书目名称 | Information Theoretic Learning | 副标题 | Renyi‘s Entropy and | 编辑 | Jose C. Principe | 视频video | | 概述 | Includes supplementary material: | 丛书名称 | Information Science and Statistics | 图书封面 | 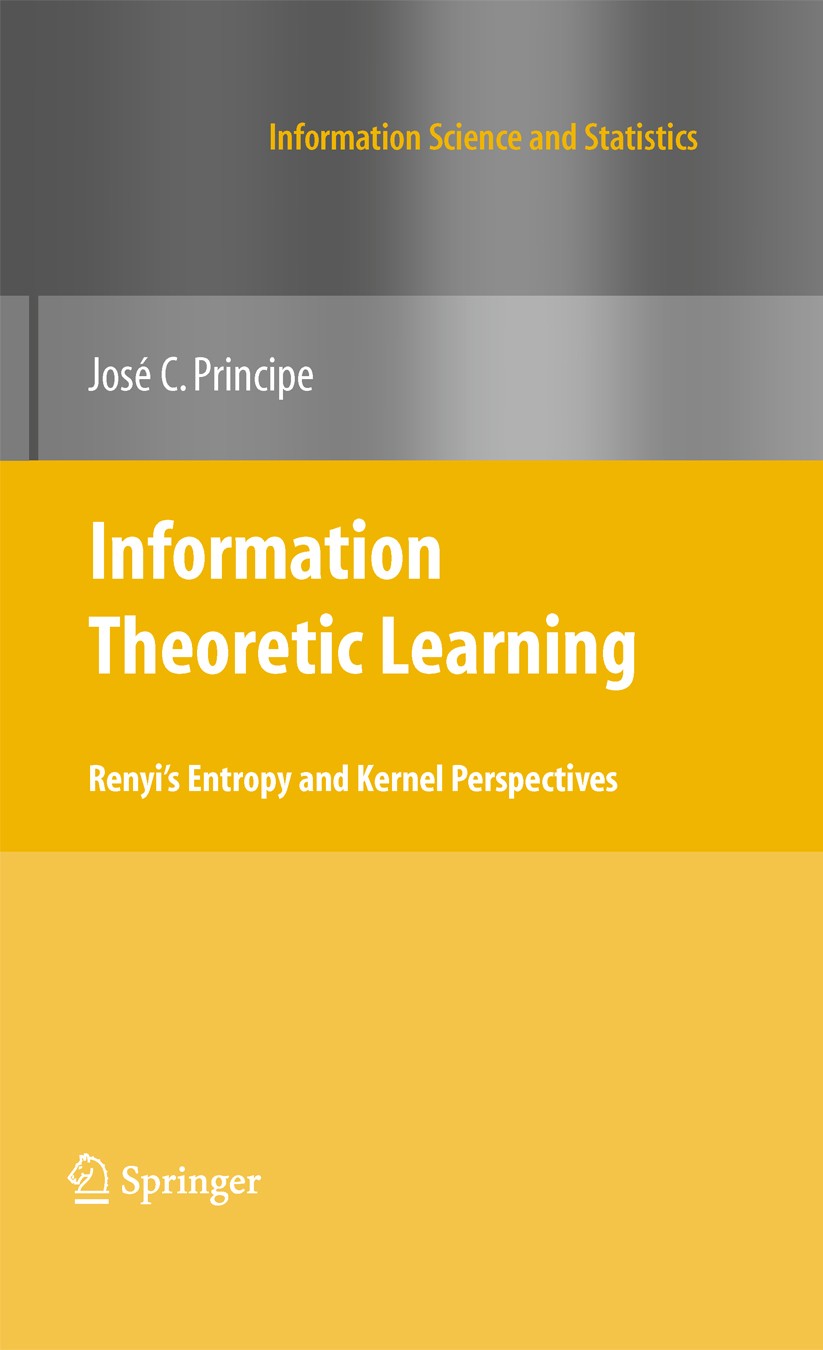 | 描述 | .This book presents the first cohesive treatment of Information Theoretic Learning (ITL) algorithms to adapt linear or nonlinear learning machines both in supervised or unsupervised paradigms. ITL is a framework where the conventional concepts of second order statistics (covariance, L2 distances, correlation functions) are substituted by scalars and functions with information theoretic underpinnings, respectively entropy, mutual information and correntropy. .ITL quantifies the stochastic structure of the data beyond second order statistics for improved performance without using full-blown Bayesian approaches that require a much larger computational cost. This is possible because of a non-parametric estimator of Renyi’s quadratic entropy that is only a function of pairwise differences between samples. The book compares the performance of ITL algorithms with the second order counterparts in many engineering and machine learning applications..Students, practitioners and researchers interested in statistical signal processing, computational intelligence, and machine learning will find in this book the theory to understand the basics, the algorithms to implement applications, and exciti | 出版日期 | Book 2010 | 关键词 | Correntropy; Information theoretic learning; Nongaussian signal processing; RKHS and information theory | 版次 | 1 | doi | https://doi.org/10.1007/978-1-4419-1570-2 | isbn_softcover | 978-1-4614-2585-4 | isbn_ebook | 978-1-4419-1570-2Series ISSN 1613-9011 Series E-ISSN 2197-4128 | issn_series | 1613-9011 | copyright | Springer-Verlag New York 2010 |
The information of publication is updating
|
|