书目名称 | Knowledge Science, Engineering and Management | 副标题 | 16th International C | 编辑 | Zhi Jin,Yuncheng Jiang,Wenjun Ma | 视频video | | 丛书名称 | Lecture Notes in Computer Science | 图书封面 | 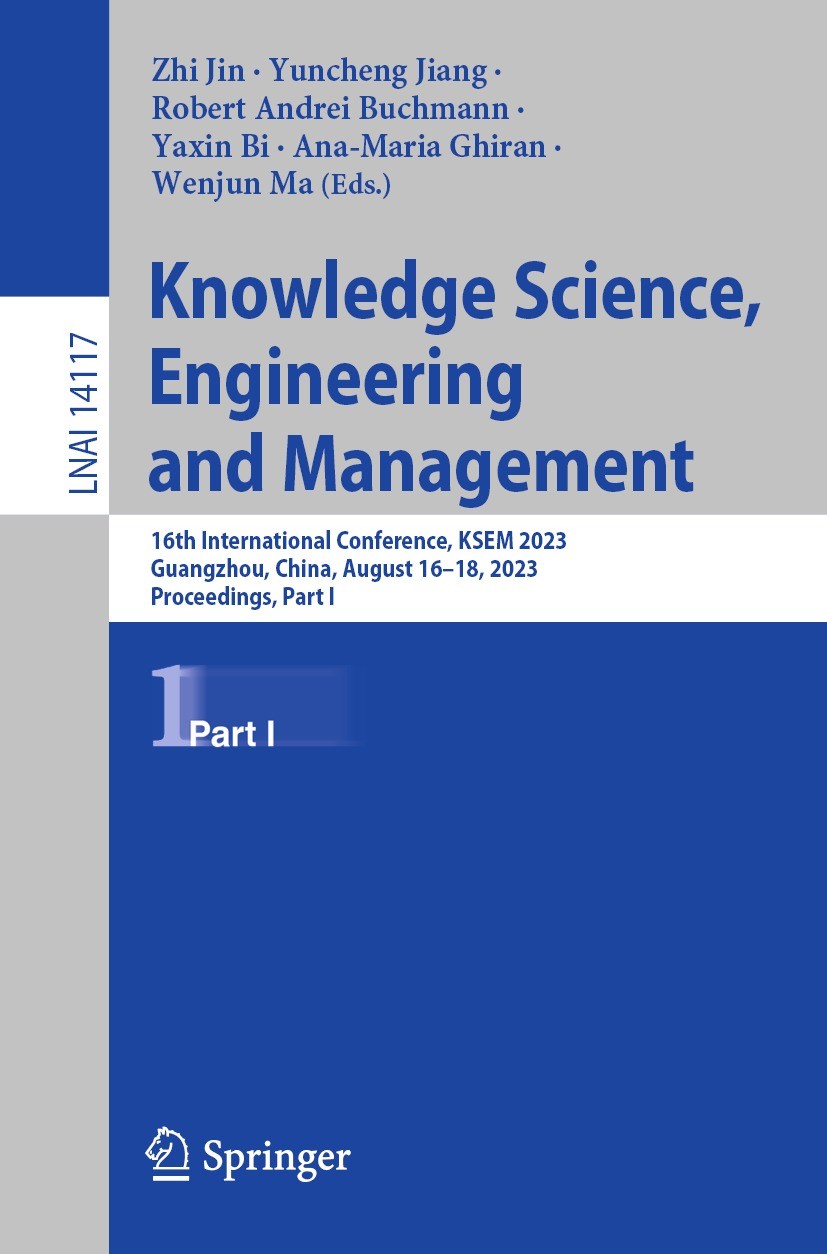 | 描述 | This volume set constitutes the refereed proceedings of the 16th International Conference on Knowledge Science, Engineering and Management, KSEM 2023, which was held in Guangzhou, China, during August 16–18, 2023. .The 114 full papers and 30 short papers included in this book were carefully reviewed and selected from 395 submissions. They were organized in topical sections as follows: knowledge science with learning and AI; knowledge