书目名称 | Machine Learning Assisted Evolutionary Multi- and Many- Objective Optimization | 编辑 | Dhish Kumar Saxena,Sukrit Mittal,Erik D. Goodman | 视频video | | 概述 | Dedicated to machine learning based performance enhancements in evolutionary multi- and many objective optimization.Discusses the topics in a clear and structured manner, covering the search, post-opt | 丛书名称 | Genetic and Evolutionary Computation | 图书封面 | 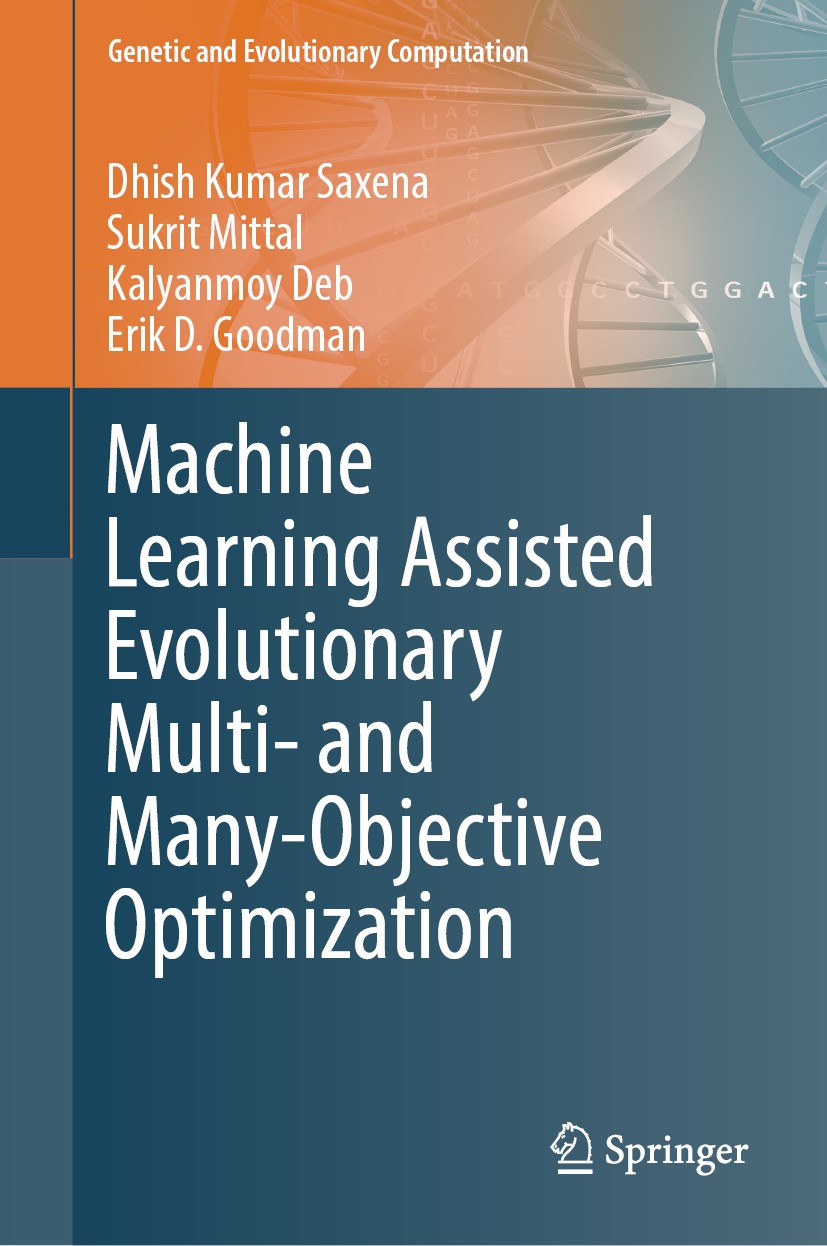 | 描述 | This book focuses on machine learning (ML) assisted evolutionary multi- and many-objective optimization (EMâO). EMâO algorithms, namely EMâOAs, iteratively evolve a set of solutions towards a good Pareto Front approximation. The availability of multiple solution sets over successive generations makes EMâOAs amenable to application of ML for different pursuits. .Recognizing the immense potential for ML-based enhancements in the EMâO domain, this book intends to serve as an exclusive resource for both domain novices and the experienced researchers and practitioners. To achieve this goal, the book first covers the foundations of optimization, including problem and algorithm types. Then, well-structured chapters present some of the key studies on ML-based enhancements in the EMâO domain, systematically addressing important aspects. These include learning to understand the problem structure, converge better, diversify better, simultaneously converge and diversify better, and analyze the Pareto Front. In doing so, this book broadly summarizes the literature, beginning with foundational work on innovization (2003) and objective reduction (2006), and extending to the most recently proposed | 出版日期 | Book 2024 | 关键词 | Evolutionary Multi-objective Optimization; Machine Learning; Evolutionary Computation; Convergence; Dive | 版次 | 1 | doi | https://doi.org/10.1007/978-981-99-2096-9 | isbn_softcover | 978-981-99-2098-3 | isbn_ebook | 978-981-99-2096-9Series ISSN 1932-0167 Series E-ISSN 1932-0175 | issn_series | 1932-0167 | copyright | The Editor(s) (if applicable) and The Author(s), under exclusive license to Springer Nature Singapor |
The information of publication is updating
|
|