书目名称 | Learning in Graphical Models | 编辑 | Michael I. Jordan | 视频video | | 丛书名称 | NATO Science Series D: | 图书封面 | 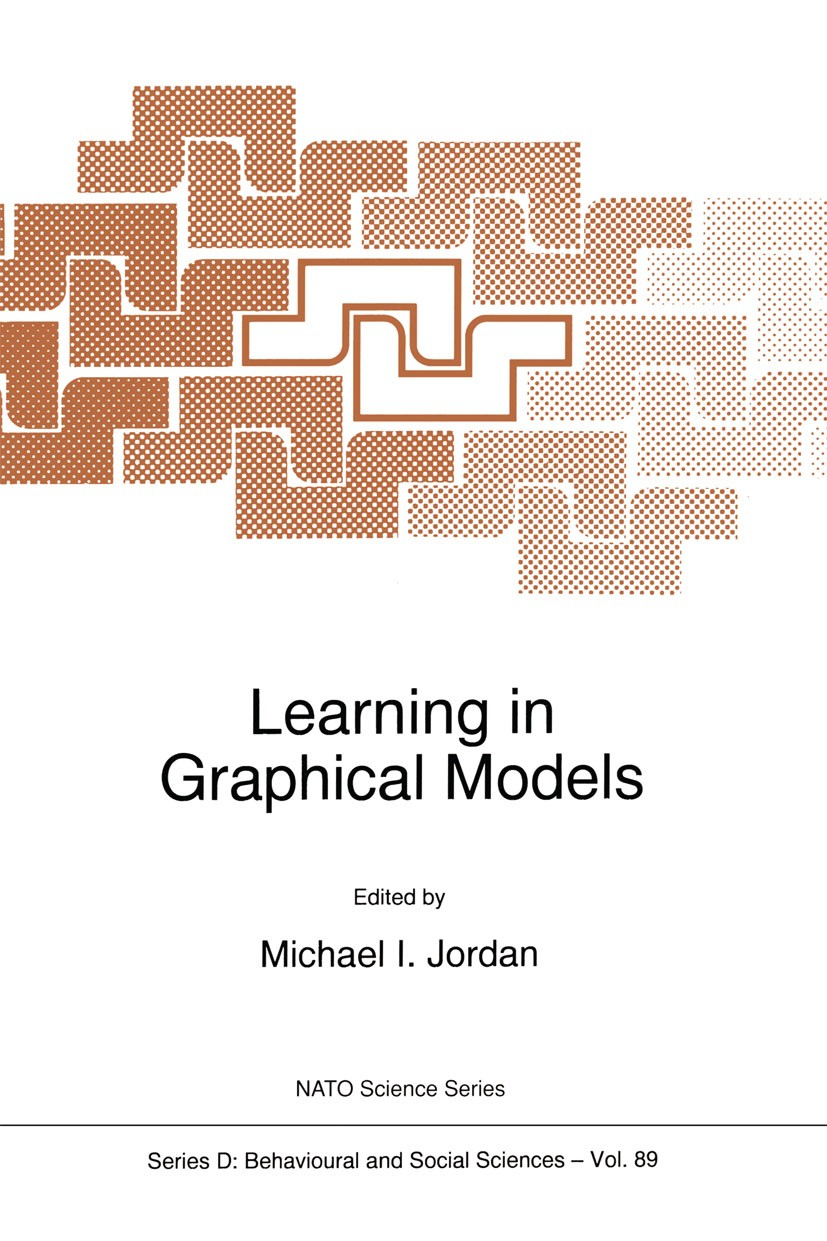 | 描述 | In the past decade, a number of different research communitieswithin the computational sciences have studied learning in networks,starting from a number of different points of view. There has beensubstantial progress in these different communities and surprisingconvergence has developed between the formalisms. The awareness ofthis convergence and the growing interest of researchers inunderstanding the essential unity of the subject underlies the currentvolume. .Two research communities which have used graphical or networkformalisms to particular advantage are the .belief network.community and the .neural network. community. Belief networksarose within computer science and statistics and were developed withan emphasis on prior knowledge and exact probabilistic calculations.Neural networks arose within electrical engineering, physics andneuroscience and have emphasised pattern recognition and systemsmodelling problems. This volume draws together researchers from thesetwo communities and presents both kinds of networks as instances of ageneral unified graphical formalism. The book focuses on probabilisticmethods for learning and inference in graphical models, algorithmanalysis and des | 出版日期 | Book 1998 | 关键词 | Bayesian network; Latent variable model; Monte Carlo method; algorithms; clustering; data analysis; electr | 版次 | 1 | doi | https://doi.org/10.1007/978-94-011-5014-9 | isbn_softcover | 978-94-010-6104-9 | isbn_ebook | 978-94-011-5014-9Series ISSN 0258-123X | issn_series | 0258-123X | copyright | Springer Science+Business Media Dordrecht 1998 |
The information of publication is updating
|
|