morik | 视频video | | 丛书名称 | Lecture Notes in Computer Science | 图书封面 | 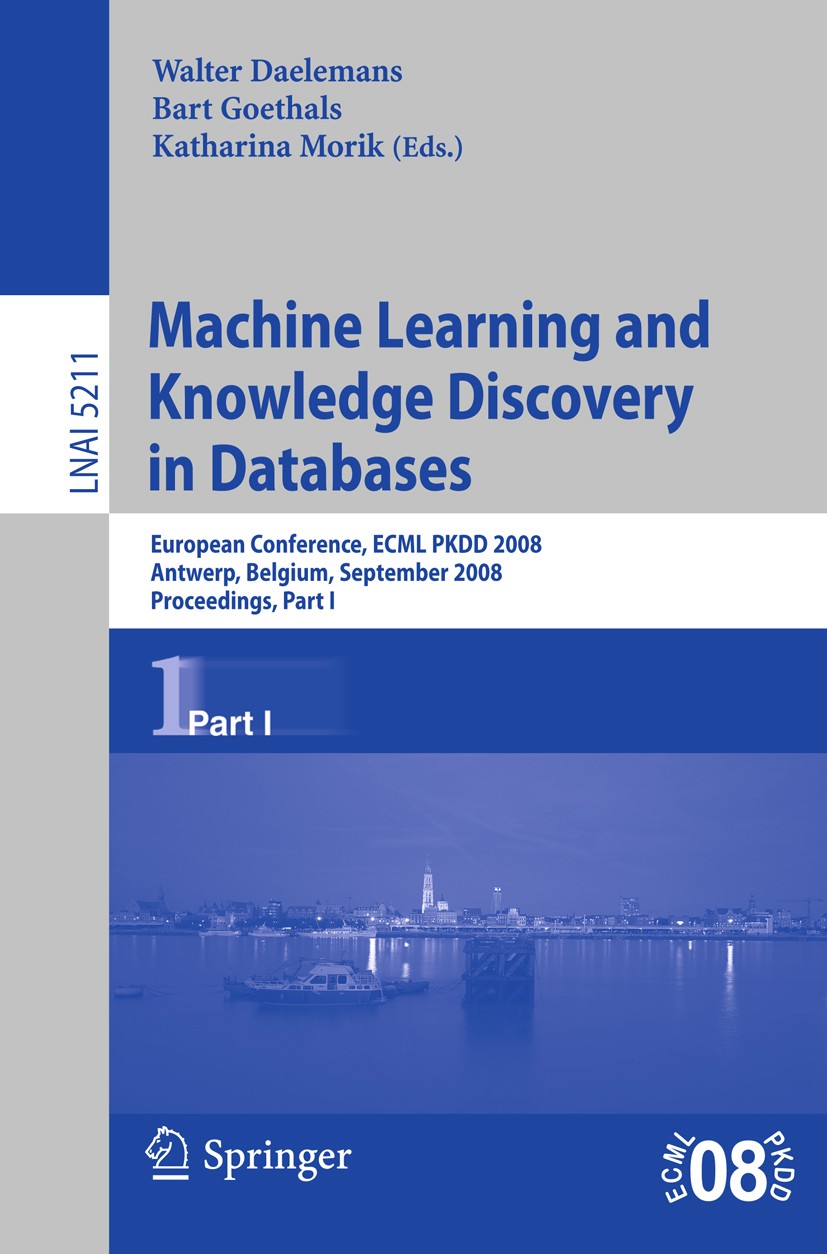 | 描述 | This book constitutes the refereed proceedings of the joint conference on Machine Learning and Knowledge Discovery in Databases: ECML PKDD 2008, held in Antwerp, Belgium, in September 2008. The 100 papers presented in two volumes, together with 5 invited talks, were carefully reviewed and selected from 521 submissions. In addition to the regular papers the volume contains 14 abstracts of papers appearing in full version in the Machine Learning Journal and the Knowledge Discovery and Databases Journal of Springer. The conference intends to provide an international forum for the discussion of the latest high quality research results in all areas related to machine learning and knowledge discovery in databases. The topics addressed are application of machine learning and data mining methods to real-world problems, particularly exploratory research that describes novel learning and mining tasks and applications requiring non-standard techniques. | 出版日期 | Conference proceedings 2008 | 关键词 | Averaging; Support Vector Machine; active learning; algorithmic learning; association rule mining; bayesi | 版次 | 1 | doi | https://doi.org/10.1007/978-3-540-87479-9 | isbn_softcover | 978-3-540-87478-2 | isbn_ebook | 978-3-540-87479-9Series ISSN 0302-9743 Series E-ISSN 1611-3349 | issn_series | 0302-9743 | copyright | Springer-Verlag Berlin Heidelberg 2008 |
The information of publication is updating
|
|