书目名称 | Genetic Programming | 副标题 | 27th European Confer | 编辑 | Mario Giacobini,Bing Xue,Luca Manzoni | 视频video | | 丛书名称 | Lecture Notes in Computer Science | 图书封面 | 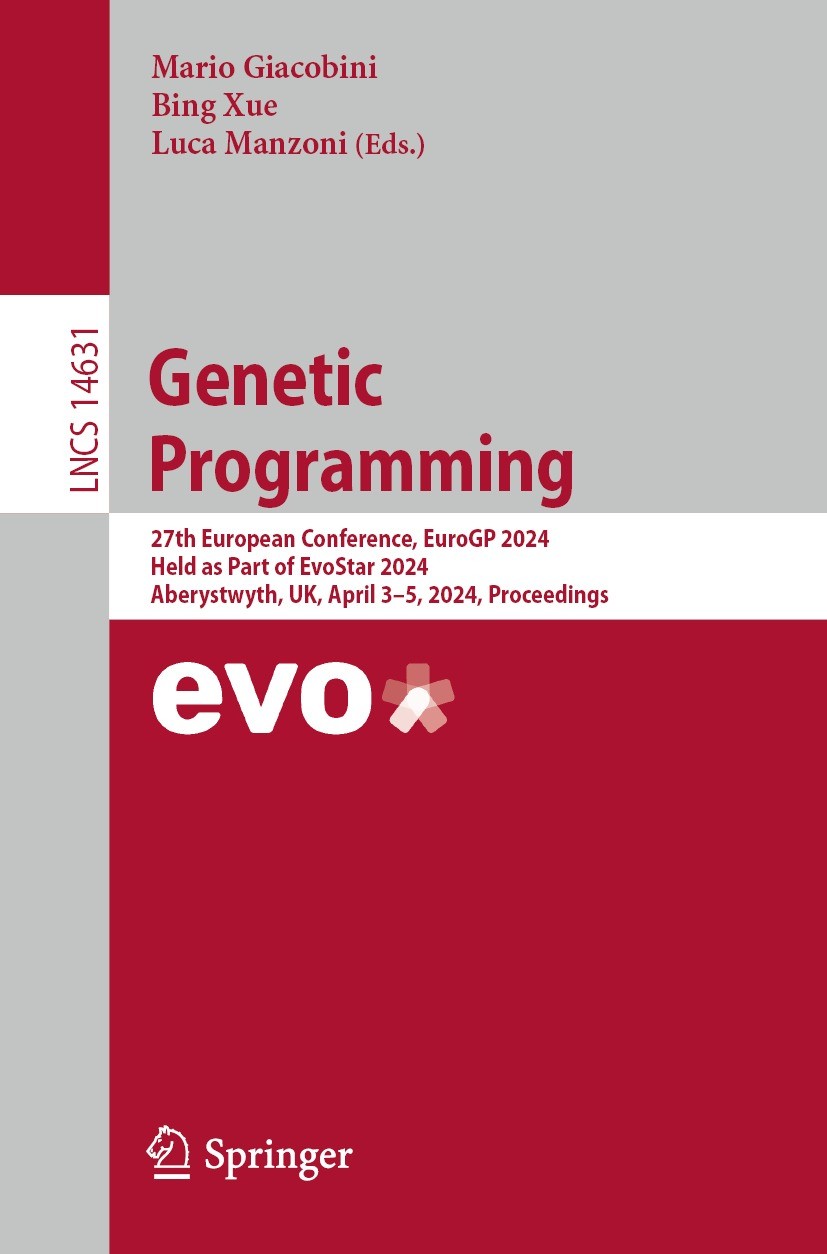 | 描述 | .This book constitutes the refereed proceedings of the 27th European Conference on Genetic Programming, EuroGP 2024, held in Aberystwyth, UK, April 3–5, 2024 and co-located with the EvoStar events, EvoCOP, EvoMUSART, and EvoApplications..The 13 papers (9 selected for long presentation and 4 for short presentation) collected in this book were carefully reviewed and selected from 24 submissions. The wide range of topics in this volume reflects the current state of research in the field. The collection of papers cover topics including developing new variants of GP algorithms, as well as exploring GP applications to the optimization of machine learning methods and the evolution of control policies. | 出版日期 | Conference proceedings 2024 | 关键词 | artificial intelligence; computer programming; computer systems; correlation analysis; distributed syste | 版次 | 1 | doi | https://doi.org/10.1007/978-3-031-56957-9 | isbn_softcover | 978-3-031-56956-2 | isbn_ebook | 978-3-031-56957-9Series ISSN 0302-9743 Series E-ISSN 1611-3349 | issn_series | 0302-9743 | copyright | The Editor(s) (if applicable) and The Author(s), under exclusive license to Springer Nature Switzerl |
The information of publication is updating
|
|