书目名称 | Introduction to Deep Learning | 副标题 | From Logical Calculu | 编辑 | Sandro Skansi | 视频video | | 概述 | Offers a welcome clarity of expression, maintaining mathematical rigor yet presenting the ideas in an intuitive and colourful manner.Includes references to open problems studied in other disciplines, | 丛书名称 | Undergraduate Topics in Computer Science | 图书封面 | 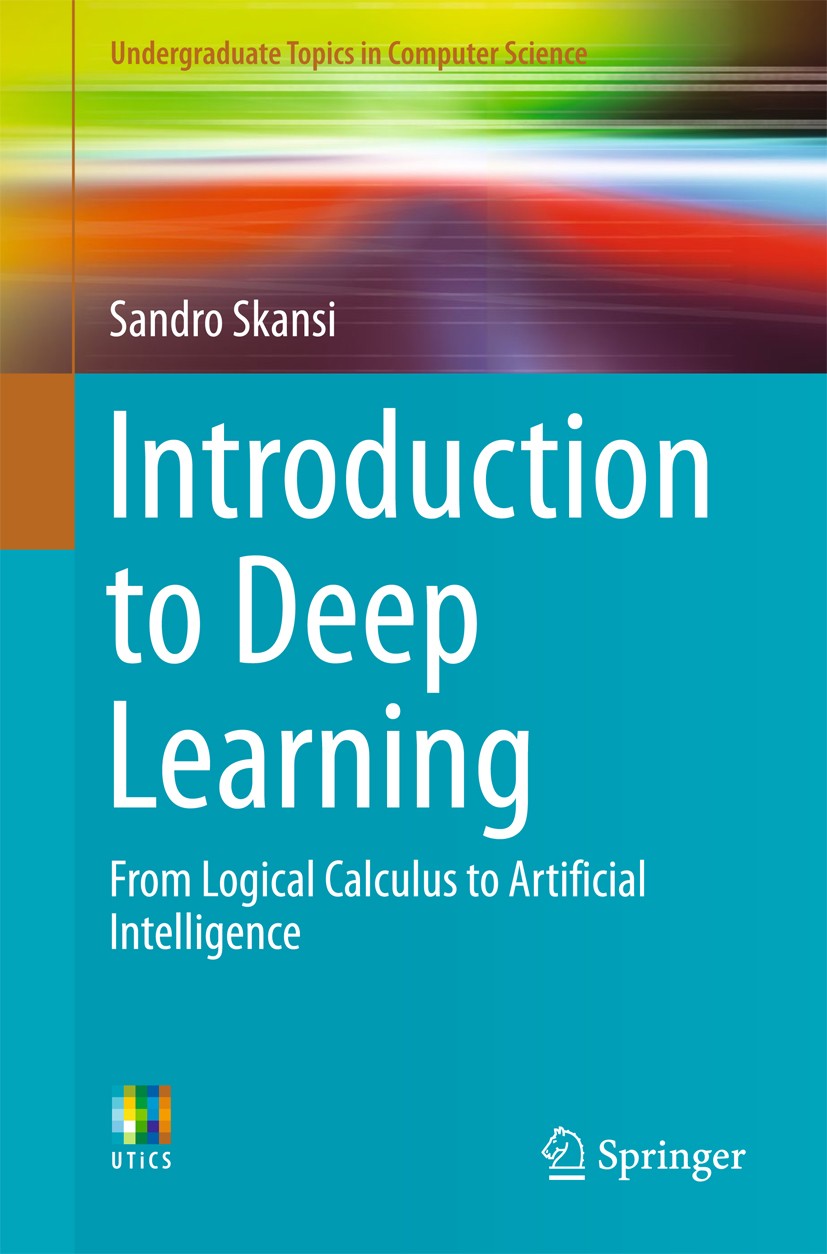 | 描述 | .This textbook presents a concise, accessible and engaging first introduction to deep learning, offering a wide range of connectionist models which represent the current state-of-the-art. The text explores the most popular algorithms and architectures in a simple and intuitive style, explaining the mathematical derivations in a step-by-step manner. The content coverage includes convolutional networks, LSTMs, Word2vec, RBMs, DBNs, neural Turing machines, memory networks and autoencoders. Numerous examples in working Python code are provided throughout the book, and the code is also supplied separately at an accompanying website..Topics and features: introduces the fundamentals of machine learning, and the mathematical and computational prerequisites for deep learning; discusses feed-forward neural networks, and explores the modifications to these which can be applied to any neural network; examines convolutional neural networks, and the recurrent connections to a feed-forward neural network; describes the notion of distributed representations, the concept of the autoencoder, and the ideas behind language processing with deep learning; presents a brief history of artificial intellige | 出版日期 | Textbook 2018 | 关键词 | Deep learning; Neural networks; Pattern recognition; Natural language processing; Autoencoders | 版次 | 1 | doi | https://doi.org/10.1007/978-3-319-73004-2 | isbn_softcover | 978-3-319-73003-5 | isbn_ebook | 978-3-319-73004-2Series ISSN 1863-7310 Series E-ISSN 2197-1781 | issn_series | 1863-7310 | copyright | Springer International Publishing AG, part of Springer Nature 2018 |
The information of publication is updating
|
|