书目名称 | Information and Complexity in Statistical Modeling | 编辑 | Jorma Rissanen | 视频video | | 概述 | The author is a distinguished scientist in information theory and statistical modeling | 丛书名称 | Information Science and Statistics | 图书封面 | 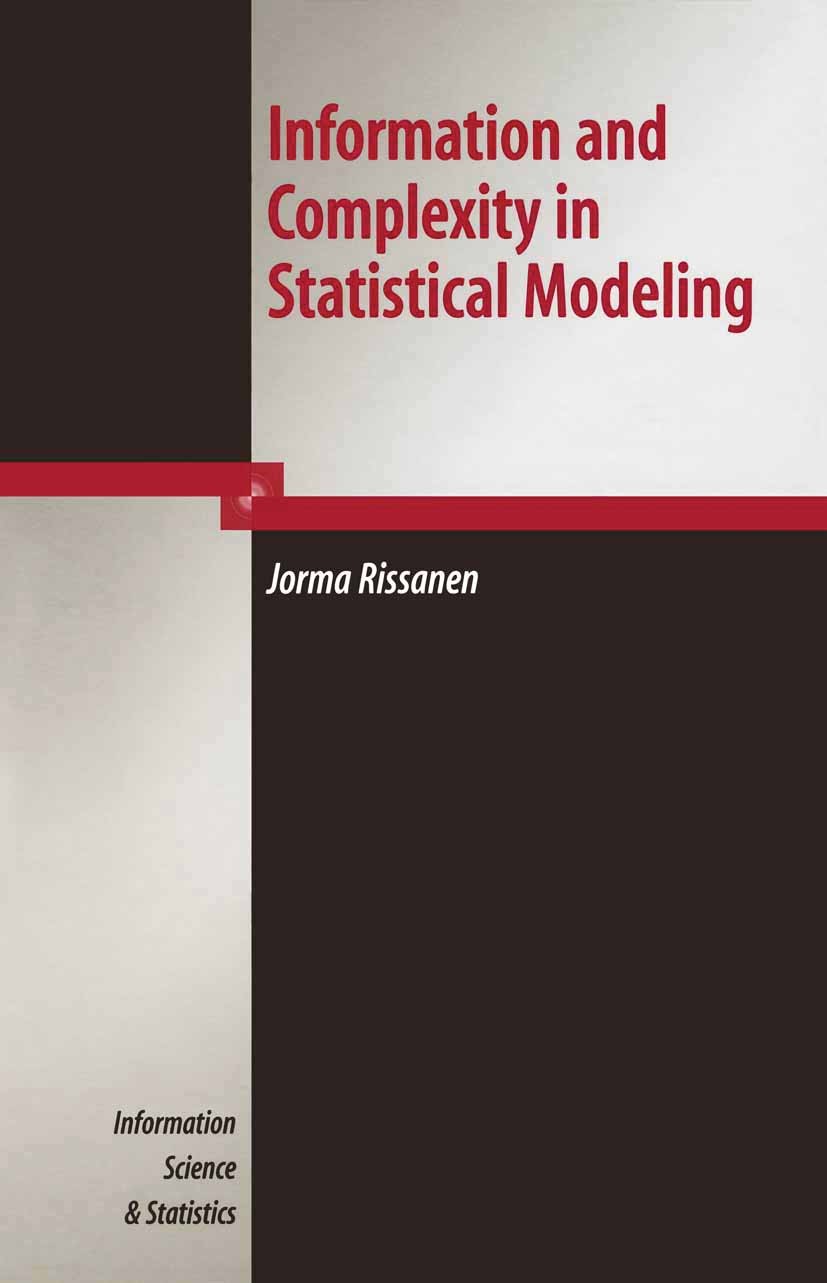 | 描述 | .No statistical model is "true" or "false," "right" or "wrong"; the models just have varying performance, which can be assessed. The main theme in this book is to teach modeling based on the principle that the objective is to extract the information from data that can be learned with suggested classes of probability models. The intuitive and fundamental concepts of complexity, learnable information, and noise are formalized, which provides a firm information theoretic foundation for statistical modeling. Inspired by Kolmogorov‘s structure function in the algorithmic theory of complexity, this is accomplished by finding the shortest code length, called the stochastic complexity, with which the data can be encoded when advantage is taken of the models in a suggested class, which amounts to the MDL (Minimum Description Length) principle. The complexity, in turn, breaks up into the shortest code length for the optimal model in a set of models that can be optimally distinguished from the given data and the rest, which defines "noise" as the incompressible part in the data without useful information....Such a view of the modeling problem permits a unified treatment of any type of paramet | 出版日期 | Book 2007 | 关键词 | Excel; Information; Minimum Description Length; Shannon; Signal; algorithm; algorithms; bioinformatics; calc | 版次 | 1 | doi | https://doi.org/10.1007/978-0-387-68812-1 | isbn_softcover | 978-1-4419-2267-0 | isbn_ebook | 978-0-387-68812-1Series ISSN 1613-9011 Series E-ISSN 2197-4128 | issn_series | 1613-9011 | copyright | Springer-Verlag New York 2007 |
The information of publication is updating
|
|