书目名称 | Machine Learning Meets Medical Imaging |
副标题 | First International |
编辑 | Kanwal Bhatia,Herve Lombaert |
视频video | |
概述 | Includes supplementary material: |
丛书名称 | Lecture Notes in Computer Science |
图书封面 | 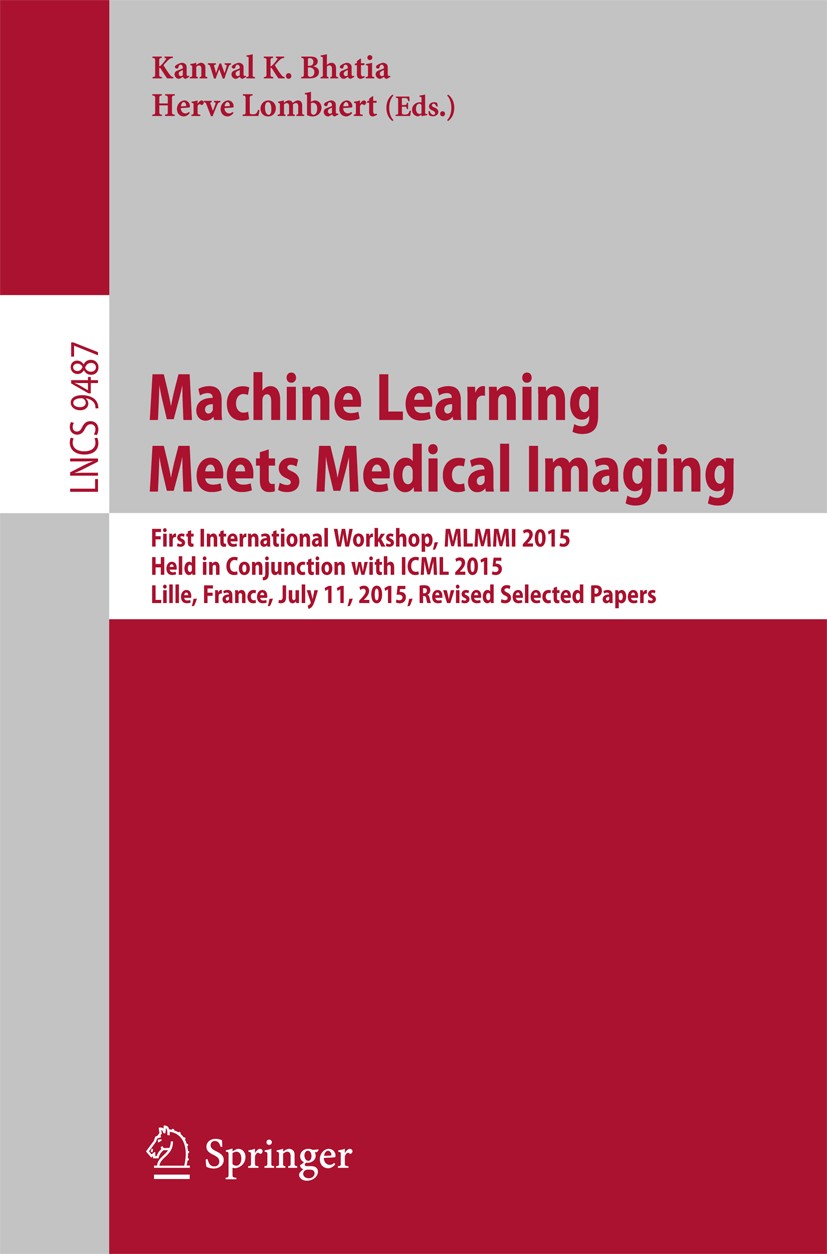 |
描述 | . Normal0falsefalsefalseEN-USX-NONEX-NONE . /* Style Definitions */ table.MsoNormalTable {mso-style-name:"Table Normal"; mso-tstyle-rowband-size:0; mso-tstyle-colband-size:0; mso-style-noshow:yes; mso-style-priority:99; mso-style-qformat:yes; mso-style-parent:""; mso-padding-alt:0in 5.4pt 0in 5.4pt; mso-para-margin:0in; mso-para-margin-bottom:.0001pt; mso-pagination:widow-orphan; font-size:11.0pt; font-family:"Calibri","sans-serif"; mso-ascii-font-family:Calibri; mso-ascii-theme-font:minor-latin; mso-fareast-font-family:"Times New Roman"; mso-fareast-theme-font:minor-fareast; mso-hansi-font-family:Calibri; mso-hansi-theme-font:minor-latin; mso-bidi-font-family:"Times New Roman"; mso-bidi-theme-font:minor-bidi;}This book constitutes the revised selected papers of theFirst International Workshop on Machine Learning in Medical Imaging, MLMMI2015, held in July 2015 in Lille, France, in conjunction with the 32ndInternational Conference on Machine Learning, ICML 2015...The 10 papers presented in this volume were carefullyreviewed and selected for inclusion in the book. The papers communicate thespecific needs and nuances of medical imaging to the machine learning communitywhile exposing |
出版日期 | Conference proceedings 2015 |
关键词 | bioinformatics; computational biology; computer vision; machine learning; mathematical analysis; Alzheime |
版次 | 1 |
doi | https://doi.org/10.1007/978-3-319-27929-9 |
isbn_softcover | 978-3-319-27928-2 |
isbn_ebook | 978-3-319-27929-9Series ISSN 0302-9743 Series E-ISSN 1611-3349 |
issn_series | 0302-9743 |
copyright | Springer International Publishing Switzerland 2015 |