书目名称 | Generative Adversarial Networks for Image Generation | 编辑 | Xudong Mao,Qing Li | 视频video | | 概述 | Offers an overview of the theoretical concepts and the current challenges of generative adversarial networks.Proposes advanced GAN image generation approaches with higher image quality and better trai | 图书封面 | 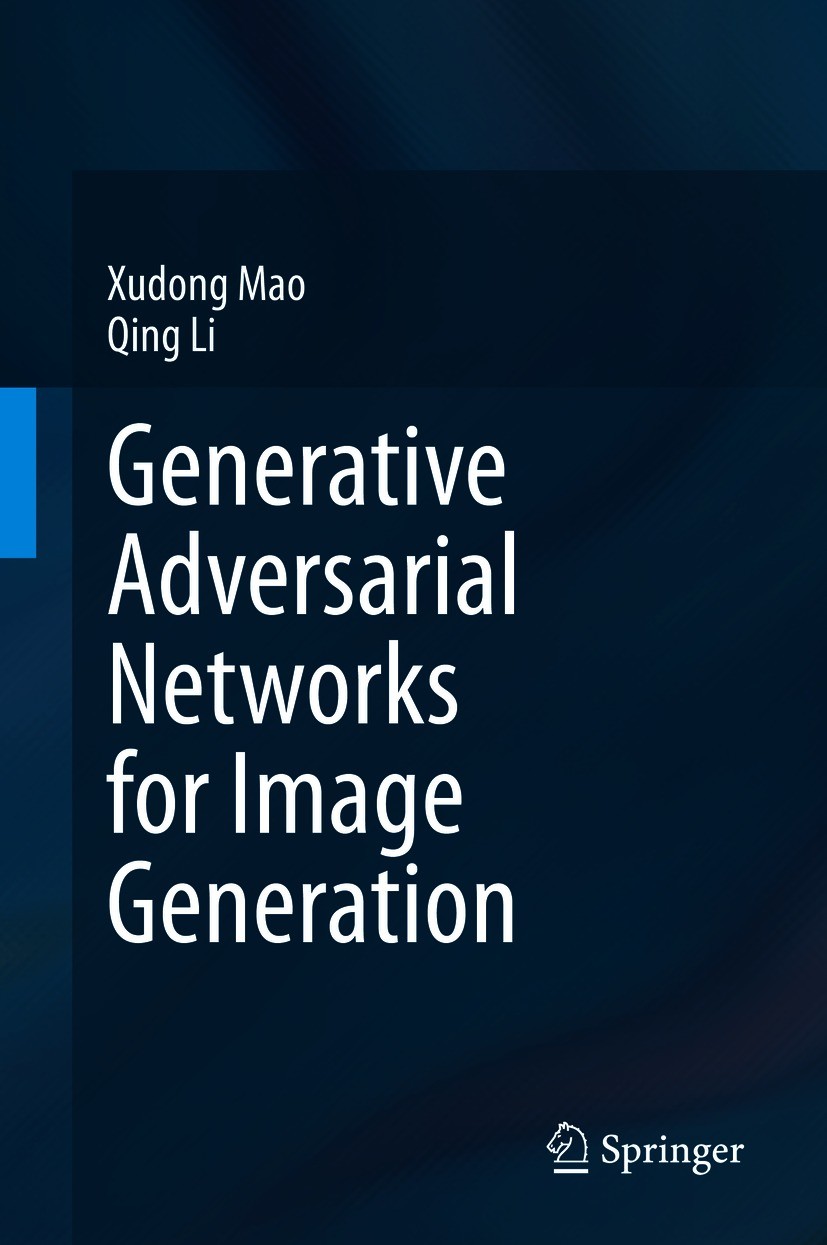 | 描述 | .Generative adversarial networks (GANs) were introduced by Ian Goodfellow and his co-authors including Yoshua Bengio in 2014, and were to referred by Yann Lecun (Facebook’s AI research director) as “the most interesting idea in the last 10 years in ML.” GANs’ potential is huge, because they can learn to mimic any distribution of data, which means they can be taught to create worlds similar to our own in any domain: images, music, speech, prose. They are robot artists in a sense, and their output is remarkable – poignant even. In 2018, Christie’s sold a portrait that had been generated by a GAN for $432,000...Although image generation has been challenging, GAN image generation has proved to be very successful and impressive. However, there are two remaining challenges for GAN image generation: the quality of the generated image and the training stability. This book first provides an overview of GANs, and then discusses the task of image generation and the detailsof GAN image generation. It also investigates a number of approaches to address the two remaining challenges for GAN image generation. Additionally, it explores three promising applications of GANs, including image-to-image | 出版日期 | Book 2021 | 关键词 | Generative Adversarial Networks; GANs; Adversarial Networks; Image Generation; Generative Models; Image t | 版次 | 1 | doi | https://doi.org/10.1007/978-981-33-6048-8 | isbn_softcover | 978-981-33-6050-1 | isbn_ebook | 978-981-33-6048-8 | copyright | Springer Nature Singapore Pte Ltd. 2021 |
The information of publication is updating
|
|