书目名称 | Introduction to Graph Neural Networks | 编辑 | Zhiyuan Liu,Jie Zhou | 视频video | | 丛书名称 | Synthesis Lectures on Artificial Intelligence and Machine Learning | 图书封面 | 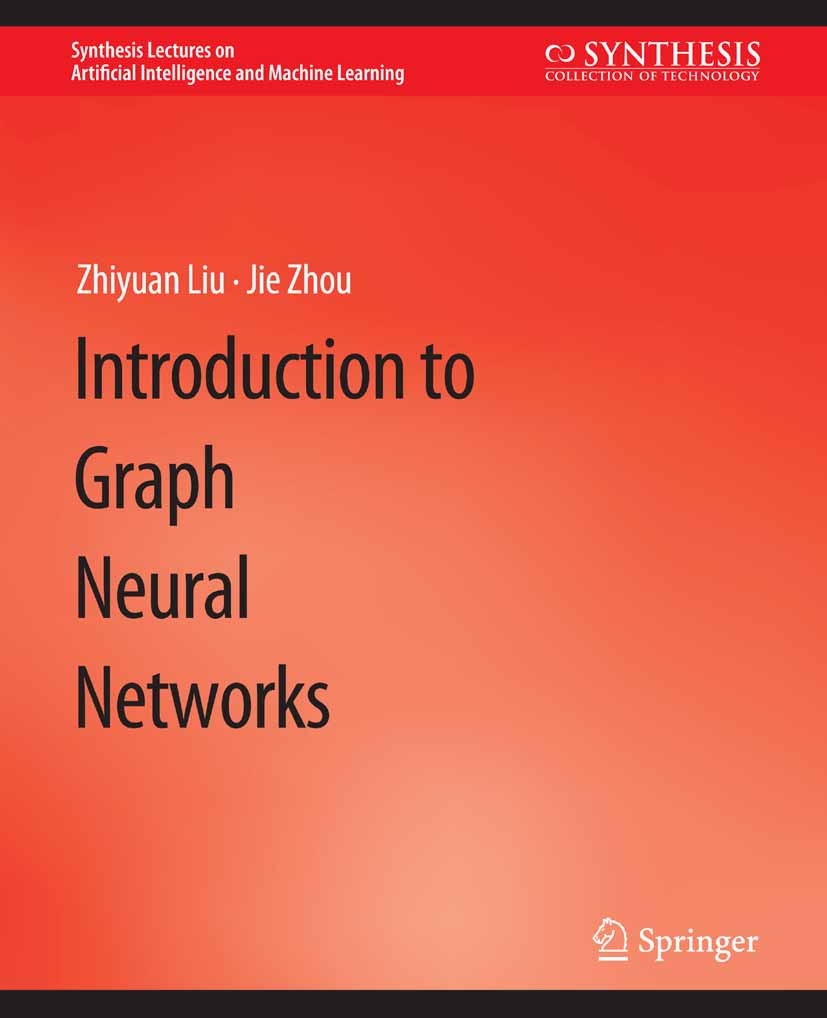 | 描述 | .Graphs are useful data structures in complex real-life applications such as modeling physical systems, learning molecular fingerprints, controlling traffic networks, and recommending friends in social networks. However, these tasks require dealing with non-Euclidean graph data that contains rich relational information between elements and cannot be well handled by traditional deep learning models (e.g., convolutional neural networks (CNNs) or recurrent neural networks (RNNs)). Nodes in graphs usually contain useful feature information that cannot be well addressed in most unsupervised representation learning methods (e.g., network embedding methods). Graph neural networks (GNNs) are proposed to combine the feature information and the graph structure to learn better representations on graphs via feature propagation and aggregation. Due to its convincing performance and high interpretability, GNN has recently become a widely applied graph analysis tool..This book provides a comprehensive introduction to the basic concepts, models, and applications of graph neural networks. It starts with the introduction of the vanilla GNN model. Then several variants of the vanilla model are introd | 出版日期 | Book 2020 | 版次 | 1 | doi | https://doi.org/10.1007/978-3-031-01587-8 | isbn_softcover | 978-3-031-00459-9 | isbn_ebook | 978-3-031-01587-8Series ISSN 1939-4608 Series E-ISSN 1939-4616 | issn_series | 1939-4608 | copyright | Springer Nature Switzerland AG 2020 |
The information of publication is updating
|
|