书目名称 | Genetic Programming | 副标题 | 20th European Confer | 编辑 | James McDermott,Mauro Castelli,Pablo García-Sánche | 视频video | | 概述 | Includes supplementary material: | 丛书名称 | Lecture Notes in Computer Science | 图书封面 | 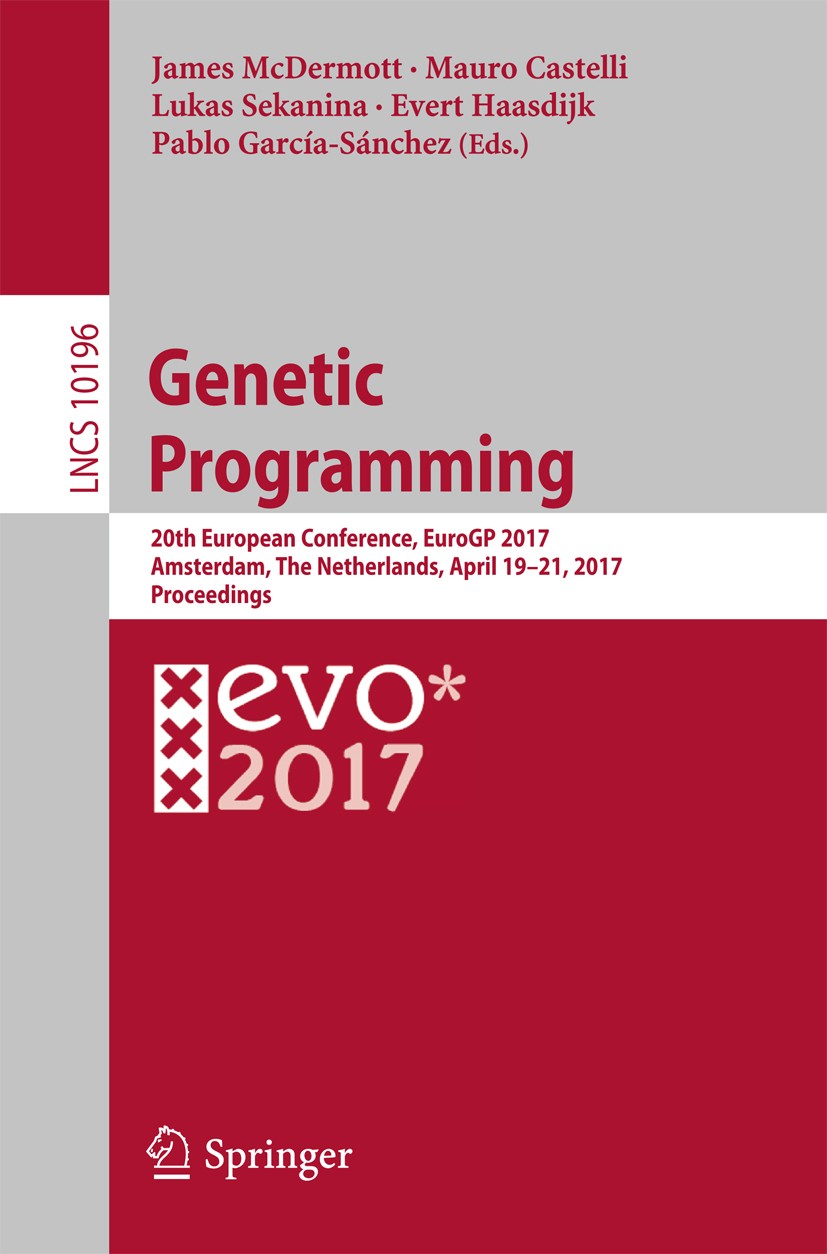 | 描述 | This book constitutes the refereed proceedings of the 20th European Conference on Genetic Programming, EuroGP 2017, held in Amsterdam, The Netherlands, in April 2017, co-located with the Evo* 2017 events, EvoCOP, EvoMUSART, and EvoApplications..The 14 revised full papers presented together with 8 poster papers were carefully reviewed and selected from 32 submissions. The wide range of topics in this volume reflects the current state of research in the field. Thus, we see topics and applications including program synthesis, genetic improvement, grammatical representations, self-adaptation, multi-objective optimisation, program semantics, search landscapes, mathematical programming, games, operations research, networks, evolvable hardware, and program synthesis benchmarks.. | 出版日期 | Conference proceedings 2017 | 关键词 | artificial intelligence; evolutionary computation; machine learning; mathematical programming; program s | 版次 | 1 | doi | https://doi.org/10.1007/978-3-319-55696-3 | isbn_softcover | 978-3-319-55695-6 | isbn_ebook | 978-3-319-55696-3Series ISSN 0302-9743 Series E-ISSN 1611-3349 | issn_series | 0302-9743 | copyright | Springer International Publishing AG 2017 |
The information of publication is updating
|
|