书目名称 | Machine Learning Safety | 编辑 | Xiaowei Huang,Gaojie Jin,Wenjie Ruan | 视频video | | 概述 | Provides a comprehensive and thorough investigation on safety concerns regarding machine learning.Shows readers to identify vulnerabilities in machine learning models and to improve the models in the | 丛书名称 | Artificial Intelligence: Foundations, Theory, and Algorithms | 图书封面 | 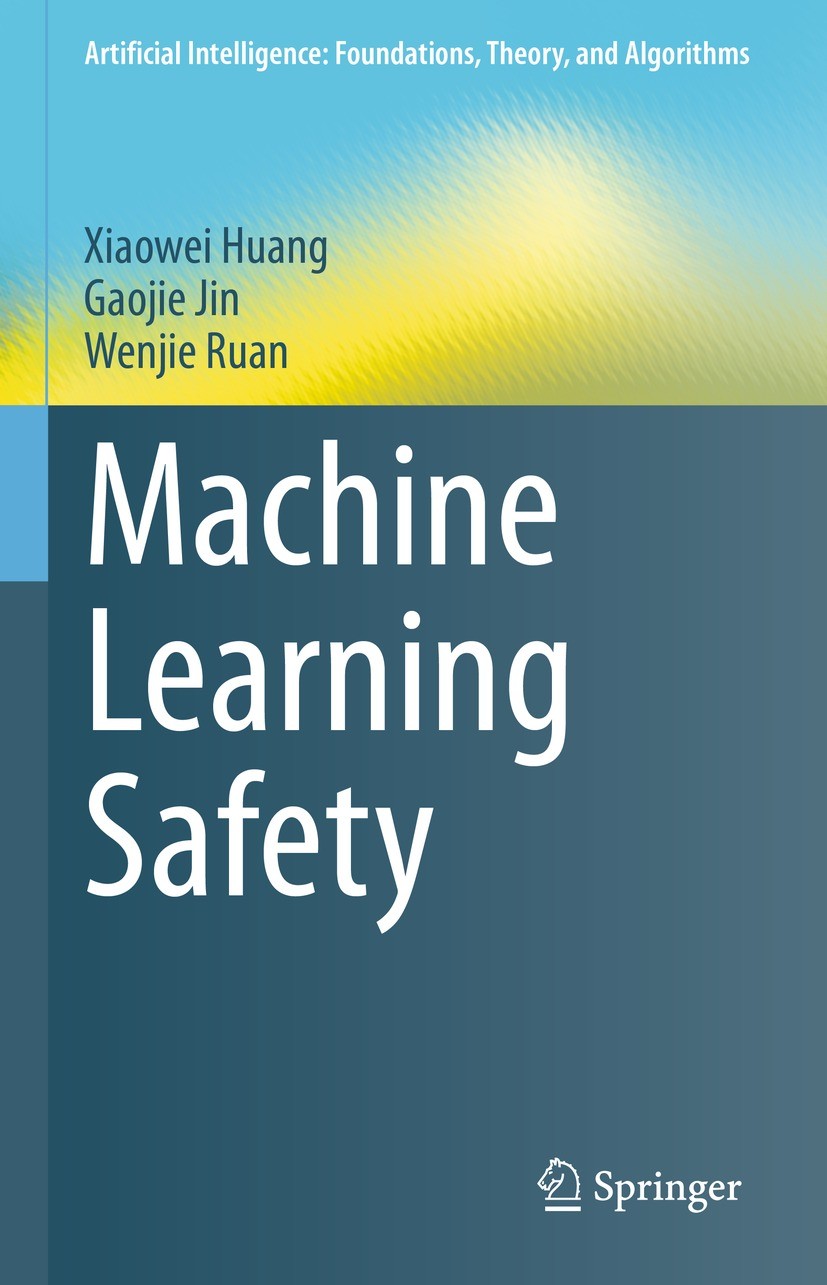 | 描述 | Machine learning algorithms allow computers to learn without being explicitly programmed. Their application is now spreading to highly sophisticated tasks across multiple domains, such as medical diagnostics or fully autonomous vehicles. While this development holds great potential, it also raises new safety concerns, as machine learning has many specificities that make its behaviour prediction and assessment very different from that for explicitly programmed software systems. This book addresses the main safety concerns with regard to machine learning, including its susceptibility to environmental noise and adversarial attacks. Such vulnerabilities have become a major roadblock to the deployment of machine learning in safety-critical applications. The book presents up-to-date techniques for adversarial attacks, which are used to assess the vulnerabilities of machine learning models; formal verification, which is used to determine if a trained machine learning model is free of vulnerabilities; and adversarial training, which is used to enhance the training process and reduce vulnerabilities.. The book aims to improve readers’ awareness of the potential safety issues regarding machi | 出版日期 | Textbook 2023 | 关键词 | Deep Learning; Machine Learning; Safety; Reliability; Robustness | 版次 | 1 | doi | https://doi.org/10.1007/978-981-19-6814-3 | isbn_softcover | 978-981-19-6816-7 | isbn_ebook | 978-981-19-6814-3Series ISSN 2365-3051 Series E-ISSN 2365-306X | issn_series | 2365-3051 | copyright | The Editor(s) (if applicable) and The Author(s), under exclusive license to Springer Nature Singapor |
The information of publication is updating
|
|