书目名称 | Machine Learning and Data Mining in Pattern Recognition |
副标题 | 4th International Co |
编辑 | Petra Perner,Atsushi Imiya |
视频video | |
丛书名称 | Lecture Notes in Computer Science |
图书封面 | 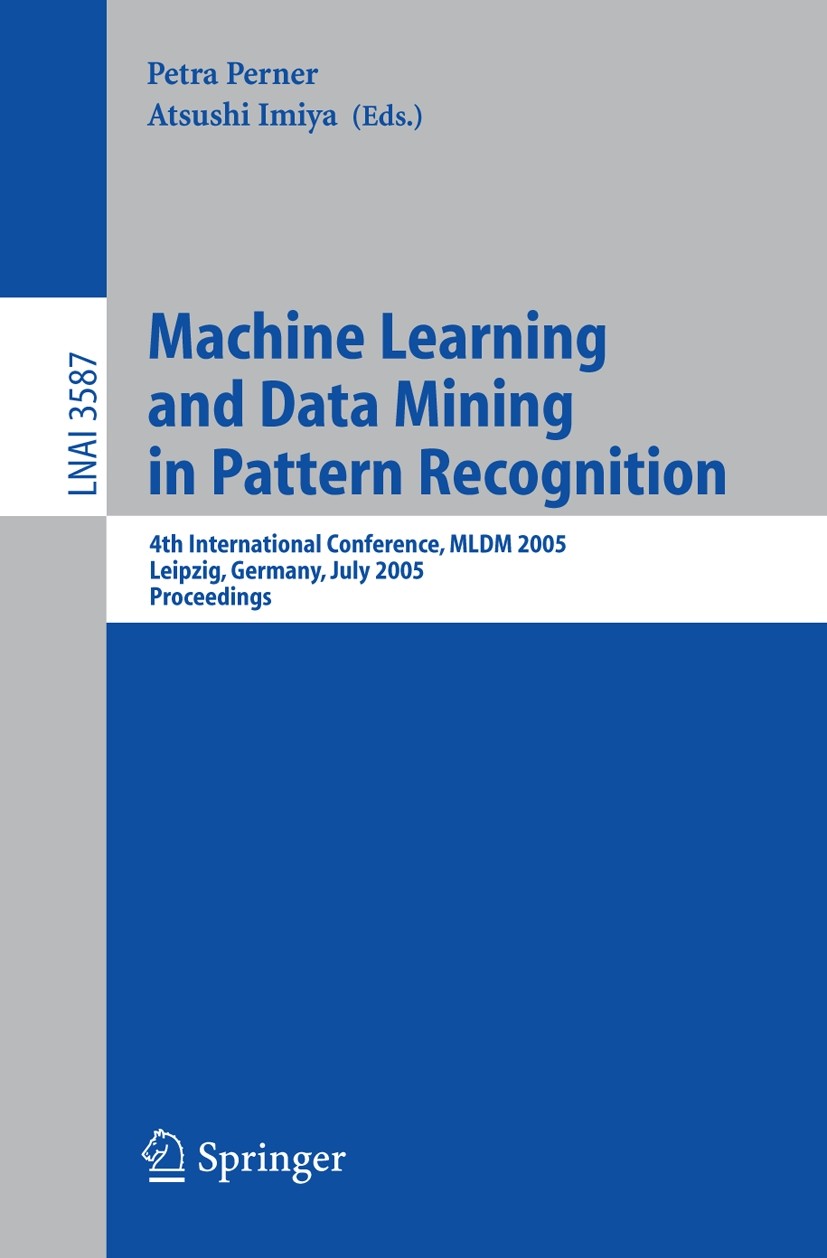 |
描述 | We met again in front of the statue of Gottfried Wilhelm von Leibniz in the city of Leipzig. Leibniz, a famous son of Leipzig, planned automatic logical inference using symbolic computation, aimed to collate all human knowledge. Today, artificial intelligence deals with large amounts of data and knowledge and finds new information using machine learning and data mining. Machine learning and data mining are irreplaceable subjects and tools for the theory of pattern recognition and in applications of pattern recognition such as bioinformatics and data retrieval. This was the fourth edition of MLDM in Pattern Recognition which is the main event of Technical Committee 17 of the International Association for Pattern Recognition; it started out as a workshop and continued as a conference in 2003. Today, there are many international meetings which are titled “machine learning” and “data mining”, whose topics are text mining, knowledge discovery, and applications. This meeting from the first focused on aspects of machine learning and data mining in pattern recognition problems. We planned to reorganize classical and well-established pattern recognition paradigms from the viewpoints of mach |
出版日期 | Conference proceedings 2005 |
关键词 | classification; computer vision; data mining; learning; machine learning; pattern mining |
版次 | 1 |
doi | https://doi.org/10.1007/b138149 |
isbn_softcover | 978-3-540-26923-6 |
isbn_ebook | 978-3-540-31891-0Series ISSN 0302-9743 Series E-ISSN 1611-3349 |
issn_series | 0302-9743 |
copyright | Springer-Verlag Berlin Heidelberg 2005 |