书目名称 | Joint Training for Neural Machine Translation |
编辑 | Yong Cheng |
视频video | |
概述 | Nominated by Tsinghua University as an outstanding Ph.D. thesis.Reports on current challenges and important advances in neural machine translation.Addresses training jointly bidirectional neural machi |
丛书名称 | Springer Theses |
图书封面 | 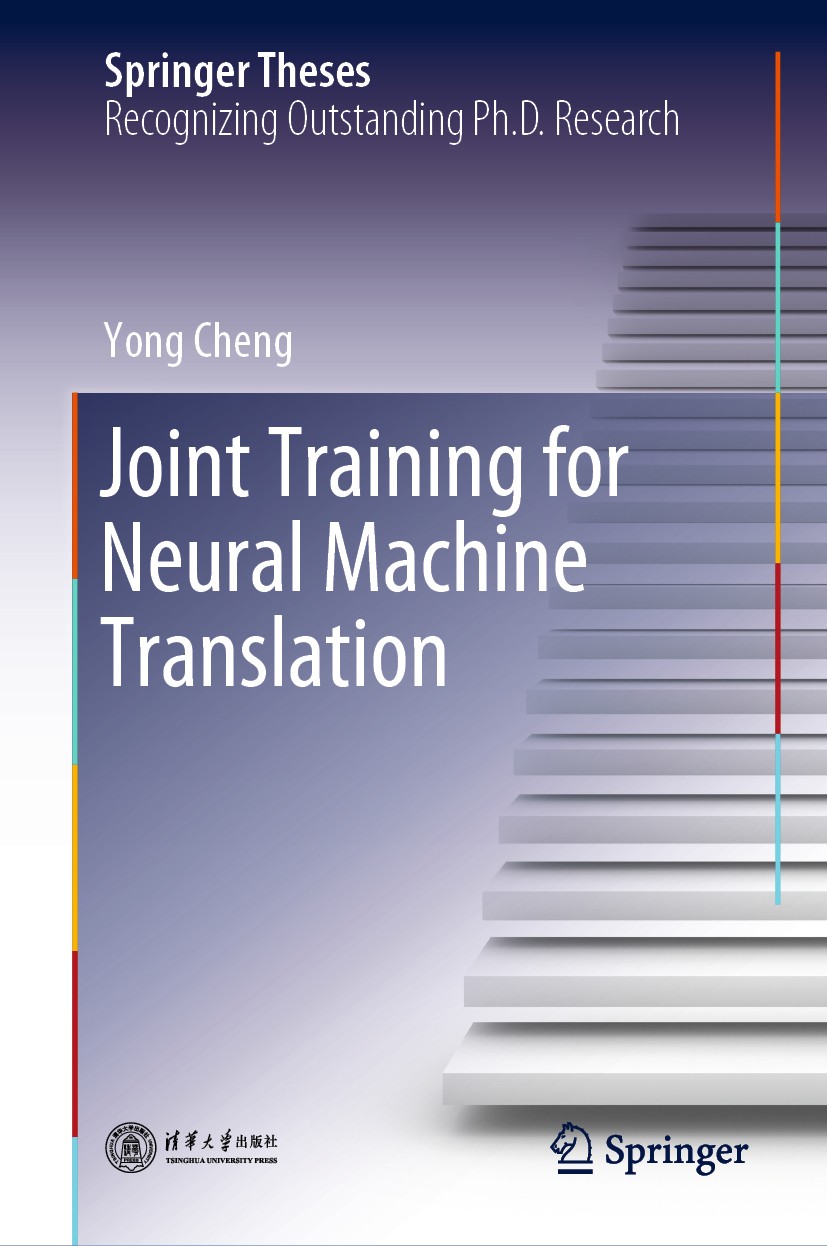 |
描述 | .This book presents four approaches to jointly training bidirectional neural machine translation (NMT) models. First, in order to improve the accuracy of the attention mechanism, it proposes an agreement-based joint training approach to help the two complementary models agree on word alignment matrices for the same training data. Second, it presents a semi-supervised approach that uses an autoencoder to reconstruct monolingual corpora, so as to incorporate these corpora into neural machine translation. It then introduces a joint training algorithm for pivot-based neural machine translation, which can be used to mitigate the data scarcity problem. Lastly it describes an end-to-end bidirectional NMT model to connect the source-to-target and target-to-source translation models, allowing the interaction of parameters between these two directional models.. |
出版日期 | Book 2019 |
关键词 | Machine Translation; Neural Machine Translation; Joint Training; Joint Modeling; Bidirectional Model |
版次 | 1 |
doi | https://doi.org/10.1007/978-981-32-9748-7 |
isbn_ebook | 978-981-32-9748-7Series ISSN 2190-5053 Series E-ISSN 2190-5061 |
issn_series | 2190-5053 |
copyright | Springer Nature Singapore Pte Ltd. 2019 |