书目名称 | Machine Learning Approaches to Non-Intrusive Load Monitoring | 编辑 | Roberto Bonfigli,Stefano Squartini | 视频video | | 丛书名称 | SpringerBriefs in Energy | 图书封面 | 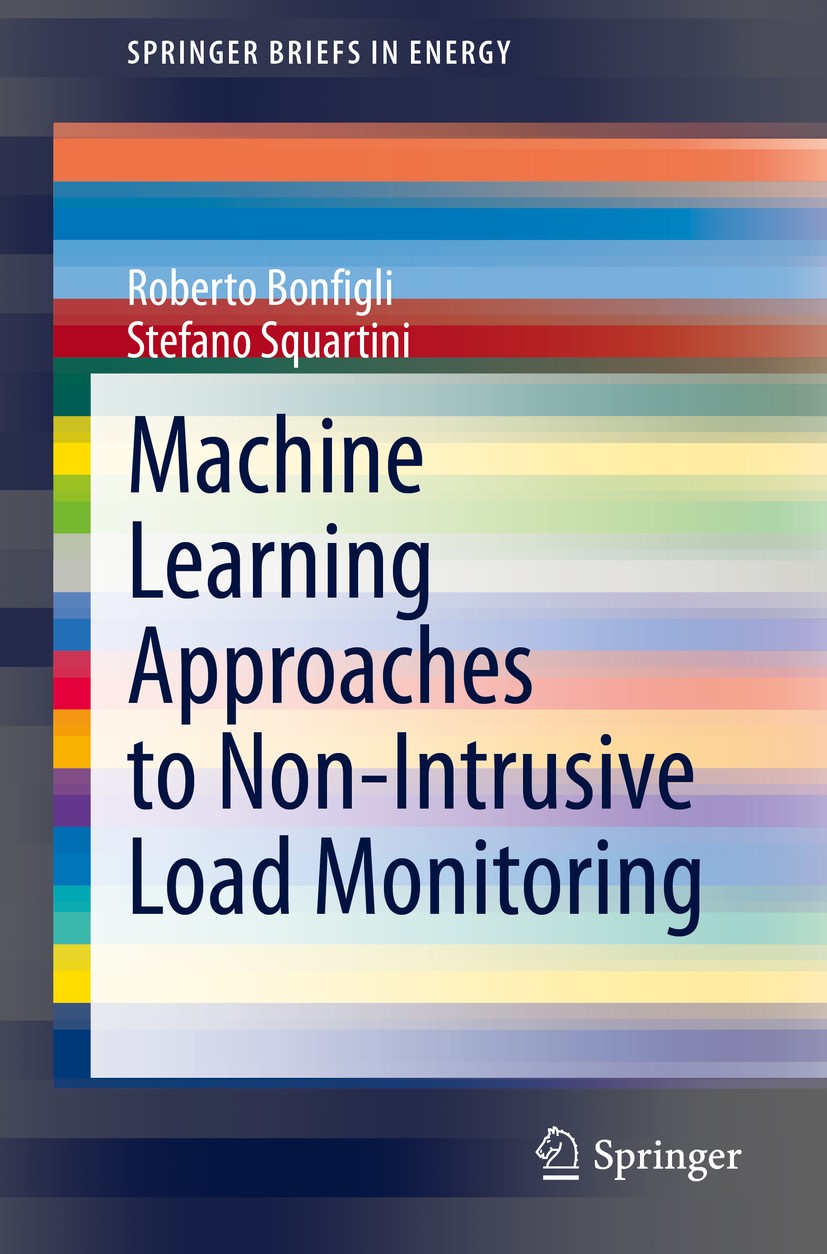 | 描述 | Research on Smart Grids has recently focused on the energy monitoring issue, with the objective of maximizing the user consumption awareness in building contexts on the one hand, and providing utilities with a detailed description of customer habits on the other. In particular, .Non-Intrusive Load Monitoring (NILM)., the subject of this book, .represents one of the hottest topics in Smart Grid applications.. NILM refers to those techniques aimed at decomposing the consumption-aggregated data acquired at a single point of measurement into the diverse consumption profiles of appliances operating in the electrical system under study. .This book provides a status report on the most promising NILM methods, with an overview of the publically available dataset on which the algorithm and experiments are based. Of the proposed methods, those based on the Hidden Markov Model (HMM) and the Deep Neural Network (DNN) are the best performing and most interesting from the future improvement point of view.. One method from each category has been selected and the performance improvements achieved are described. Comparisons are made between the two reference techniques, and pros and cons are conside | 出版日期 | Book 2020 | 关键词 | Smart Grid; Non-Intrusive Load Monitoring (NILM); Deep Neural Network (DNN); Factorial Hidden Markov Mo | 版次 | 1 | doi | https://doi.org/10.1007/978-3-030-30782-0 | isbn_softcover | 978-3-030-30781-3 | isbn_ebook | 978-3-030-30782-0Series ISSN 2191-5520 Series E-ISSN 2191-5539 | issn_series | 2191-5520 | copyright | The Author(s), under exclusive license to Springer Nature Switzerland AG 2020 |
The information of publication is updating
|
|