engineering research and applications; knowledge management systems; and emerging technologies for knowledge science, engineering and management. . | 出版日期 | Conference proceedings 2023 | 关键词 | artificial intelligence; computational linguistics; computer networks; data mining; databases; directed g | 版次 | 1 | doi | https://doi.org/10.1007/978-3-031-40283-8 | isbn_softcover | 978-3-031-40282-1 | isbn_ebook | 978-3-031-40283-8Series ISSN 0302-9743 Series E-ISSN 1611-3349 | issn_series | 0302-9743 | copyright | The Editor(s) (if applicable) and The Author(s), under exclusive license to Springer Nature Switzerl |
The information of publication is updating
书目名称Knowledge Science, Engineering and Management影响因子(影响力) 
书目名称Knowledge Science, Engineering and Management影响因子(影响力)学科排名 
书目名称Knowledge Science, Engineering and Management网络公开度 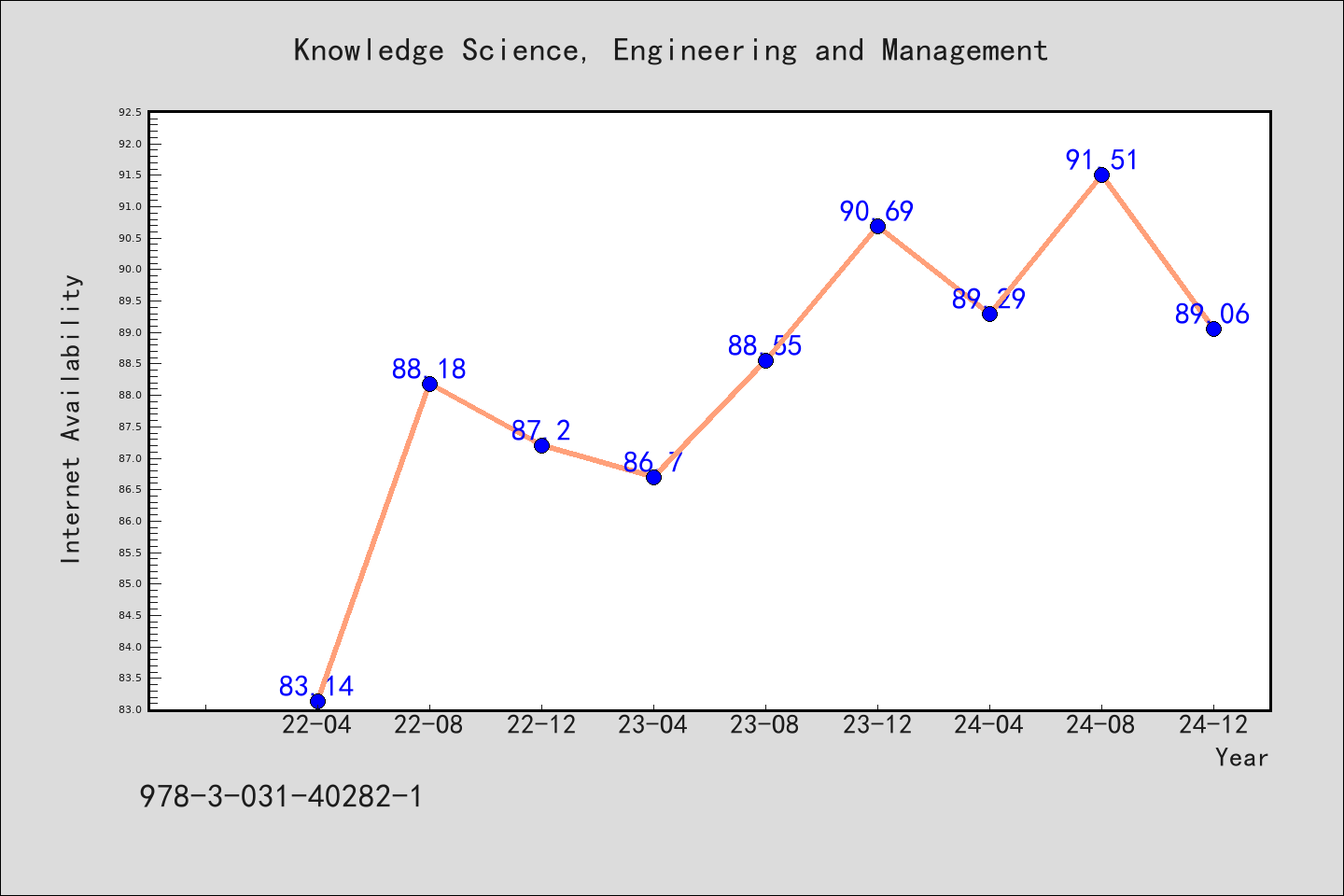
书目名称Knowledge Science, Engineering and Management网络公开度学科排名 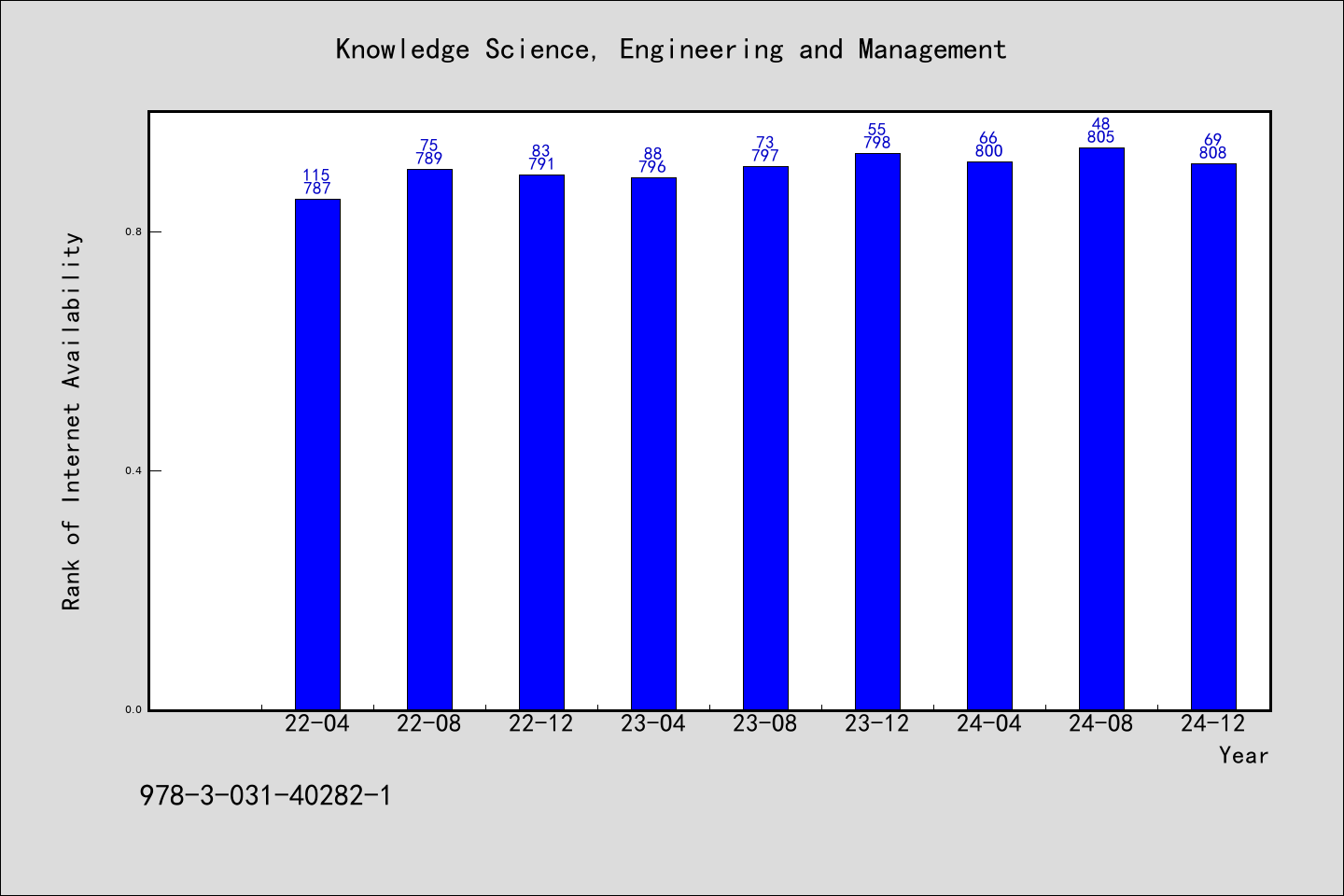
书目名称Knowledge Science, Engineering and Management被引频次 
书目名称Knowledge Science, Engineering and Management被引频次学科排名 
书目名称Knowledge Science, Engineering and Management年度引用 
书目名称Knowledge Science, Engineering and Management年度引用学科排名 
书目名称Knowledge Science, Engineering and Management读者反馈 
书目名称Knowledge Science, Engineering and Management读者反馈学科排名 
|
|
|